Human Communication-Inspired Semantic-View Collaborative Network for Multispectral Remote Sensing Image Retrieval.
IEEE J. Sel. Top. Appl. Earth Obs. Remote. Sens.(2024)
Abstract
Multispectral images (MSIs) have widespread applications, and efficiently managing these extensive MSIs via remote sensing image retrieval (RSIR) is key to boosting their practical value. While current deep learning-based methods offer strong image representation learning capabilities, adapting to complex and dynamic relationships between objects and spectral information in MSIs remains challenging. This difficulty arises due to the distinct attributes of different spectral bands and the lack of consideration of interactions among spectral combinations in MSIs, which limits their retrieval performance. For this purpose, we propose a dynamic learning system inspired by human communication named the semantic view collaborative network (SVCNet), which actively promotes the interaction between spectral and semantic information. By linking multi-view learning (MVL) with graph neural networks (GNNs) to simulate the three stages of human communication understanding, communication, and collective consensus and reflection SVCNet enhances RSIR with flexibility in representation extraction. Specifically, each spectral combination is processed to extract independent representations as view-specific knowledge. In the communication phase, we devise the graph attention-based multi-round communication module (GACM), which uses GNN to perform graph-structured modeling and adaptive updating of views and semantics. Moreover, we achieve improved MSI representations by implementing novel objective functions that align learned semantics with category information, dynamically differentiating semantic similarities and disparities in MSIs, and flexibly weighting samples for enhanced adaptability in a multi-label RSIR environment. SVCNet surpasses current state-of-the-art methods in three MSI datasets for single and multi-label retrieval tasks. It effectively handles class imbalances and distinguishes challenging samples, highlighting its extensive applicability.
MoreTranslated text
Key words
Content-based image retrieval,graph neural networks,multi-view learning,multispectral image
AI Read Science
Must-Reading Tree
Example
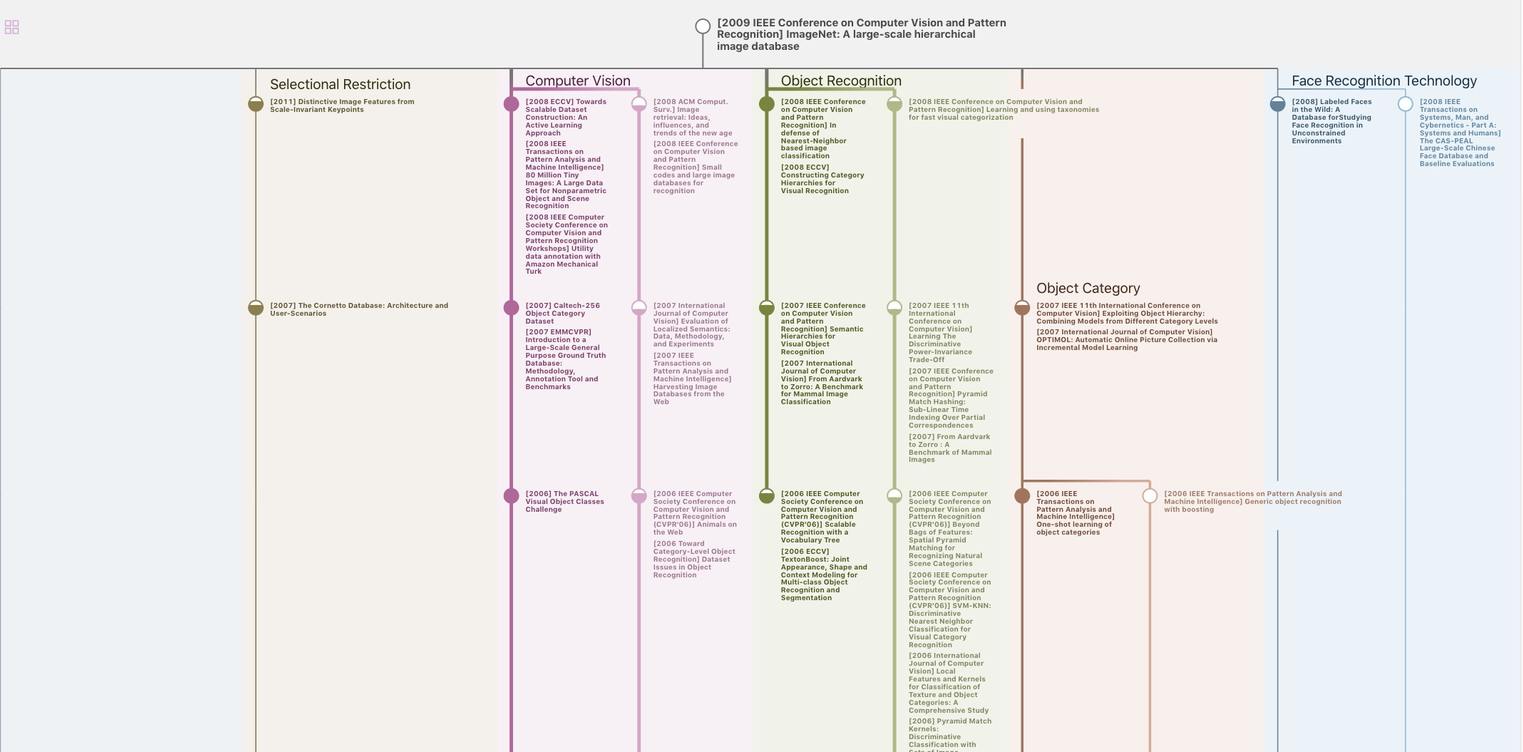
Generate MRT to find the research sequence of this paper
Chat Paper
Summary is being generated by the instructions you defined