Reproducible and high sample throughput isomiR next-generation sequencing for cancer diagnosis.
Journal of Clinical Oncology(2024)
摘要
e15013 Background: Next-generation sequencing (NGS) can produce up to 6 Tb of data per run with single-nucleotide accuracy, making it ideal for quantifying isomiRs, which encompass both canonical miRNAs and their variants, for clinical applications. However, NGS has poor reproducibility and low sample throughput in quantifying circulating isomiRs due to significant technical variations and the limitations of the multiplex strategy, as evidenced by the fact that no isomiR NGS technique has been successfully used to diagnose cancer. Methods: To address these challenges, a library construction method including a dual unique-dual-index (DUDI) technology was developed. DUDI uses a pair of Inner UDI (IUDI) and outer UDI (OUDI) to label a sample. Twelve independent batches of isomiR NGS were carried out, including three repeated batches. Each batch included 100 gastric cancer and 100 control plasma samples. Batch effect, correlation coefficient (R), and principal component (PCA) analyses were used to evaluate technical reproducibility. Machine learning binary classification was used to assess biological reproducibility, with each pair of batch data serving interchangeably as both training and testing data. Results: In this multicenter study, over 700G of isomiR data were generated from 402 gastric cancer and 498 control samples, with a maximum error rate of 1 in 7 million isomiRs being assigned to wrong samples. The PCA plot indicates high technical reproducibility across the three repeated batches, shown by the extensive intermingling of data points from each batch and the lack of distinct batch-wise clustering. This observation is reinforced by that the R value for each of 239 isomiRs between the repeated batches are close to 1. While the mutual machine learning validations between the repeated batches yielded ~95% accuracy, indicating high biological reproducibility. The accuracies of the validations between the different batches of different samples range from 70% to 82%. The lower accuracy is as expected, given the high genetic heterogeneity of cancer and the small sample size. Furthermore, the IsomiR differentiated expression profiles from the current NGS study closely match those from prior qPCR studies. Conclusions: The DUDI library construction method can produce reproducible high sample throughput NGS data, yet it is cost-effective and straightforward. The maximum number of samples that can be multiplexed in an NGS project is almost one million, i.e., 976 * 976, as IUDI and OUDI can be any of the 976 designed DUDIs. This number far exceeds the high sample throughput requirements of any NGS application. While the capability to distinguish true biological variations of IsomiRs from technical noise, demonstrated by the high technical and biological reproducibility and concordance with the qPCR data, enables the development of robust machine learning algorithms for cancer diagnostics.
更多查看译文
AI 理解论文
溯源树
样例
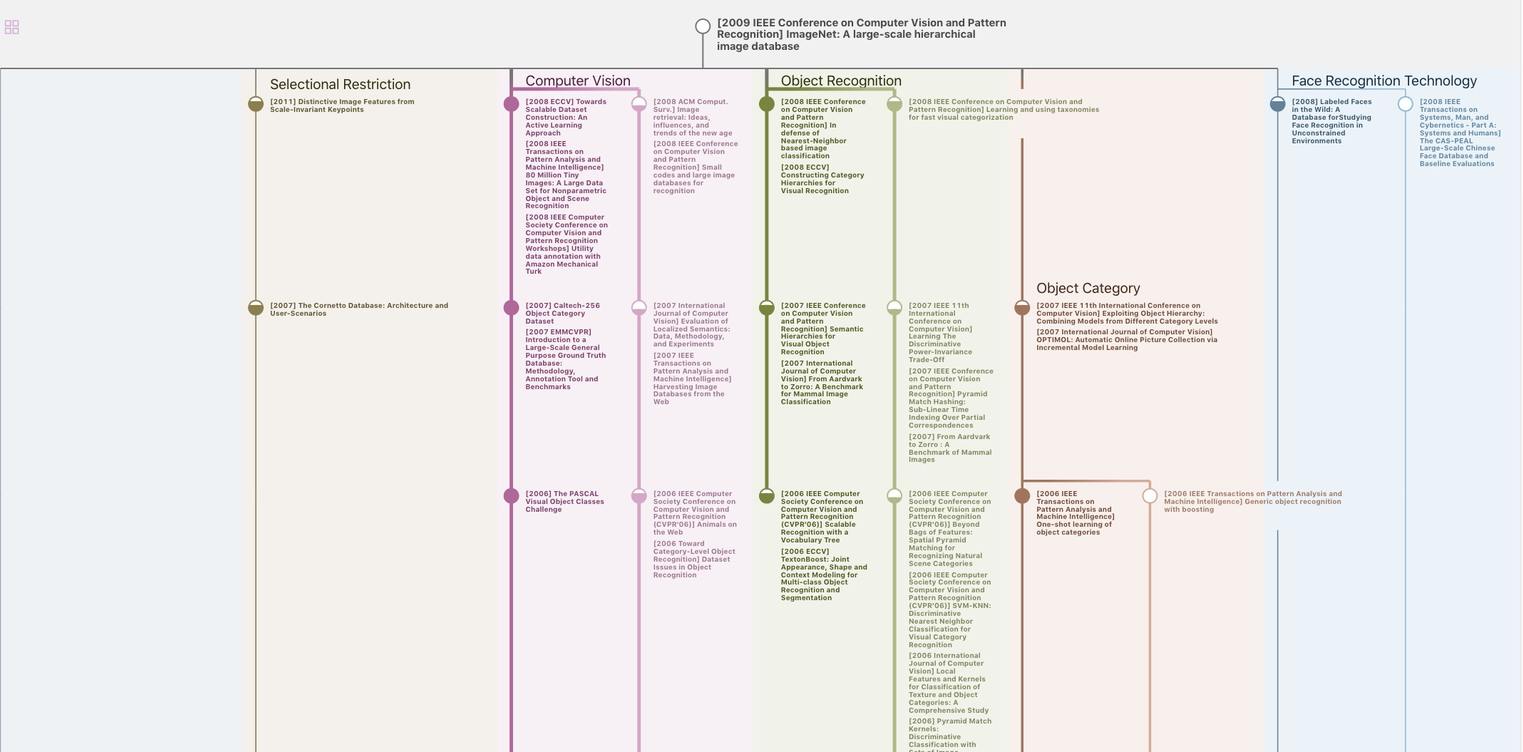
生成溯源树,研究论文发展脉络
Chat Paper
正在生成论文摘要