Mapping global grassland dynamics 2000—2022 at 30m spatial resolution using spatiotemporal Machine Learning
crossref(2024)
Abstract
The paper describes the production and evaluation of global grassland dynamics mapped annually for 2000-2022 at 30~m spatial resolution. The dataset showing the spatiotemporal distribution of cultivated and natural/semi-natural grassland classes was produced by using GLAD Landsat ARD-2 image archive, accompanied by climatic, landform and proximity covariates, spatiotemporal machine learning (per-class Random Forest) and over 2.3M reference samples (visually interpreted in Very High Resolution imagery). Custom probability thresholds (based on five-fold spatial cross-validation) were used to derive dominant class maps with balanced precision and recall values, 0.64 and 0.75 for cultivated and natural/semi-natural grassland, respectively. The produced maps (about 4~TB in size) are available under an open data license as Cloud-Optimized GeoTIFFs and as Google Earth Engine assets. The suggested uses of data include (1) integration with other compatible land cover products and (2) tracking the intensity and drivers of conversion of land to cultivated grasslands and from natural / semi-natural grasslands into other land use systems.
MoreTranslated text
AI Read Science
Must-Reading Tree
Example
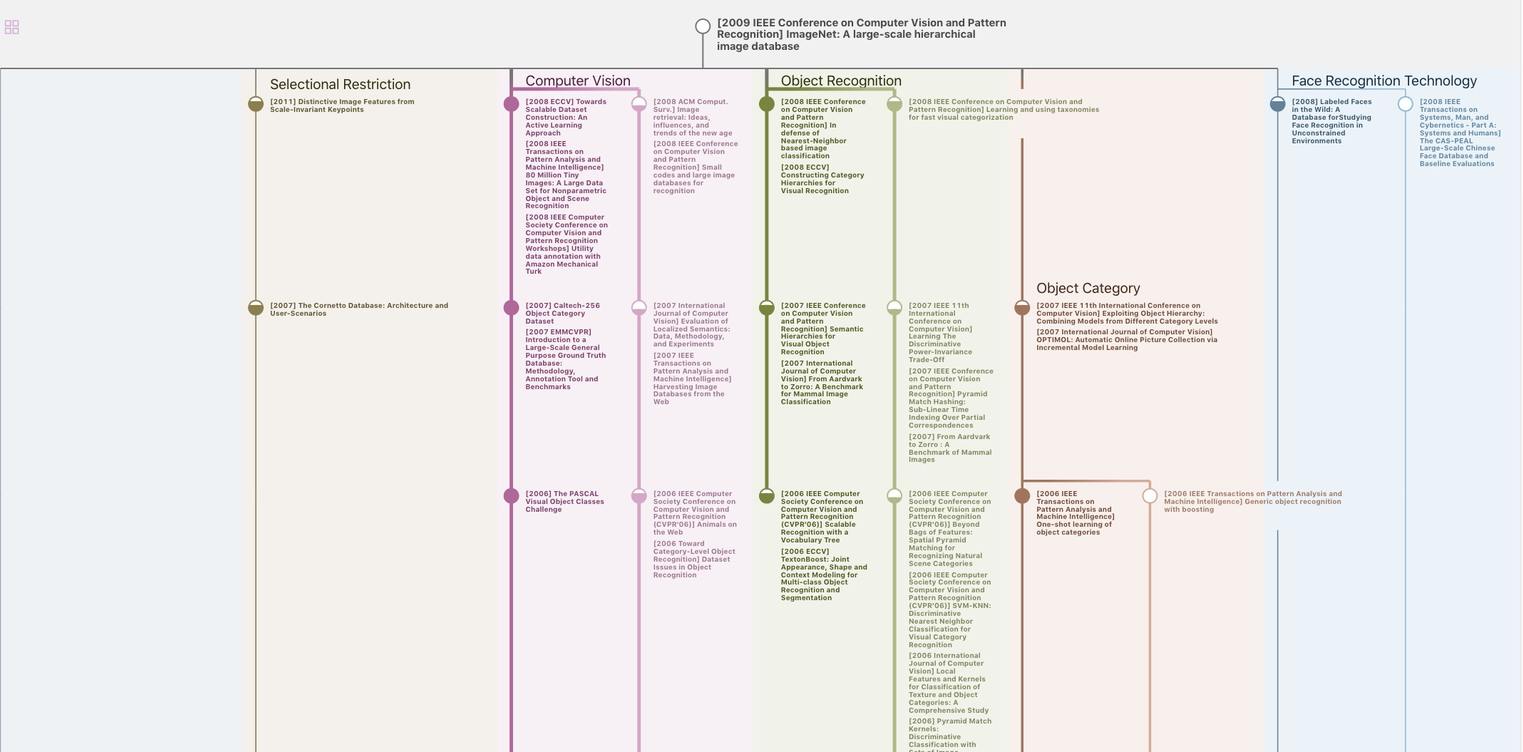
Generate MRT to find the research sequence of this paper
Chat Paper
Summary is being generated by the instructions you defined