Rock discontinuities characterization from large-scale point clouds using a point-based deep learning method
Engineering Geology(2024)
摘要
Rock discontinuities are essential for the mechanical behavior and stability of rock mass. Previous approaches for characterizing discontinuities either rely on limited handcrafted features (point normals, point curvatures, point densities, and so on) or fail to classify discontinuities, making them unsuitable for complex and large-scale scenes. To cope with these problems, an end-to-end point-based deep learning method that can automatically learn rich and high-dimensional features and classify discontinuities was developed in this study. Firstly, a roadcut and part of a natural slope were selected to train the developed network and assess its performance. Subsequently, the trained network was used to classify the remaining part of the slope. Finally, the “Density-Based Scan Algorithm with Noise” (DBSCAN) and principal component analysis (PCA) algorithms were employed to extract individual discontinuities and calculate their orientations. The two cases achieved a global accuracy (GA) of 97.25% and 94.56%, respectively, and a mean intersection over union (MIoU) of 93.77% and 88.66%, respectively, indicating the excellent performance of the network. Meanwhile, the average error in dip angle and dip direction was 0.67° and 3.33°, respectively, proving the characterization ability of the developed method was satisfactory. Furthermore, the presented method exhibits strong robustness and the potential to characterize large-scale rock discontinuities with noise. This method facilitates the application of deep learning in geosciences and provides geologists and geological engineers with a new idea for rock discontinuity characterization.
更多查看译文
关键词
Rock discontinuities,3D point clouds,Discontinuity characterization,Orientation measurement,Deep learning,End to end
AI 理解论文
溯源树
样例
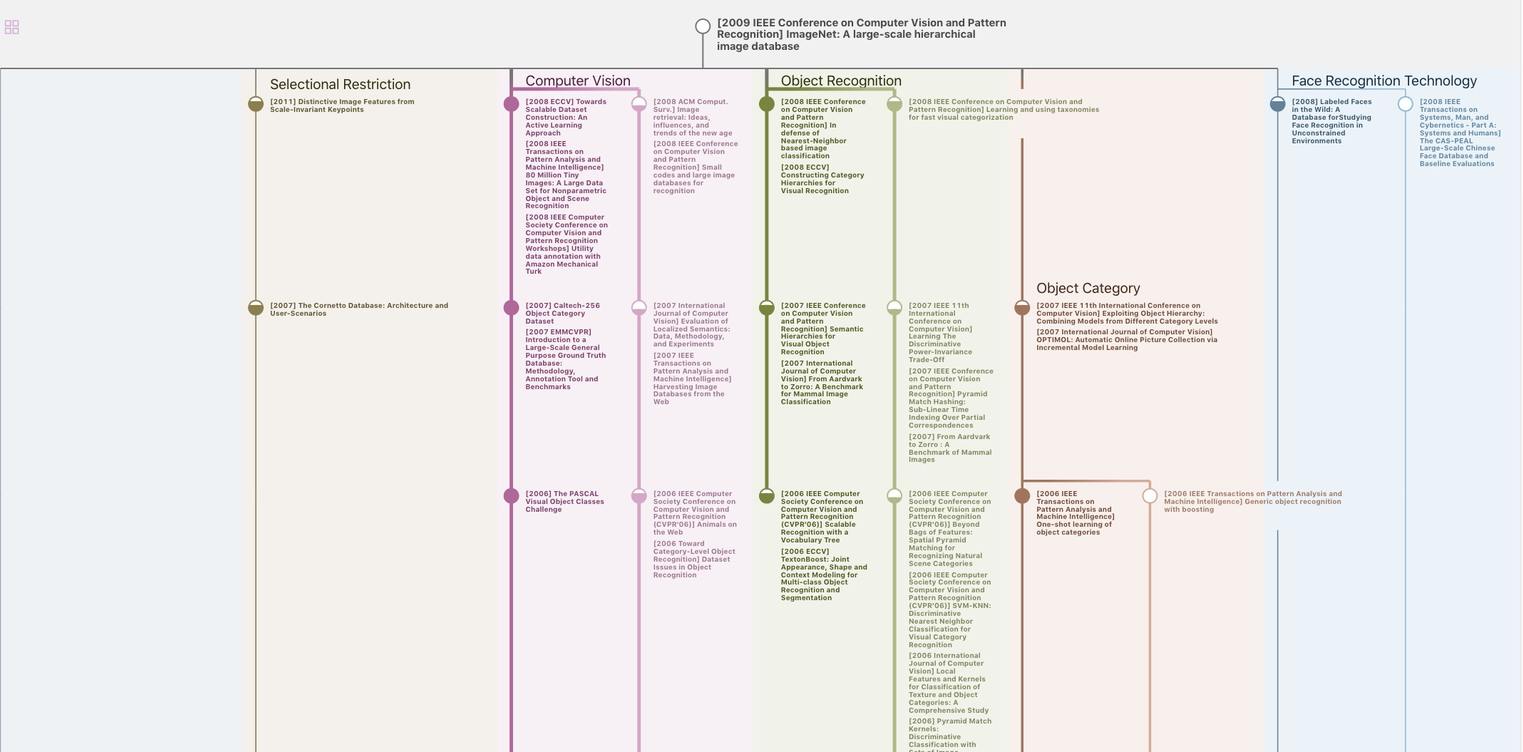
生成溯源树,研究论文发展脉络
Chat Paper
正在生成论文摘要