MSO-GP: 3-D segmentation of large and complex conjoined tree structures
Methods(2024)
摘要
Robust segmentation of large and complex conjoined tree structures in 3-D is a major challenge in computer vision. This is particularly true in computational biology, where we often encounter large data structures in size, but few in number, which poses a hard problem for learning algorithms. We show that merging multiscale opening with geodesic path propagation, can shed new light on this classic machine vision challenge, while circumventing the learning issue by developing an unsupervised visual geometry approach (digital topology/morphometry). The novelty of the proposed MSO-GP method comes from the geodesic path propagation being guided by a skeletonization of the conjoined structure that helps to achieve robust segmentation results in a particularly challenging task in this area, that of artery-vein separation from non-contrast pulmonary computed tomography angiograms. This is an important first step in measuring vascular geometry to then diagnose pulmonary diseases and to develop image-based phenotypes. We first present proof-of-concept results on synthetic data, and then verify the performance on pig lung and human lung data with less segmentation time and user intervention needs than those of the competing methods.
更多查看译文
关键词
multiscale opening,conjoined trees,digital topology,computational biology,3D segmentation,visual geometry,morphometry
AI 理解论文
溯源树
样例
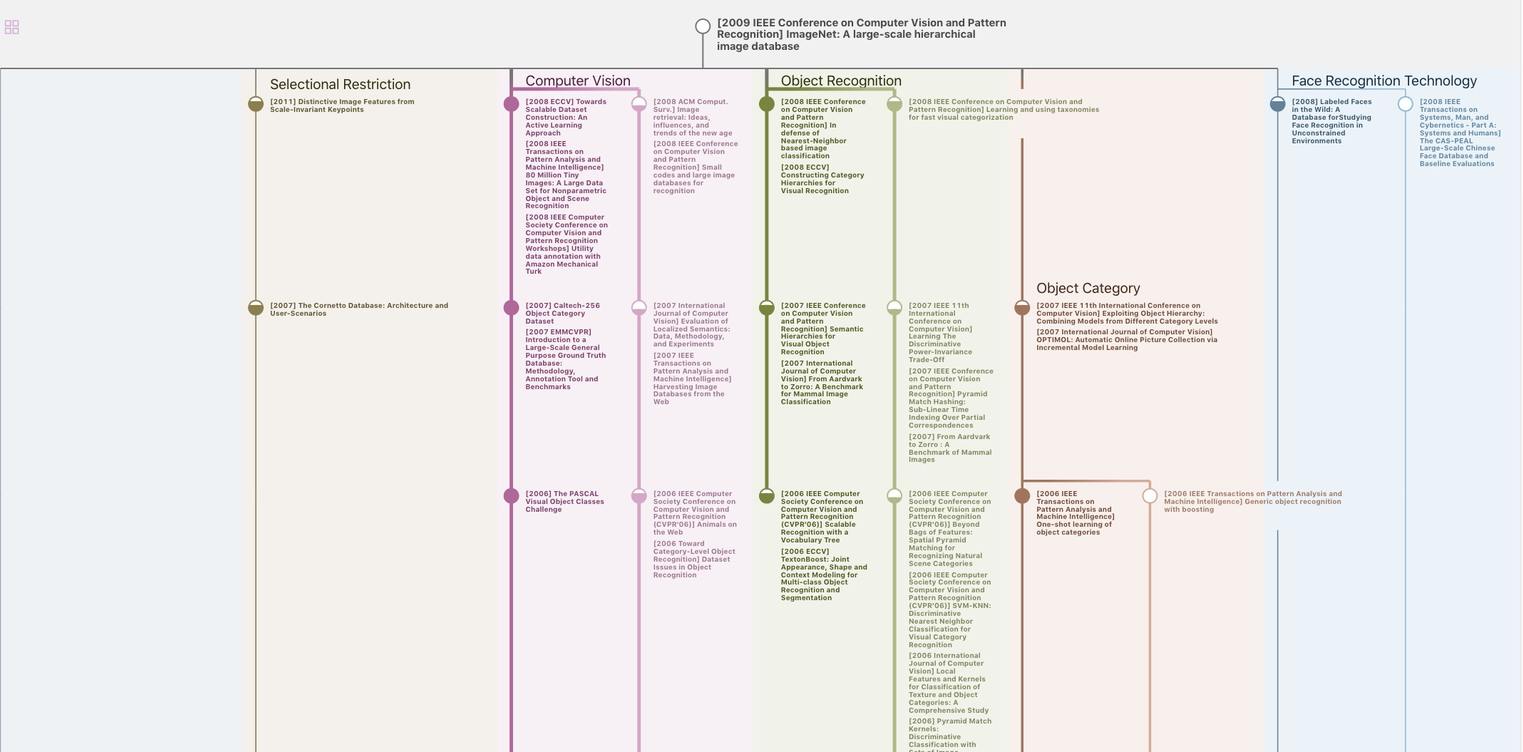
生成溯源树,研究论文发展脉络
Chat Paper
正在生成论文摘要