EDFIDepth: enriched multi-path vision transformer feature interaction networks for monocular depth estimation
The Journal of Supercomputing(2024)
Abstract
Monocular depth estimation (MDE) aims to predict pixel-level dense depth maps from a single RGB image. Some recent approaches mainly rely on encoder–decoder architectures to capture and process multi-scale features. However, they usually exploit heavier network at the expense of computational costs to obtain high-quality depth maps. In this paper, we propose a novel enriched multi-path vision transformer feature interaction network with an encoder–decoder architecture, denoted as EDFIDepth , which seeks a balance between computational costs and performance rather than pursuing the highest accuracy or extremely lightweight models. Specifically, an encoder called MPViT-D, incorporating multi-path vision transformer and a deep convolution module, is introduced to extract diverse features with both fine and coarse details at the same feature level with fewer parameters. Subsequently, we propose a lightweight decoder comprising two effective modules to establish multi-scale feature interaction: an encoder–decoder cross-feature matching (ED-CFM) module and a channel-level feature fusion (CLFF) module. The ED-CFM module is to establish connections between encoder–decoder features through a dual-path structure, where a cross-attention mechanism is deployed to enhance the relevance of multi-scale complementary depth information. Meanwhile, the CLFF module utilizes a channel attention mechanism to further fuse crucial depth information within the channels, thereby improving the accuracy of depth estimation. Extensive experiments on the indoor dataset NYUv2 and the outdoor dataset KITTI demonstrate that our method can achieve comparable state-of-the-art (SOTA) results while significantly reducing the number of trainable parameters. Our codes and approach are available at https://github.com/Zhangmg123/EDFIDEpth.
MoreTranslated text
Key words
Attention mechanism,Diversified feature extraction,Feature interaction,Monocular depth estimation
AI Read Science
Must-Reading Tree
Example
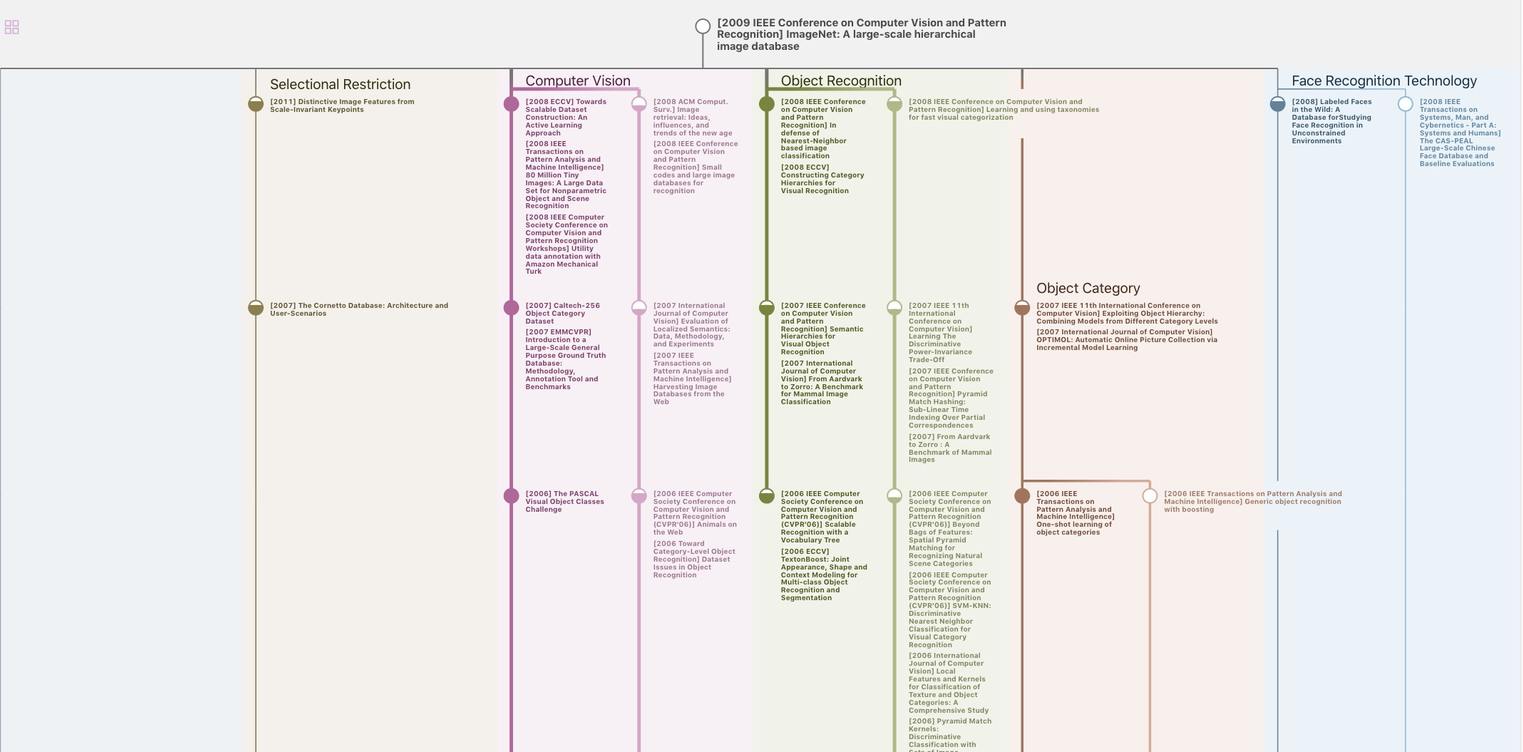
Generate MRT to find the research sequence of this paper
Chat Paper
Summary is being generated by the instructions you defined