Intelligent Bayesian regularization backpropagation neuro computing paradigm for state features estimation of underwater passive object
Frontiers in Physics(2024)
摘要
In underwater environments, the accurate estimation of state features for passive object is a critical aspect of various applications, including underwater robotics, surveillance, and environmental monitoring. This study presents an innovative neuro computing approach for instantaneous state features reckoning of passive marine object following dynamic Markov chains. This paper introduces the potential of intelligent Bayesian regularization backpropagation neuro computing (IBRBNC) for the precise estimation of state features of underwater passive object. The proposed paradigm combines the power of artificial neural network with Bayesian regularization technique to address the challenges associated with noisy and limited underwater sensor data. The IBRBNC paradigm leverages deep neural networks with a focus on backpropagation to model complex relationships in the underwater environment. Furthermore, Bayesian regularization is introduced to incorporate prior knowledge and mitigate overfitting, enhancing the model’s robustness and generalization capabilities. This dual approach results in a highly adaptive and intelligent system capable of accurately estimating the state features of passive object in real-time. To evaluate the efficacy of this intelligent computing approach, a controlled supervised maneuvering trajectory for underwater passive object is constructed. Real-time estimations of location, velocity, and turn rate for dynamic target are scrutinized across five distinct scenarios by varying the Gaussian observed noise’s standard deviation, aiming to minimize mean square errors (MSEs) between real and estimated values. The effectiveness of the proposed IBRBNC paradigm is demonstrated through extensive simulations and experimental trials. Results showcase its superiority over traditional nonlinear filtering methods like interacting multiple model extended Kalman filter (IMMEKF) and interacting multiple model unscented Kalman filter (IMMUKF), especially in the presence of noise, incomplete measurements and sparse data.
更多查看译文
关键词
neuro computing,Markov chain,state features,sensor data,robustness,Bayesian regularization
AI 理解论文
溯源树
样例
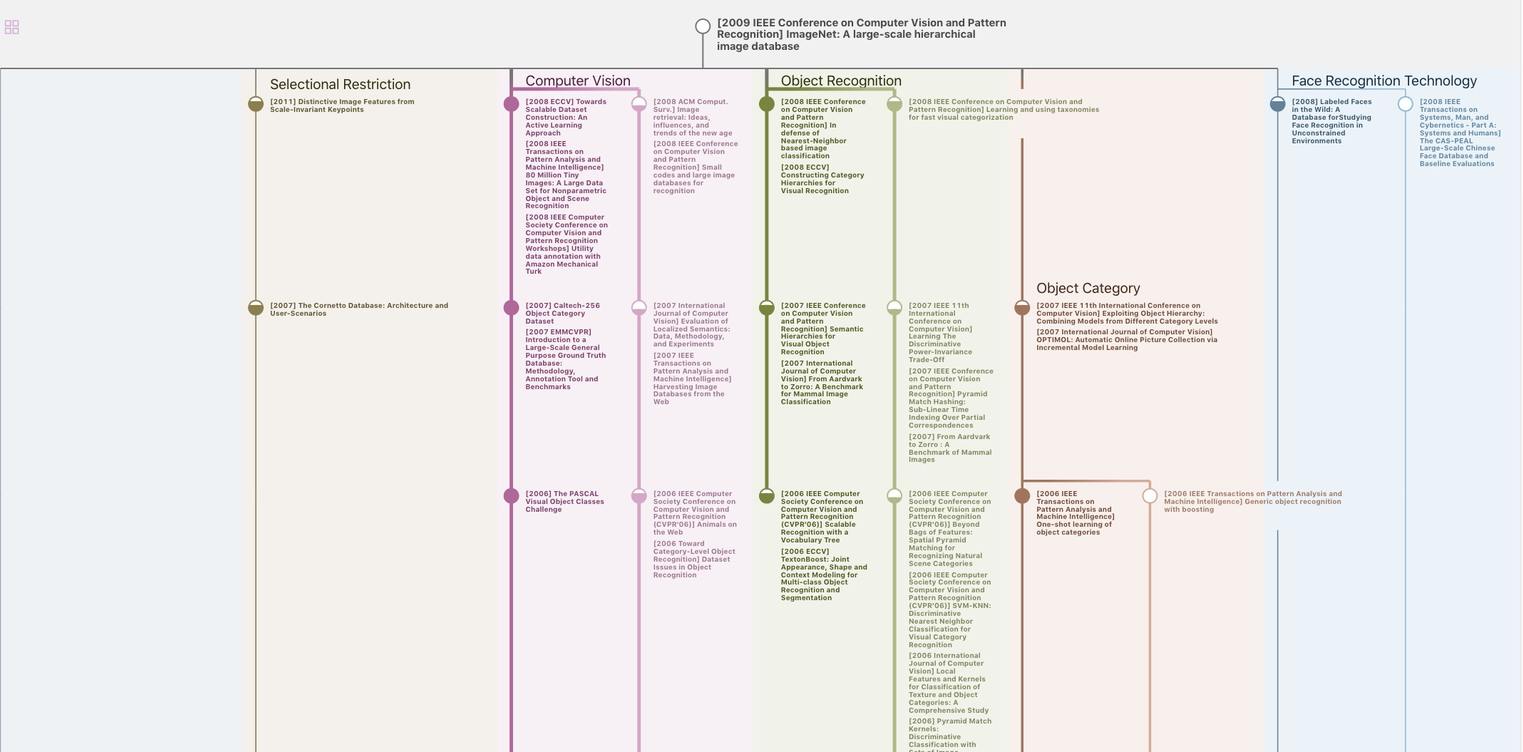
生成溯源树,研究论文发展脉络
Chat Paper
正在生成论文摘要