CPS-3WS: A Critical Pattern Supported Three-way Sampling Method for Classifying Class-overlapped Imbalanced Data
Information Sciences(2024)
摘要
Class-imbalance problem widely exists in real applications ranging from medial diagnosis to economic fraud detection, etc. As one of the mainstream techniques in dealing with imbalanced data, SMOTE (Synthetic Minority Over-sampling TEchnique) and its extensions mainly rebalance the datasets via generation of observations in specific regions with various adapted strategies. Many of them do not consider the cost of role assignment of samples, and the intractable data complexity (overlap, small disjuncts, etc.) poses additional challenges to them. This paper proposes a critical pattern supported three-way sampling method (CPS-3WS) for classifying class-overlapped imbalanced data, introducing the philosophy of thinking in threes to effective classification in imbalanced learning. Specifically, CPS-3WS uses a three-way sample partition strategy with the Bayes posterior probability by dividing majority and minority classes into three disjoint subsets: risky, critical and safe patterns. CPS-3WS conducts a three-way hybrid sampling through (i) evaluating the risky majority pattern to be eliminated and (ii) selecting critical minority pattern to synthesize new samples under local information constraint. Extensive experiments on 42 UCI benchmark datasets demonstrate the superiority of the proposed CPS-3WS compared with 11 data-level methods. The source code of CPS-3WS is available at https://github.com/ytyancp/CPS-3WS.
更多查看译文
关键词
Three-way sampling
AI 理解论文
溯源树
样例
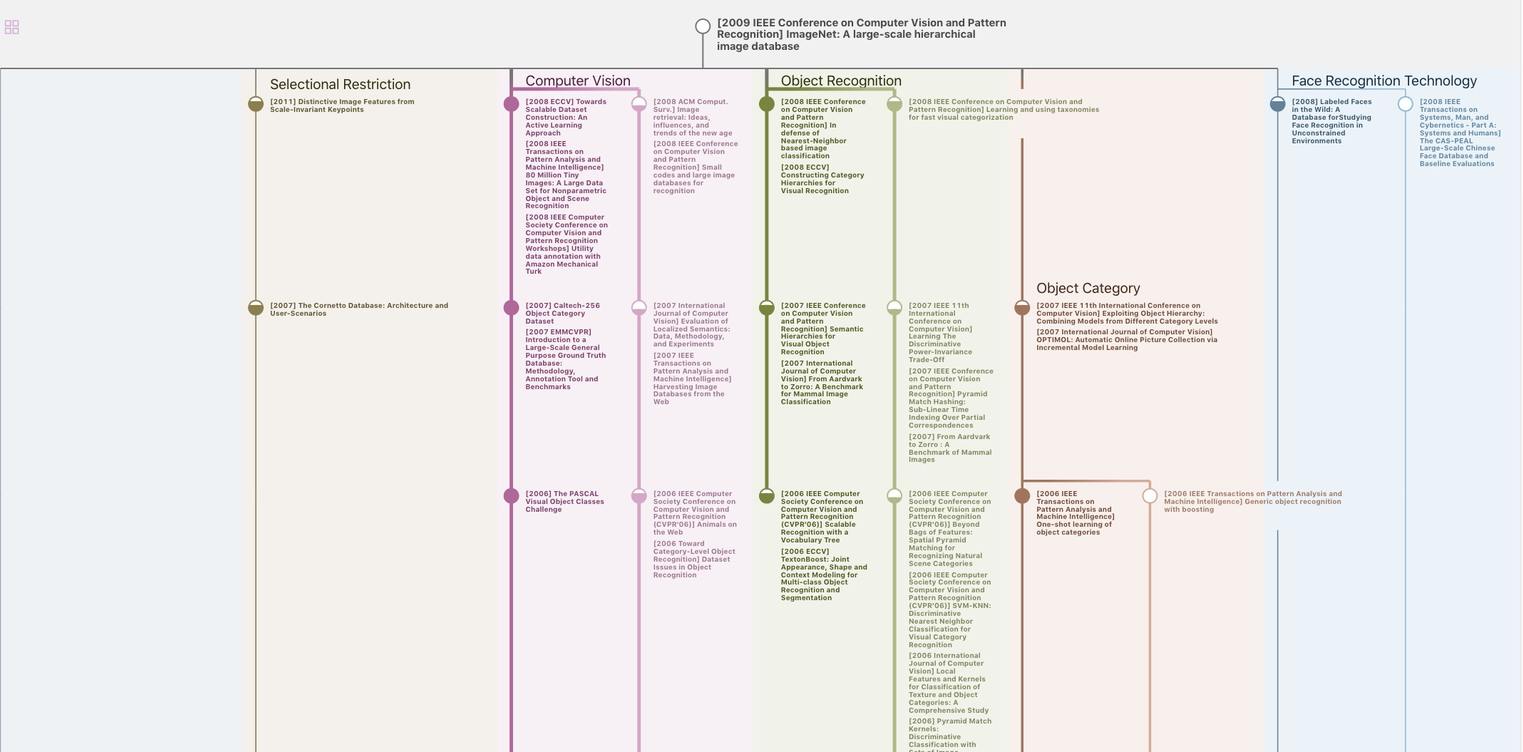
生成溯源树,研究论文发展脉络
Chat Paper
正在生成论文摘要