How Does Gradient Descent Learn Features – A Local Analysis for Regularized Two-Layer Neural Networks
CoRR(2024)
Abstract
The ability of learning useful features is one of the major advantages of
neural networks. Although recent works show that neural network can operate in
a neural tangent kernel (NTK) regime that does not allow feature learning, many
works also demonstrate the potential for neural networks to go beyond NTK
regime and perform feature learning. Recently, a line of work highlighted the
feature learning capabilities of the early stages of gradient-based training.
In this paper we consider another mechanism for feature learning via gradient
descent through a local convergence analysis. We show that once the loss is
below a certain threshold, gradient descent with a carefully regularized
objective will capture ground-truth directions. Our results demonstrate that
feature learning not only happens at the initial gradient steps, but can also
occur towards the end of training.
MoreTranslated text
AI Read Science
Must-Reading Tree
Example
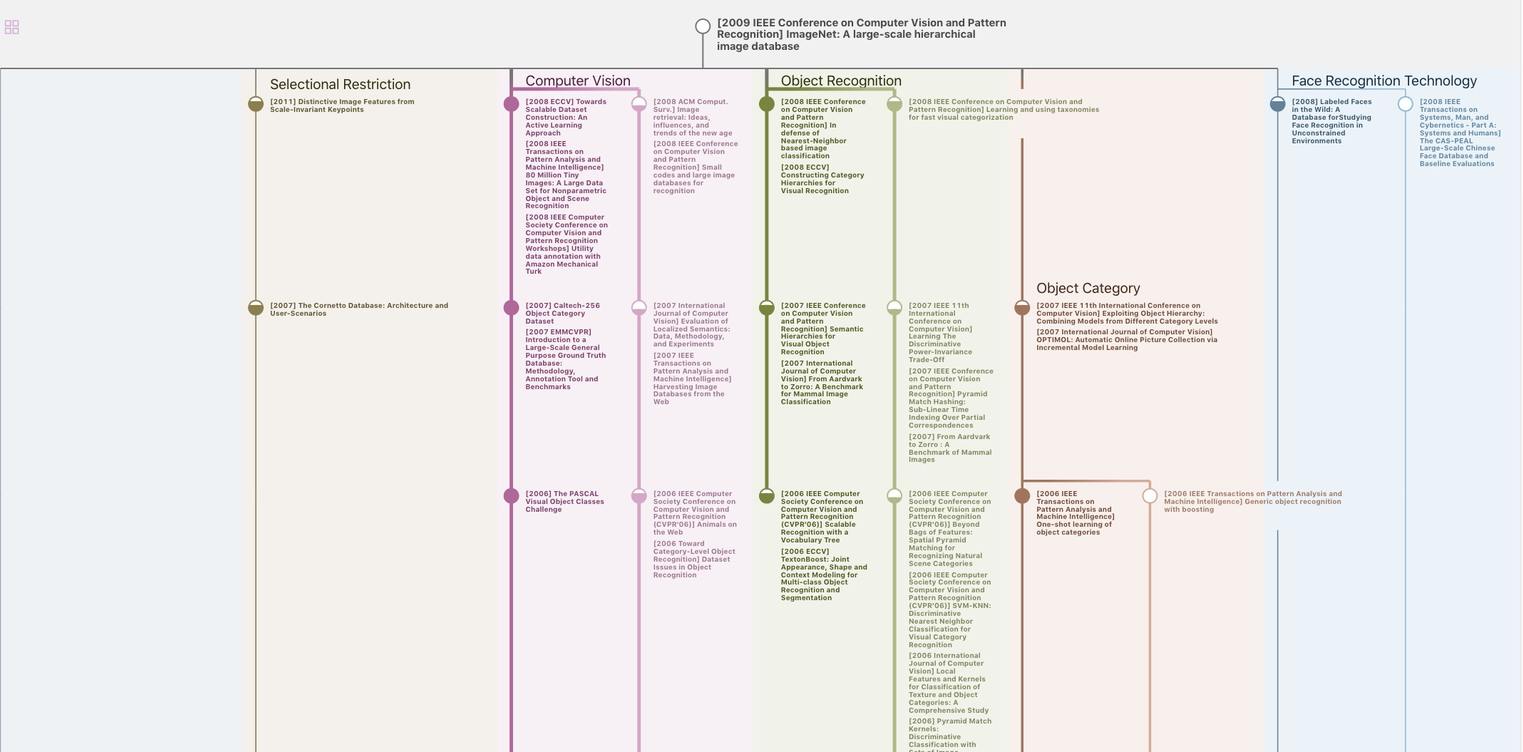
Generate MRT to find the research sequence of this paper
Chat Paper
Summary is being generated by the instructions you defined