Location Prediction Using Bayesian Optimization LSTM for RIS-Assisted Wireless Communications
IEEE Transactions on Vehicular Technology(2024)
摘要
Reconfigurable intelligent surface (RIS) represent a novel form of electromagnetic metamaterial that have been extensively studied for user equipment (UE) positioning by exploiting the multipath propagation of signals. A novel RIS-assisted localization prediction (RLP) method based on Bayesian optimization and long short-term memory (BO-LSTM) has been proposed in this paper. This method capitalizes on the predictive advantages of LSTM for data sequence and RIS's flexible and controllable multidimensional feature parameters, establishing a mobile UE localization model in an RIS-assisted wireless communications system based on the interplay between time slot transmission power and user location information. In order to provide a more stable communication environment for data collection during the localization process, a power allocation optimization (PAO) method is proposed for maximizing time slot channel capacity in the RLP system based on the number of RIS reflection elements. The study conducts a thorough comparison of simulation results of BO-LSTM, convolutional neural networks (CNN)-LSTM and improved bidirectional LSTM (BiLSTM) combined with Adaptive boost, employing adaptive moment estimation (Adam) and stochastic gradient descent with momentum (SGDM) optimizers. Experimental results demonstrate that the BO-LSTM-based RLP method exhibits improved prediction accuracy. These findings suggest the effectiveness of the proposed method and highlight its potential for further enhancement.
更多查看译文
关键词
reconfigurable intelligent surface,Bayesian optimization LSTM,location prediction,wireless communications
AI 理解论文
溯源树
样例
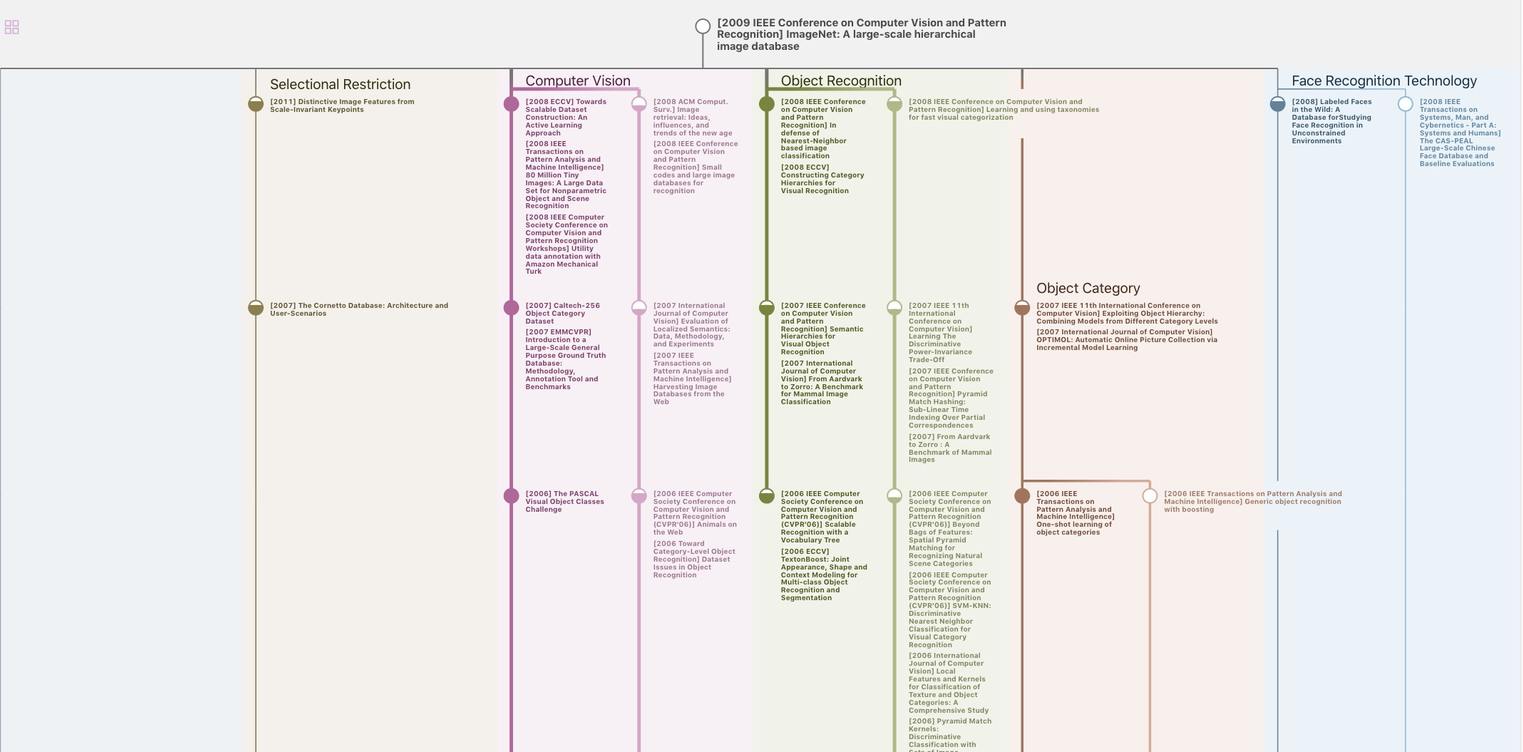
生成溯源树,研究论文发展脉络
Chat Paper
正在生成论文摘要