Private Stochastic Convex Optimization with Heavy Tails: Near-Optimality from Simple Reductions
arxiv(2024)
摘要
We study the problem of differentially private stochastic convex optimization
(DP-SCO) with heavy-tailed gradients, where we assume a k^th-moment
bound on the Lipschitz constants of sample functions rather than a uniform
bound. We propose a new reduction-based approach that enables us to obtain the
first optimal rates (up to logarithmic factors) in the heavy-tailed setting,
achieving error G_2 ·1/√(n) + G_k · (√(d)/nϵ)^1 - 1/k under (ϵ, δ)-approximate
differential privacy, up to a mild (1/δ) factor,
where G_2^2 and G_k^k are the 2^nd and k^th moment
bounds on sample Lipschitz constants, nearly-matching a lower bound of [Lowy
and Razaviyayn 2023].
We further give a suite of private algorithms in the heavy-tailed setting
which improve upon our basic result under additional assumptions, including an
optimal algorithm under a known-Lipschitz constant assumption, a near-linear
time algorithm for smooth functions, and an optimal linear time algorithm for
smooth generalized linear models.
更多查看译文
AI 理解论文
溯源树
样例
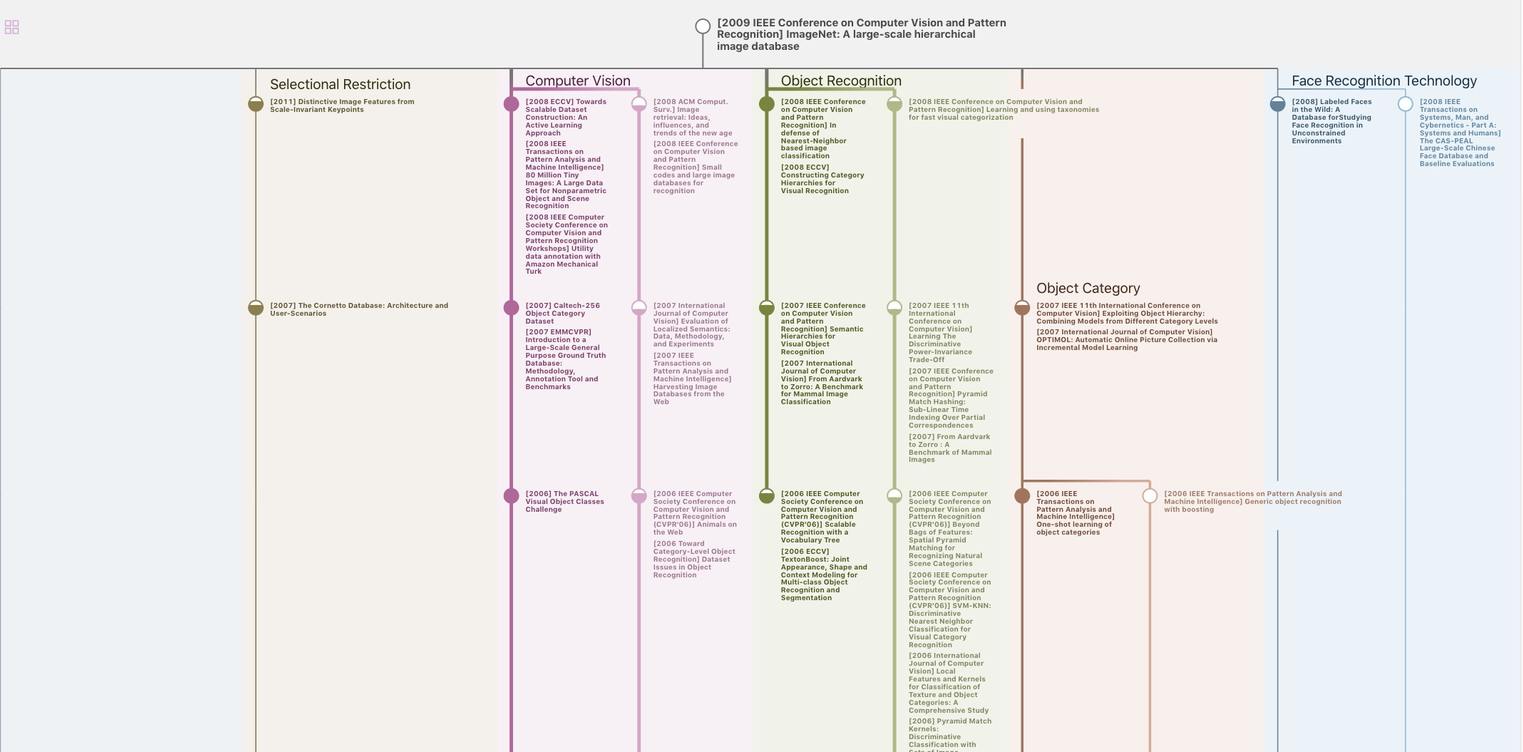
生成溯源树,研究论文发展脉络
Chat Paper
正在生成论文摘要