A Quantum Neural Network-Based Approach to Power Quality Disturbances Detection and Recognition
arxiv(2024)
摘要
Power quality disturbances (PQDs) significantly impact the stability and reliability of power systems, necessitating accurate and efficient detection and recognition methods. While numerous classical algorithms for PQDs detection and recognition have been extensively studied and applied, related work in the quantum domain is still in its infancy. In this paper, an improved quantum neural networks (QNN) model for PQDs detection and recognition is proposed. Specifically, the model constructs a quantum circuit comprising data qubits and ancilla qubits. Classical data is transformed into quantum data by embedding it into data qubits via the encoding layer. Subsequently, parametric quantum gates are utilized to form the variational layer, which facilitates qubit information transformation, thereby extracting essential feature information for detection and recognition. The expected value is obtained by measuring ancilla qubits, enabling the completion of disturbance classification based on this expected value. An analysis reveals that the runtime and space complexities of the QNN are $O\left ( poly\left ( N \right ) \right )$ and $O\left ( N \right )$, respectively. Extensive experiments validate the feasibility and superiority of the proposed model in PQD detection and recognition. The model achieves accuracies of 99.75\%, 97.85\% and 95.5\% in experiments involving the detection of disturbances, recognition of seven single disturbances, and recognition of ten mixed disturbances, respectively. Additionally, noise simulation and comparative experiments demonstrate that the proposed model exhibits robust anti-noise capabilities, requires few training parameters, and maintains high accuracy.
更多查看译文
AI 理解论文
溯源树
样例
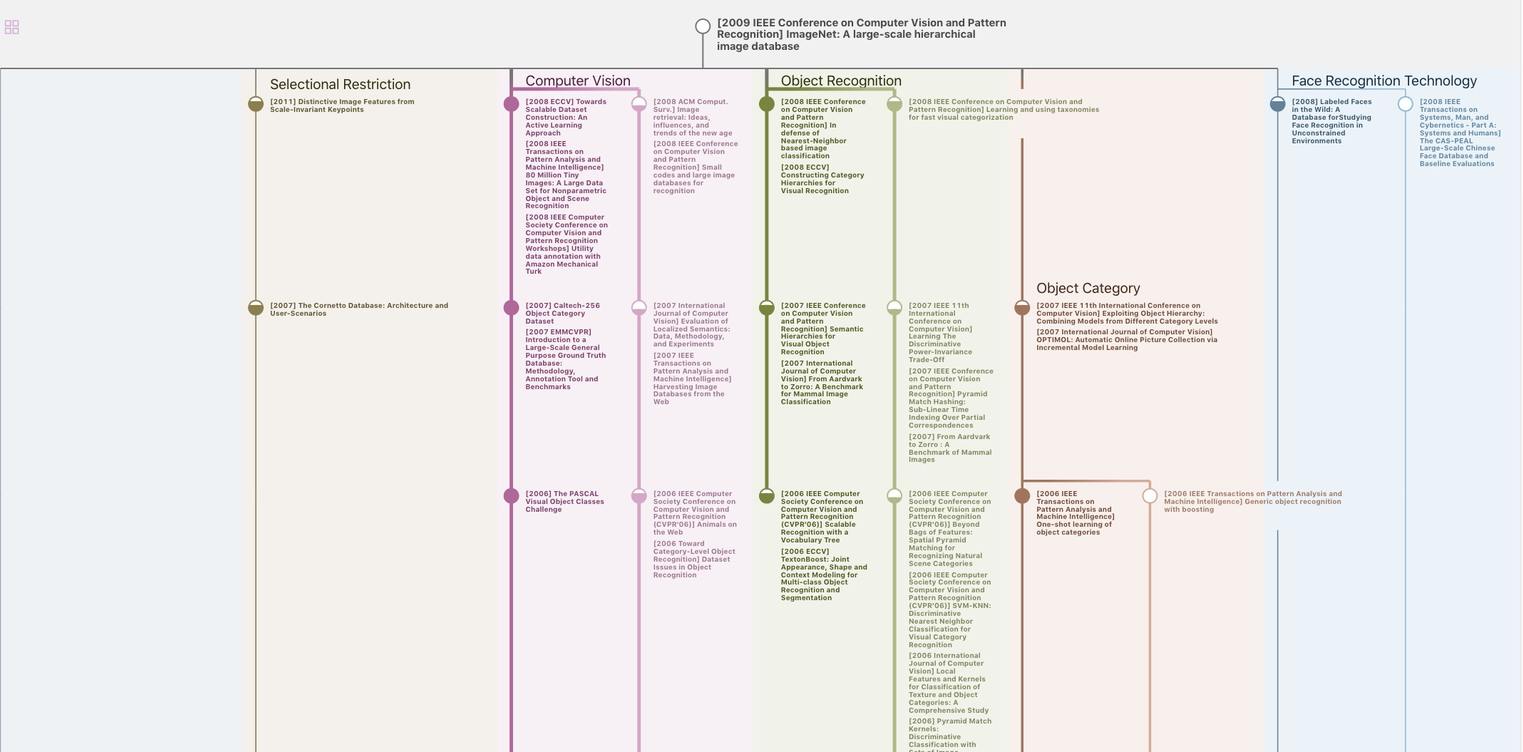
生成溯源树,研究论文发展脉络
Chat Paper
正在生成论文摘要