Contextual Dynamic Pricing: Algorithms, Optimality, and Local Differential Privacy Constraints
CoRR(2024)
Abstract
We study the contextual dynamic pricing problem where a firm sells products
to T sequentially arriving consumers that behave according to an unknown
demand model. The firm aims to maximize its revenue, i.e. minimize its regret
over a clairvoyant that knows the model in advance. The demand model is a
generalized linear model (GLM), allowing for a stochastic feature vector in
ℝ^d that encodes product and consumer information. We first show that
the optimal regret upper bound is of order √(dT), up to a logarithmic
factor, improving upon existing upper bounds in the literature by a √(d)
factor. This sharper rate is materialised by two algorithms: a confidence
bound-type (supCB) algorithm and an explore-then-commit (ETC) algorithm. A key
insight of our theoretical result is an intrinsic connection between dynamic
pricing and the contextual multi-armed bandit problem with many arms based on a
careful discretization. We further study contextual dynamic pricing under the
local differential privacy (LDP) constraints. In particular, we propose a
stochastic gradient descent based ETC algorithm that achieves an optimal regret
upper bound of order d√(T)/ϵ, up to a logarithmic factor, where
ϵ>0 is the privacy parameter. The regret upper bounds with and without
LDP constraints are accompanied by newly constructed minimax lower bounds,
which further characterize the cost of privacy. Extensive numerical experiments
and a real data application on online lending are conducted to illustrate the
efficiency and practical value of the proposed algorithms in dynamic pricing.
MoreTranslated text
AI Read Science
Must-Reading Tree
Example
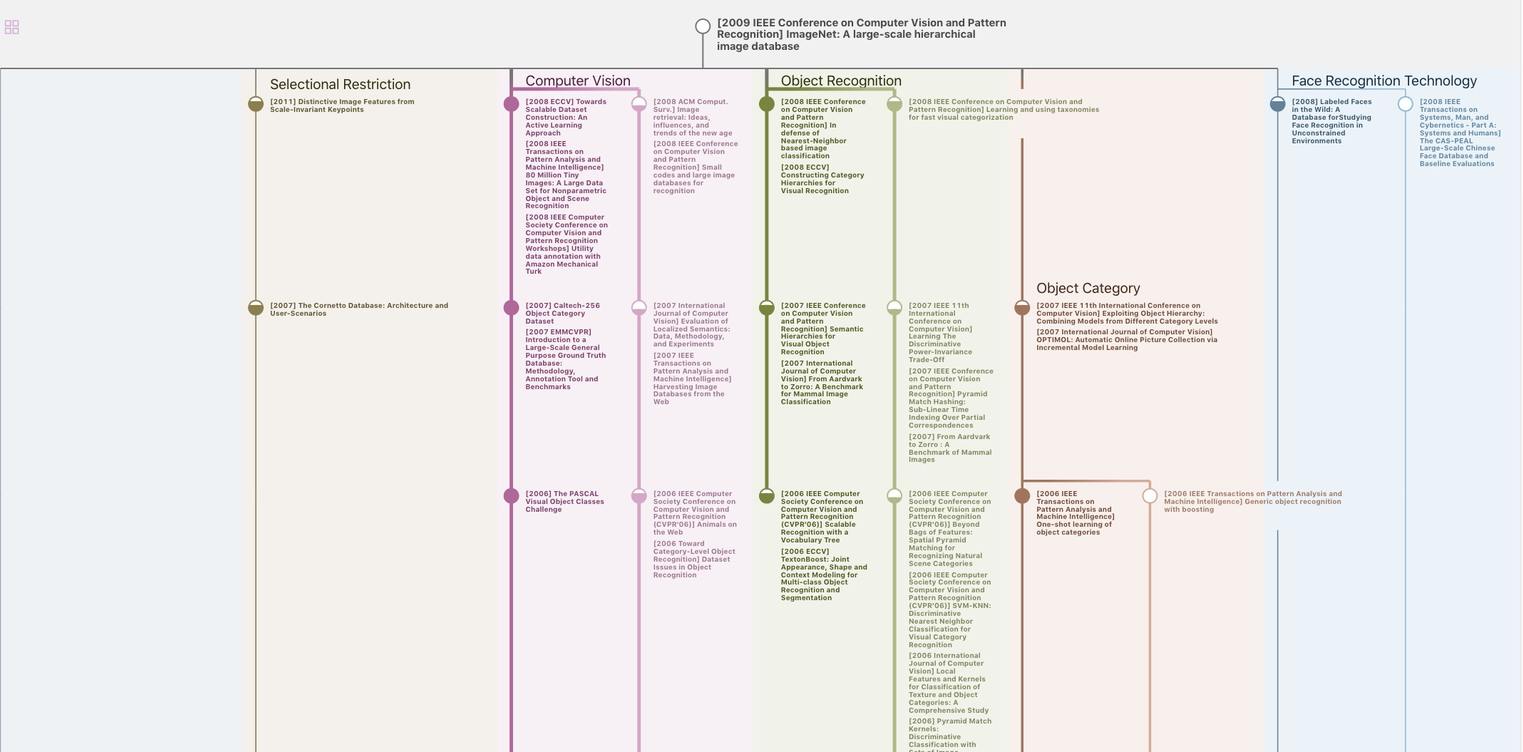
Generate MRT to find the research sequence of this paper
Chat Paper
Summary is being generated by the instructions you defined