Research on Multi-Feature Fusion False Review Detection Based on DynDistilBERT-BiLSTM-CNN
IEEE Internet of Things Journal(2024)
摘要
With the rapid expansion of e-commerce and social media platforms, the prevalence of fake reviews has become increasingly problematic, misleading consumers and harming both the reputation of businesses and fair market competition. This paper aims to develop a more effective technological solution to accurately identify and filter deceptive reviews, ensuring a truthful shopping and communication environment for consumers. Initially, a multi-feature fusion strategy is introduced, integrating text characteristics of reviews, reviewer behavior, and product information. Through a parameterized attention mechanism, the model meticulously assigns weights to various influential features, thereby enhancing the detection of deceptive reviews.Furthermore, a composite architecture, DynDistilBERT-BiLSTM-CNN, is proposed. DynDistilBERT employs a control gate to assess the complexity of the input text and the required processing power in real-time during model forward propagation, dynamically selecting active layers within DistilBERT. This selection process is optimized with a hierarchical training strategy to minimize additional computational overhead. BiLSTM excels in processing sequential data, capturing temporal text features, while CNN focuses on identifying local text features. This approach reduces computational resource consumption for simpler tasks while maintaining high accuracy for complex tasks, recognizing both local features and contextual relationships in text.Extensive testing on the Amazon dataset, compared to models like ALBERT, SpanBERT, DistilBERT, and RoBERTa, demonstrates that our model achieves accuracy improvements of approximately 5.7%, 5.2%, 5.0%, and 3.9%, with a peak accuracy of 92.6%. These findings underscore the effectiveness of the multi-feature fusion strategy and the superior performance of the DynDistilBERT-BiLSTM-CNN architecture in handling complex textual data.
更多查看译文
关键词
DynDistilBERT model,multi-feature fusion,false review detection,deep learning,text analysis
AI 理解论文
溯源树
样例
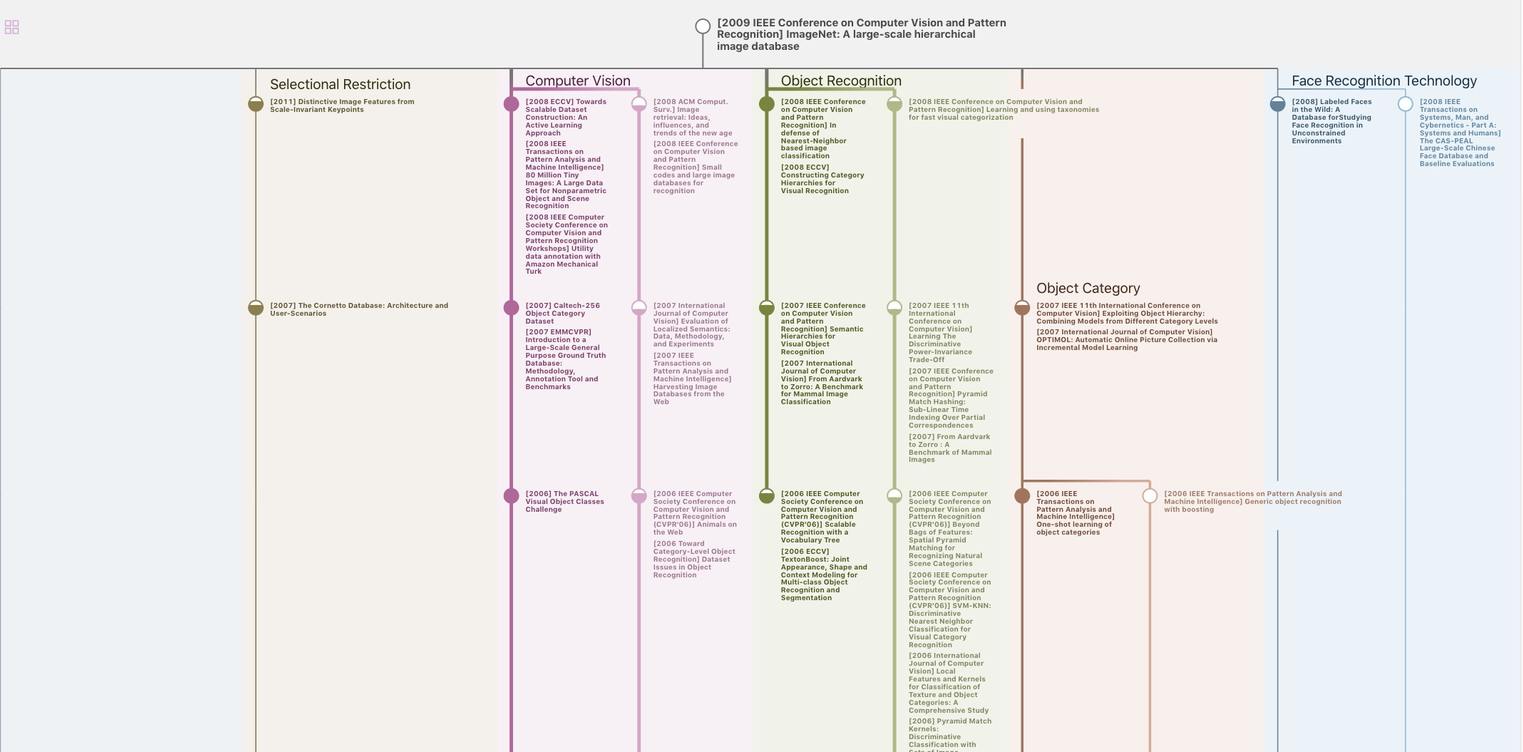
生成溯源树,研究论文发展脉络
Chat Paper
正在生成论文摘要