Optimal weighting factor design based on entropy technique in finite control set model predictive torque control for electric drive applications.
Scientific reports(2024)
Abstract
In the conventional finite control set model predictive torque control, the cost function consists of different control objectives with varying units of measurements. Due to presence of diverse variables in cost function, weighting factors are used to set the relative importance of these objectives. However, selection of these weighting factors in predictive control of electric drives and power converters still remains an open research challenge. Improper selection of weighting factors can lead to deterioration of the controller performance. This work proposes a novel weighting factor tuning method based on the Multi-Criteria-Decision-Making (MCDM) technique called the Entropy method. This technique has several advantages for multi-objective problem optimization. It provides a quantitive approach and incorporates uncertainties and adaptability to assess the relative importance of different criteria or objectives. This technique performs the online tuning of the weighting factor by forming a data set of the control objectives, i.e., electromagnetic torque and stator flux magnitude. After obtaining the error set of control variables, the objective matrix is normalized, and the entropy technique is applied to design the corresponding weights. An experimental setup based on the dSpace dS1104 controller is used to validate the effectiveness of the proposed method for a two-level, three-phase voltage source inverter (2L-3P) fed induction motor drive. The dynamic response of the proposed technique is compared with the previously proposed MCDM-based weighting factor tuning technique and conventional MPTC. The results reveal that the proposed method provides an improved dynamic response of the drive under changing operating conditions with a reduction of 28% in computational burden and 38% in total harmonic distortion, respectively.
MoreTranslated text
Key words
Model predictive torque control (MTPC),Weighting factor,Multi-decision making criteria,Entropy method,Induction motor
AI Read Science
Must-Reading Tree
Example
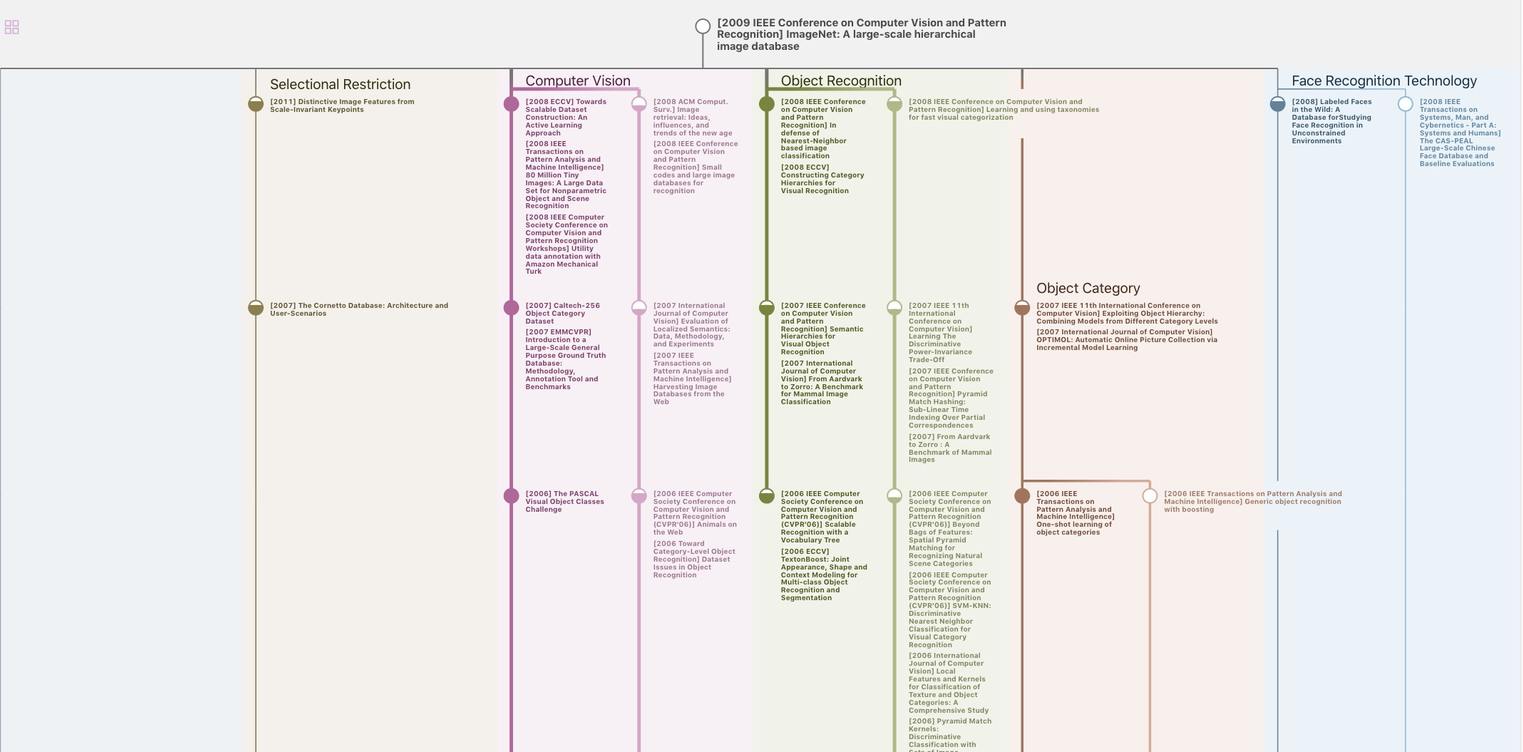
Generate MRT to find the research sequence of this paper
Chat Paper
Summary is being generated by the instructions you defined