Impact of artificial intelligence (AI) decision support on clinical trial participation: A before-after implementation study on a nationwide molecular tumor board.
Journal of Clinical Oncology(2024)
Abstract
1557 Background: Molecular Tumor Boards (MTB) have become the standard for deriving consensus therapy (Rx) recommendations (rec) for patients (pts) with advanced cancer undergoing comprehensive genomic profiling (CGP). However, finding matched Rx and trial options remains laborious, needing informatics strategies. Methods: We examined the outcomes before and after implementation of a decision support system (DSS) at the MTB of the Australian Molecular Screening & Therapeutics program (MoST, ACTRN12616000908437), enrolling pts with refractory solid tumors and linking CGP results to Rx and trials through rec on clinical reports. The DSS comprises a variant interpretation pipeline, a symbolic AI system integrating the TOPOGRAPH database and an annotated trials registry, and a web platform used by the MTB for producing reports shortlisting matching Rx and therapeutic trials. The primary endpoint is the cumulative participation rate (CPR) in genomic matched trials (excluding MoST-related trials) in pts who received Rx after CGP. The secondary endpoints are the CPR in any trial, and overall survival (OS) from MTB. The Fine-Gray model was used to estimate the subdistribution hazard ratios (sHR) for between-group differences in participation. Kaplan-Meier and Cox regression were used for OS analysis. The time-to-event analyses were adjusted for age, ECOG, cancer type, lines of prior rx, and lead-time bias (OS only). Results: In 2203 of 5186 pts (42.5%) received ≥ 1 Rx after CGP, 971 pts were reviewed at MTB before and 1272 after DSS implementation in Sep 2020. At 3, 6 and 12 months (m) after enrolment, CPR in matched trials were 3.2%, 5.0%, and 6.8% before implementation and 3.6%, 5.8%, and 8.9% afterwards (sHR 1.19, 95% CI 0.90–1.57, p=0.23). Pts who received an MTB rec were more likely to participate in a matched trial (sHR 4.75, 3.13–7.23, p<0.001). Notably, DSS specifically increased the likelihood of trial participation following MTB rec (interaction sHR 2.69, 1.02–7.07, p=0.045), with 12m CPR increasing from 10.0% to 12.2% after implementation of the DSS in pts with a MTB rec, whilst for pts without a MTB rec, the CPR fell from 3.1% to 1.6% after implementation. No association was seen between participation in any trials and MTB recs (sHR 1.12, 0.95–1.32) or implementation (sHR 0.95, 0.81–1.11; CPR pre 21.6% v post 23.7% at 12m). No OS difference was seen comparing before with after implementation of the DSS (median 16.7 v 15.3m, adjusted HR 0.97, 0.87–1.08). However, pts receiving genomic matched Rx had a longer OS (median 20.1 v 15.0m unmatched, HR 0.76, p=0.002). Conclusions: The observed interaction between the implementation of DSS and MTB rec suggests there is effect modification in participation rates of pts in genomic matched trials, warranting exploration into how AI might enhance trial participation among pts with incurable cancers undergoing CGP.
MoreTranslated text
AI Read Science
Must-Reading Tree
Example
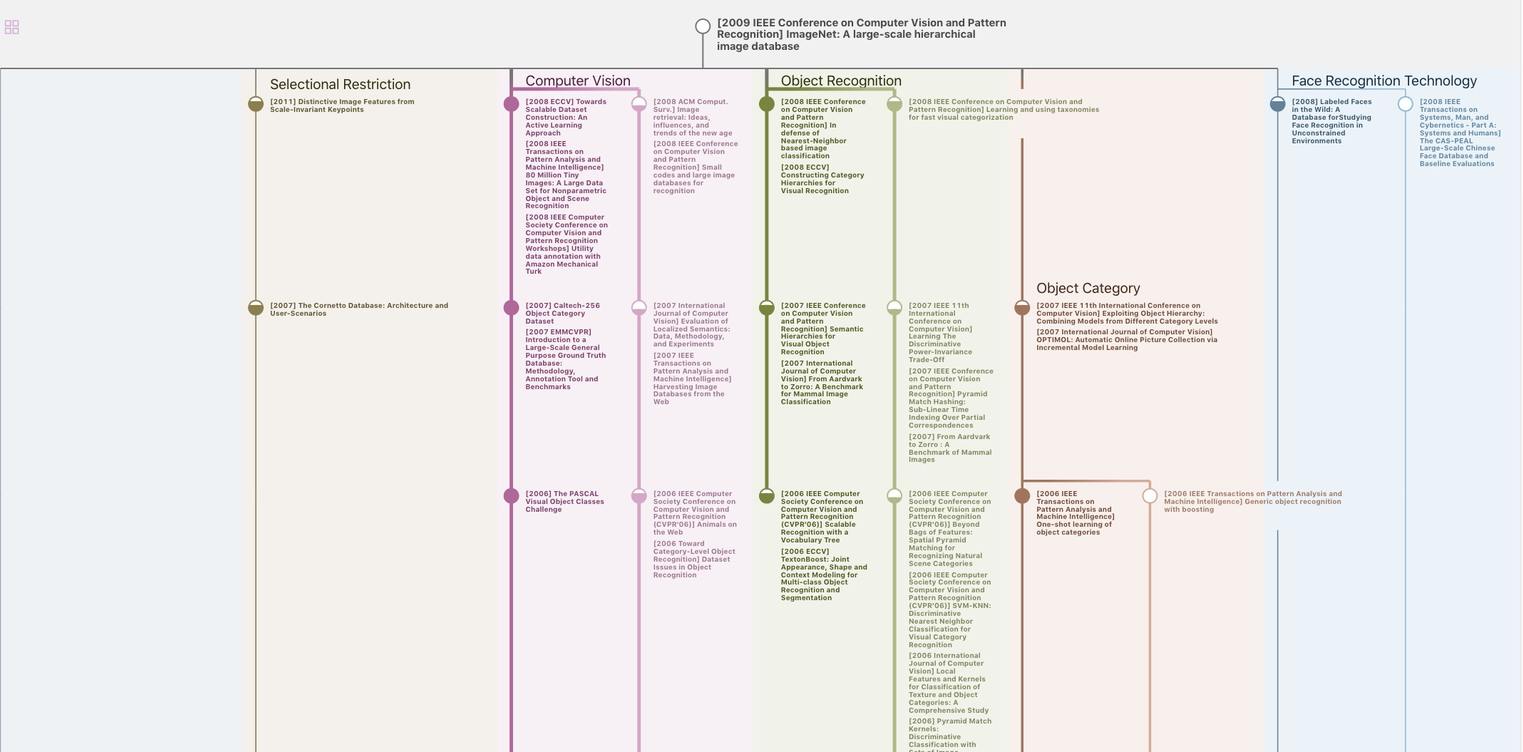
Generate MRT to find the research sequence of this paper
Chat Paper
Summary is being generated by the instructions you defined