Machine learning-based prediction of compressive strength in circular FRP-confined concrete columns
Frontiers in Materials(2024)
摘要
This research aims to evaluate the compressive strength of FRP-confined columns using machine learning models. By systematically organizing codes and models proposed by various researchers, significant indicators influencing compressive strength have been identified. A comprehensive database comprising 366 samples, including both CFRP and GFRP, has been assembled. Based on this database, a machine learning model was developed to accurately predict compressive strength. A thorough evaluation was conducted, comparing models proposed by codes and researchers. Additionally, a detailed parameter analysis was performed using the XGBoost model. The findings highlight the importance of both code-based and researcher-proposed models in enhancing our understanding of compressive strength. However, certain models show tendencies towards conservative or overestimated predictions, indicating the need for further accuracy enhancement. Among the models considered, the XGBoost model demonstrated the highest goodness of fit (0.97) and the lowest coefficient of variation (8%), making it a suitable choice for investigating compressive strength. Notable parameters significantly influencing compressive strength include FRP thickness, elastic modulus, and concrete strength.
更多查看译文
关键词
FRP-confined columns,compressive strength,machine learning,XGBoost,prediction model
AI 理解论文
溯源树
样例
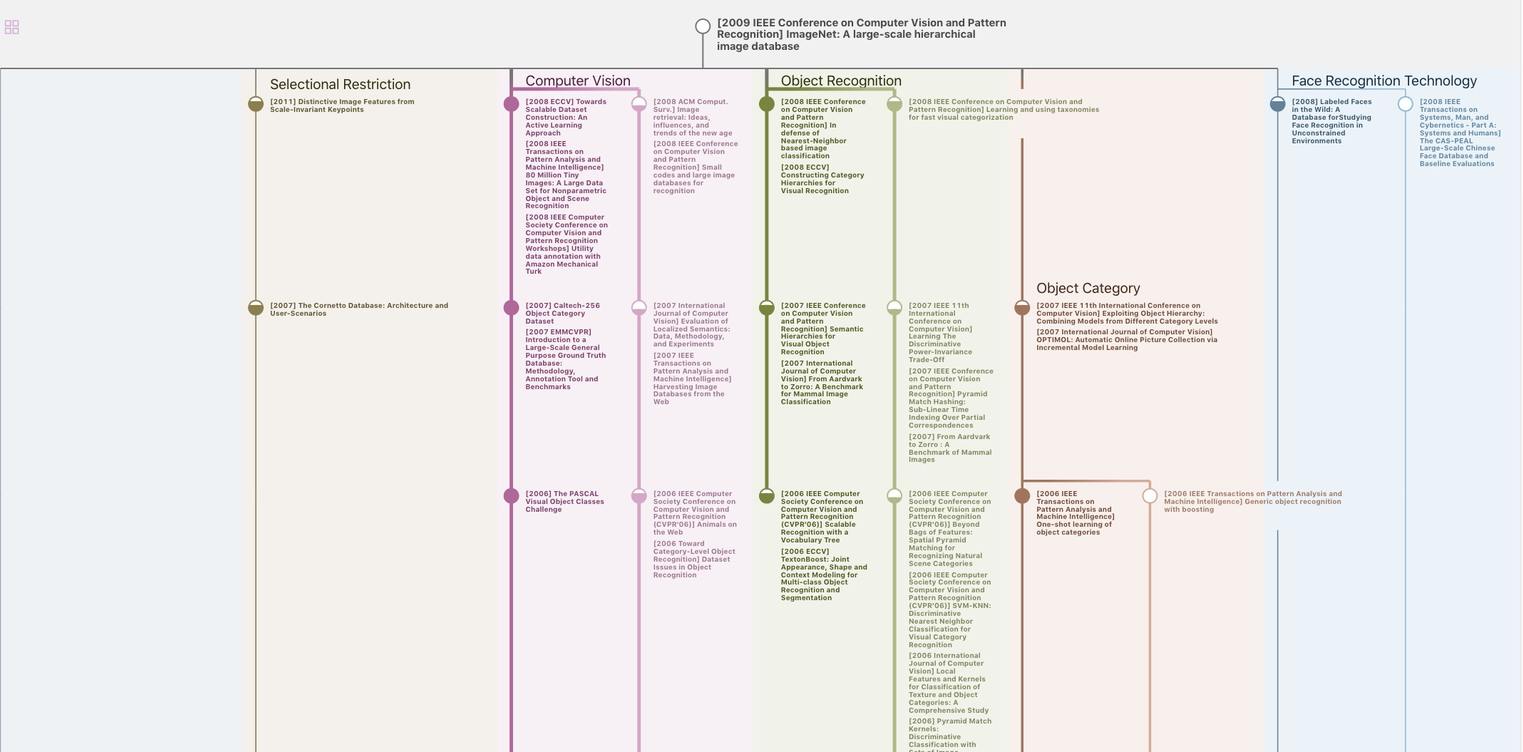
生成溯源树,研究论文发展脉络
Chat Paper
正在生成论文摘要