Understanding Auditory Evoked Brain Signal via Physics-informed Embedding Network with Multi-Task Transformer
CoRR(2024)
Abstract
In the fields of brain-computer interaction and cognitive neuroscience,
effective decoding of auditory signals from task-based functional magnetic
resonance imaging (fMRI) is key to understanding how the brain processes
complex auditory information. Although existing methods have enhanced decoding
capabilities, limitations remain in information utilization and model
representation. To overcome these challenges, we propose an innovative
multi-task learning model, Physics-informed Embedding Network with Multi-Task
Transformer (PEMT-Net), which enhances decoding performance through
physics-informed embedding and deep learning techniques. PEMT-Net consists of
two principal components: feature augmentation and classification. For feature
augmentation, we propose a novel approach by creating neural embedding graphs
via node embedding, utilizing random walks to simulate the physical diffusion
of neural information. This method captures both local and non-local
information overflow and proposes a position encoding based on relative
physical coordinates. In the classification segment, we propose adaptive
embedding fusion to maximally capture linear and non-linear characteristics.
Furthermore, we propose an innovative parameter-sharing mechanism to optimize
the retention and learning of extracted features. Experiments on a specific
dataset demonstrate PEMT-Net's significant performance in multi-task auditory
signal decoding, surpassing existing methods and offering new insights into the
brain's mechanisms for processing complex auditory information.
MoreTranslated text
AI Read Science
Must-Reading Tree
Example
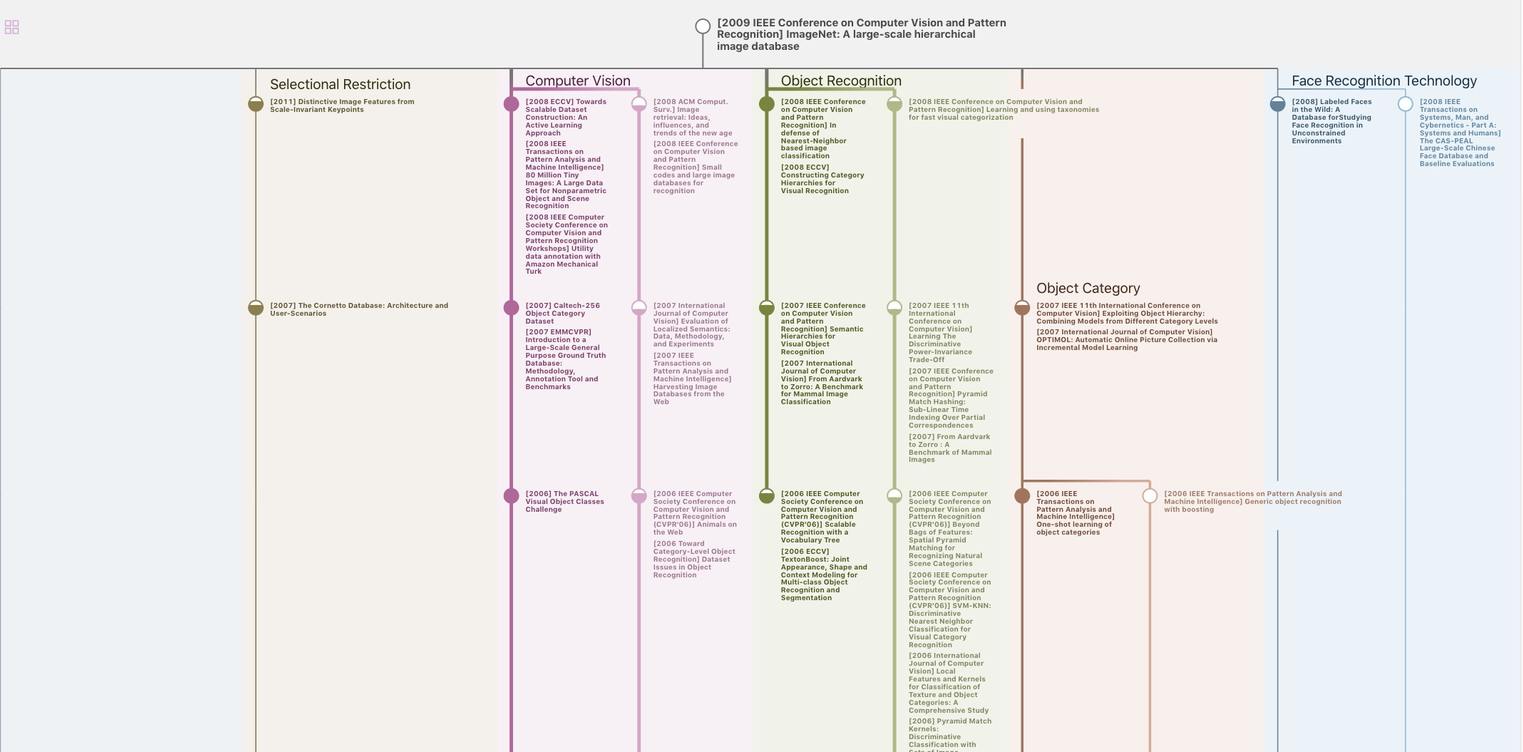
Generate MRT to find the research sequence of this paper
Chat Paper
Summary is being generated by the instructions you defined