Understanding Localization by a Tailored GPT
Proceedings of the 22nd Annual International Conference on Mobile Systems, Applications and Services(2024)
摘要
Conventional deep learning approaches for indoor localization often suffer from their reliance on high-quality training samples and display limited adaptability across varied scenarios. To address these challenges, we repurpose the Transformer model, celebrated for its profound contextual insights, to explore the underlying principles of indoor localization. Our microbenchmark results compellingly demonstrate the superiority of our approach, showing improvements of 30% to 70% across a diverse set of 50 scenarios compared to other state-of-the-art methods. In conclusion, we propose a specialized Generative Pre-training Transformer (GPT) variant, termed LocGPT, configured with 36 million parameters that are tailored to facilitate transfer learning. By fine-tuning this pre-trained model, we achieve near-par accuracy using merely half the conventional dataset, thereby heralding a pioneering stride in transfer learning within the indoor localization domain.
更多查看译文
AI 理解论文
溯源树
样例
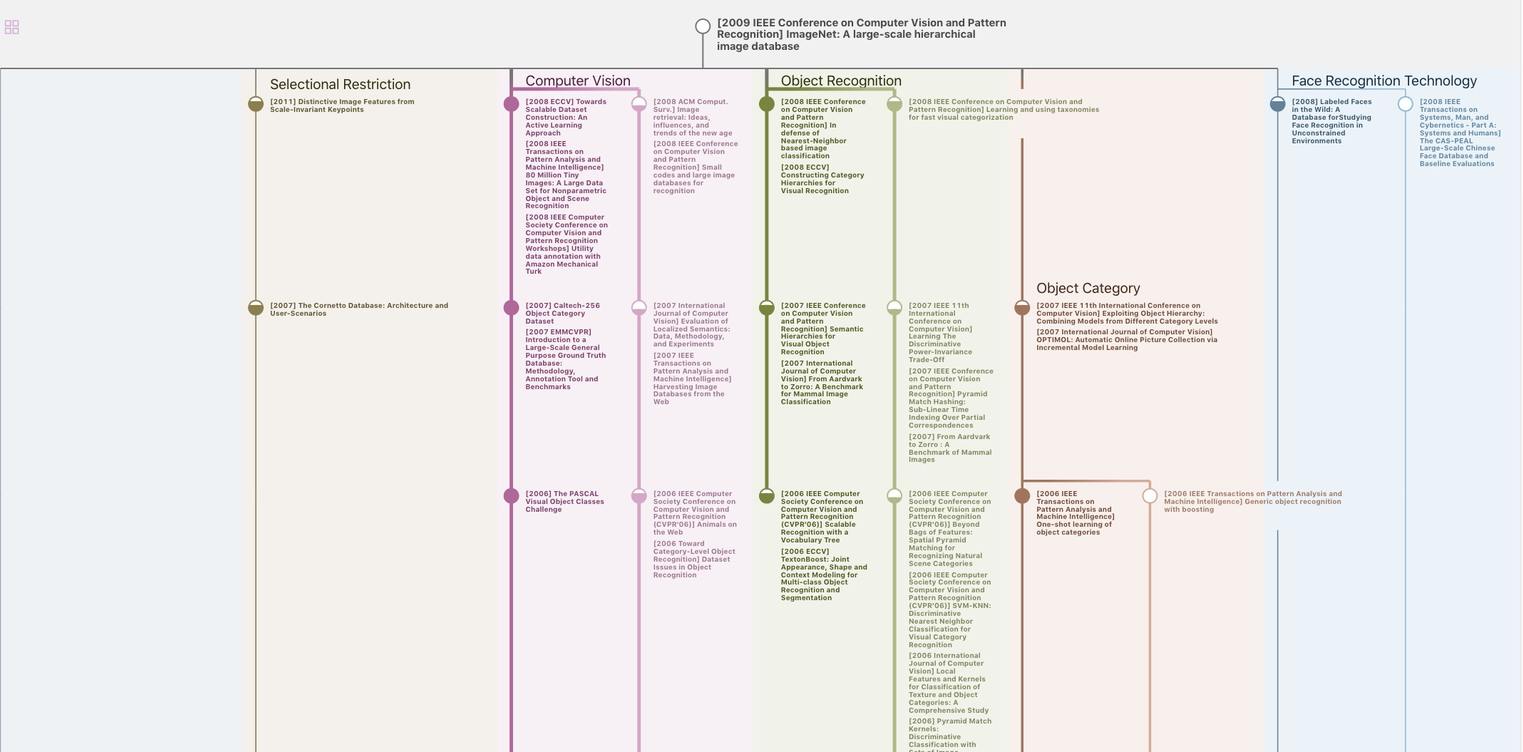
生成溯源树,研究论文发展脉络
Chat Paper
正在生成论文摘要