Disrupting how we recruit to cancer clinical trials.
Journal of Clinical Oncology(2024)
Abstract
11072 Background: Traditional methods to identify eligible patients for cancer clinical trials rely heavily on manual review, a time-consuming bottle neck limiting access to novel treatments, particularly for underserved populations. This study presents an automated informatics platform designed to simplify and streamline patient identification and trial matching. Methods: Our platform leverages three tools: 1. Parser:Extracts and categorizes trial data based on cancer type, stage, and receptor status from the National Cancer Institute's ClinicalTrials.gov database, storing it in a structured JSON format; 2. Screener: Utilizes Epic Clarity electronic health record data to identify upcoming patients approaching treatment decision points, times when clinical trials are more relevant. Patients are classified as "newly diagnosed" or "potentially progressed" based on progress notes and imaging reports. Eligibility criteria, including stage and receptor status, are assessed using regular expressions; 3. Matcher: Pairs eligible patients with relevant trials based on compatible cancer stage and receptor status. One Cancer Center (CC) is prospectively using the platform to identify patients. The 2nd CC is refining the code using retrospective data. Accuracy was measured comparing automated to manual review by experienced trained abstractors. Results: Implementation across two cancer centers yielded: 1. Reduced manual review burden: Between June 2023 – Jan 2024, of 674 breast cancer patients seen at CC #1, 371 were new patients (N=371) and thus, at a treatment decision point; 3 did not undergo imaging. Of the 300 established patients undergoing imaging studies, the algorithm identified 50/300 (17%) scans as “positive,” and 61 (20%) with mixed disease progression/ regression, requiring manual review and trial matching. 189 scans were read as “negative”: 96% True Negatives; 4% were False Negatives. Automated screening reduced manual review from 300 to 111 patients, significantly alleviating workload on healthcare personnel. 2. Variable staging accuracy: While receptor status classification proved effective, stage accuracy varied between centers, highlighting a need for further refinement in this area. 3. Accurate receptor status classification: The system achieved high accuracy in demonstrating reliable assessment of this vital factor. Conclusions: This automated informatics platform demonstrates significant potential to streamline case identification for cancer clinical trials and thus, foster broader access to novel therapies. Its adaptability makes it suitable for implementation across diverse healthcare settings, empowering wider participation in advancements in cancer care. Ongoing efforts to refine the staging module are underway. Clinical trial information: NCT05146297 . [Table: see text]
MoreTranslated text
AI Read Science
Must-Reading Tree
Example
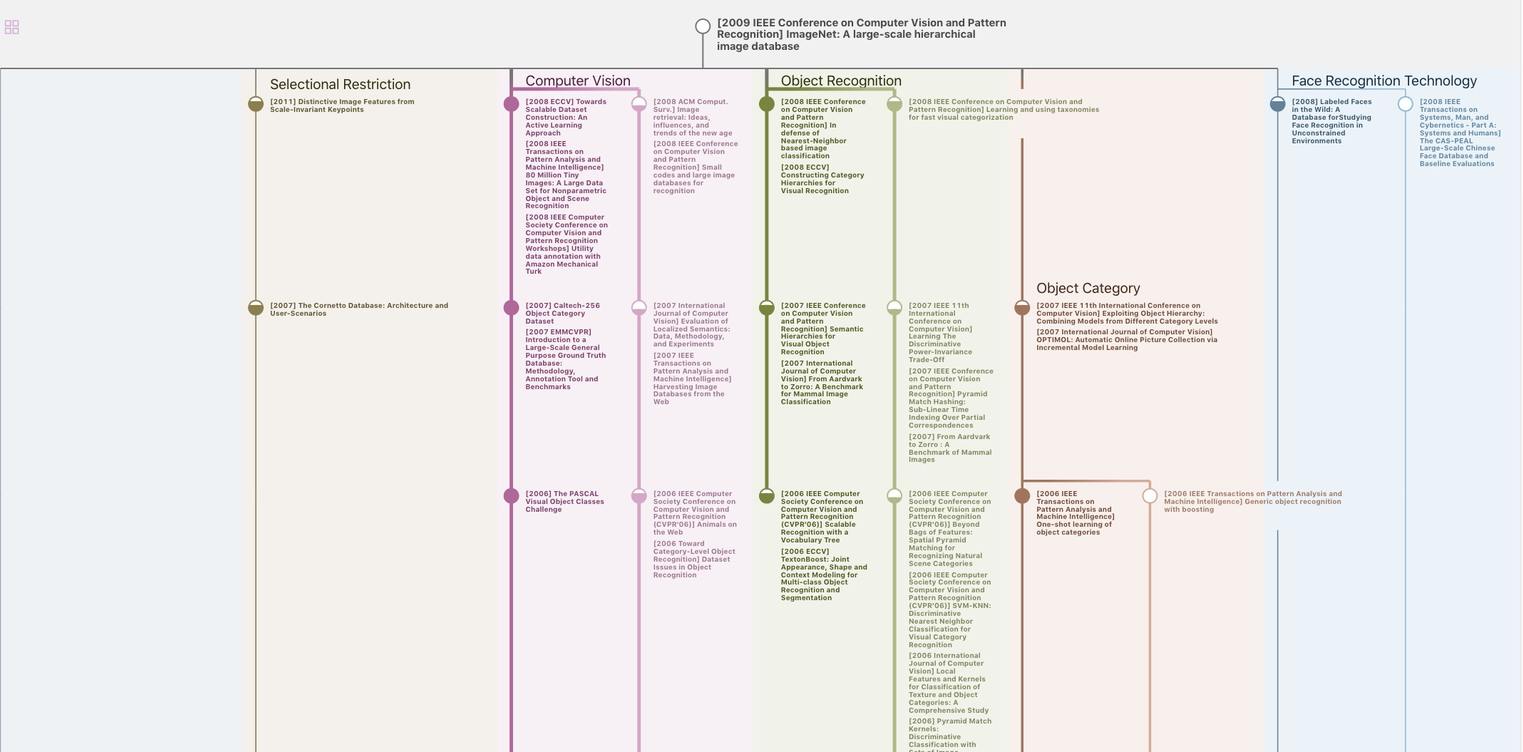
Generate MRT to find the research sequence of this paper
Chat Paper
Summary is being generated by the instructions you defined