Robust Communication and Computation Using Deep Learning Via Joint Uncertainty Injection
2024 19th International Symposium on Wireless Communication Systems (ISWCS)(2024)
摘要
The convergence of communication and computation, along with the integrationof machine learning and artificial intelligence, stand as key empoweringpillars for the sixth-generation of communication systems (6G). This paperconsiders a network of one base station serving a number of devicessimultaneously using spatial multiplexing. The paper then presents aninnovative deep learning-based approach to simultaneously manage the transmitand computing powers, alongside computation allocation, amidst uncertainties inboth channel and computing states information. More specifically, the paperaims at proposing a robust solution that minimizes the worst-case delay acrossthe served devices subject to computation and power constraints. The paper usesa deep neural network (DNN)-based solution that maps estimated channels andcomputation requirements to optimized resource allocations. During training,uncertainty samples are injected after the DNN output to jointly account forboth communication and computation estimation errors. The DNN is then trainedvia backpropagation using the robust utility, thus implicitly learning theuncertainty distributions. Our results validate the enhanced robust delayperformance of the joint uncertainty injection versus the classical DNNapproach, especially in high channel and computational uncertainty regimes.
更多查看译文
关键词
Deep Learning,Computational Robustness,Robust Communication,Joint Uncertainty,Resource Allocation,Deep Neural Network,Power Calculation,Base Station,Computational Requirements,Power Constraint,Optimal Resource Allocation,Spatial Multiplexing,Integration Of Machine Learning,Delay Performance,Output Of The Deep Neural Network,Resource Management,Computational Resources,Additive Noise,Input Layer,Communication Delay,Delay Estimation,Digital Twin,Cycle Calculation,Deep Neural Network Parameters,Beamforming Vector,Antennal,Robust Object,6G Networks,Softmax Function
AI 理解论文
溯源树
样例
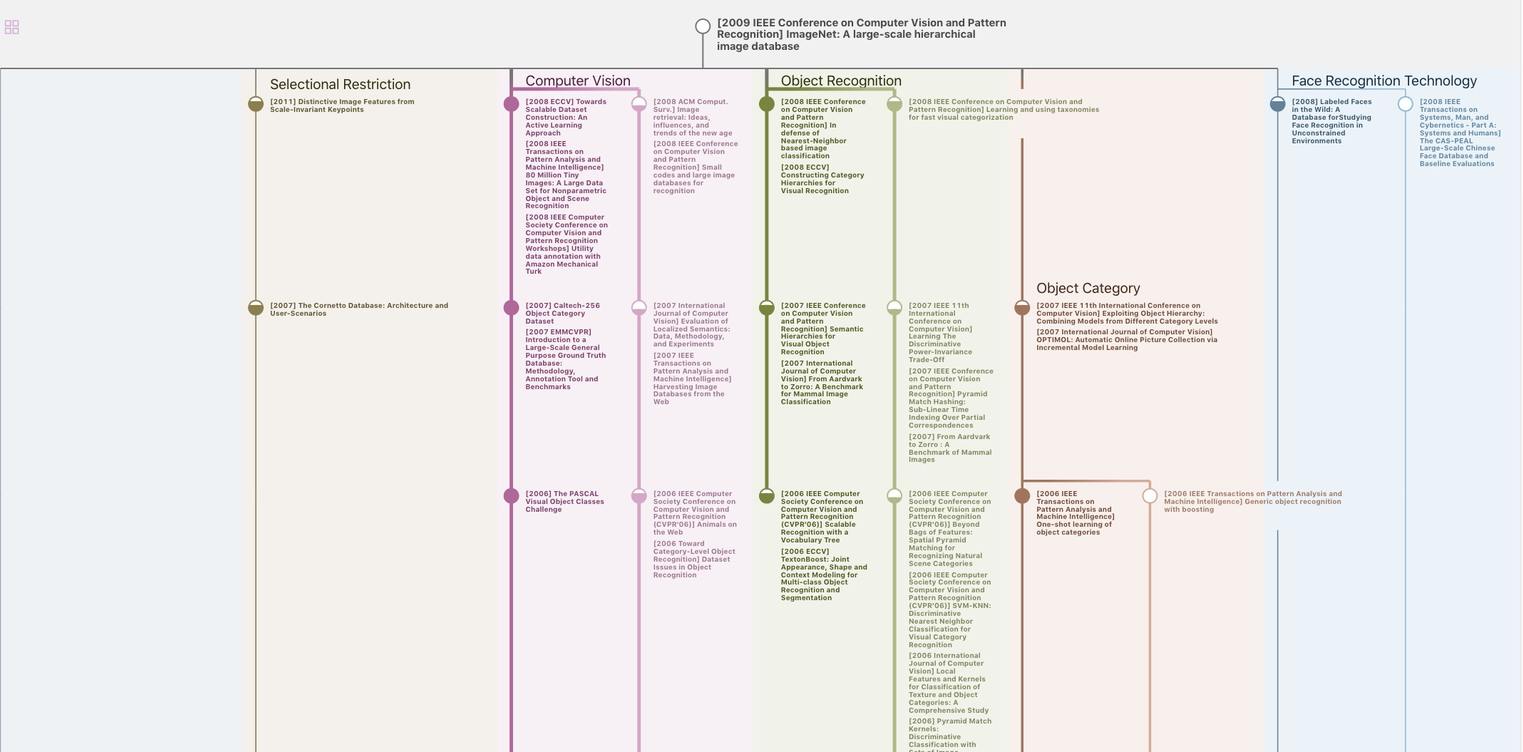
生成溯源树,研究论文发展脉络
Chat Paper
正在生成论文摘要