Longitudinal risk prediction for pediatric glioma with temporal deep learning.
medRxiv : the preprint server for health sciences(2024)
Abstract
Pediatric glioma recurrence can cause morbidity and mortality; however, recurrence pattern and severity are heterogeneous and challenging to predict with established clinical and genomic markers. Resultingly, almost all children undergo frequent, long-term, magnetic resonance (MR) brain surveillance regardless of individual recurrence risk. Deep learning analysis of longitudinal MR may be an effective approach for improving individualized recurrence prediction in gliomas and other cancers but has thus far been infeasible with current frameworks. Here, we propose a self-supervised, deep learning approach to longitudinal medical imaging analysis, temporal learning, that models the spatiotemporal information from a patient's current and prior brain MRs to predict future recurrence. We apply temporal learning to pediatric glioma surveillance imaging for 715 patients (3,994 scans) from four distinct clinical settings. We find that longitudinal imaging analysis with temporal learning improves recurrence prediction performance by up to 41% compared to traditional approaches, with improvements in performance in both low- and high-grade glioma. We find that recurrence prediction accuracy increases incrementally with the number of historical scans available per patient. Temporal deep learning may enable point-of-care decision-support for pediatric brain tumors and be adaptable more broadly to patients with other cancers and chronic diseases undergoing surveillance imaging.
MoreTranslated text
AI Read Science
Must-Reading Tree
Example
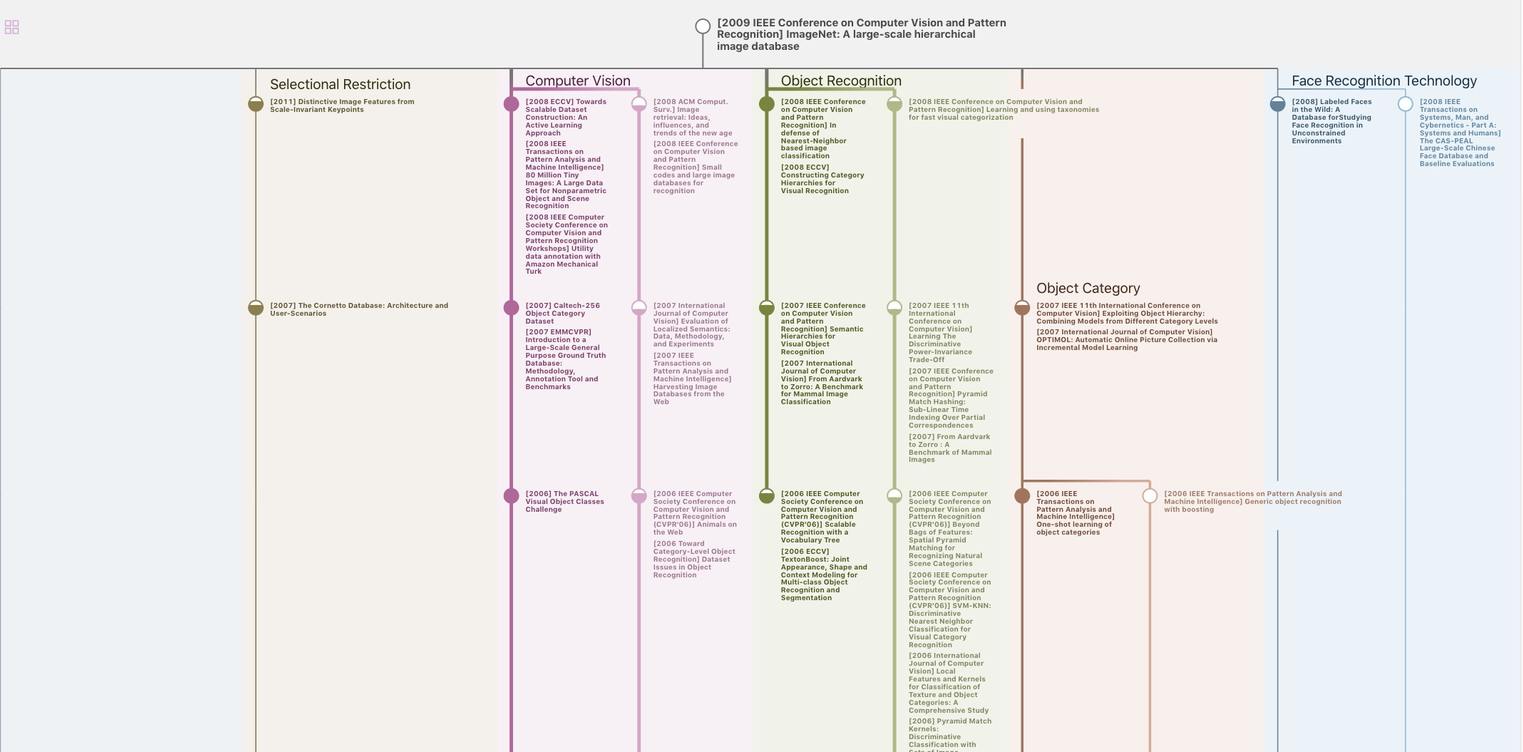
Generate MRT to find the research sequence of this paper
Chat Paper
Summary is being generated by the instructions you defined