WEA-DINO: An Improved DINO With Word Embedding Alignment for Remote Scene Zero-Shot Object Detection
IEEE GEOSCIENCE AND REMOTE SENSING LETTERS(2024)
Abstract
Remote sensing scene zero-shot object detection (ZSD) aims to detect and recognize both seen and unseen categories of landscape elements with the guidance of the word embeddings. In this task, two primary challenges are identified. First, there exists considerable variability within categories of landscape elements, causing a misalignment between visual features and word embeddings, particularly noticeable for unseen categories. Second, the existing detection models struggle to provide accurate localization predictions, greatly impacting overall performance. To address these two issues, we propose word embedding alignment-DINO (WEA-DINO). Based on the original DINO structure, our WEA-DINO-Head is specifically designed to align the hidden features of "matching queries" with word embedding features, effectively addressing the misalignment issue between visual features and word embeddings. Furthermore, aligning the hidden features of "denoising queries" with word embedding features enables the translation of localization capabilities from known categories to previously unseen ones. Through extensive experimentation on the DIOR benchmark dataset, our method demonstrates state-of-the-art (SOTA) performance. The code is available at https://github.com/cv516Buaa/WEA-DINO.
MoreTranslated text
Key words
Feature alignment,remote sensing,word embedding guidance,zero-shot object detection (ZSD)
AI Read Science
Must-Reading Tree
Example
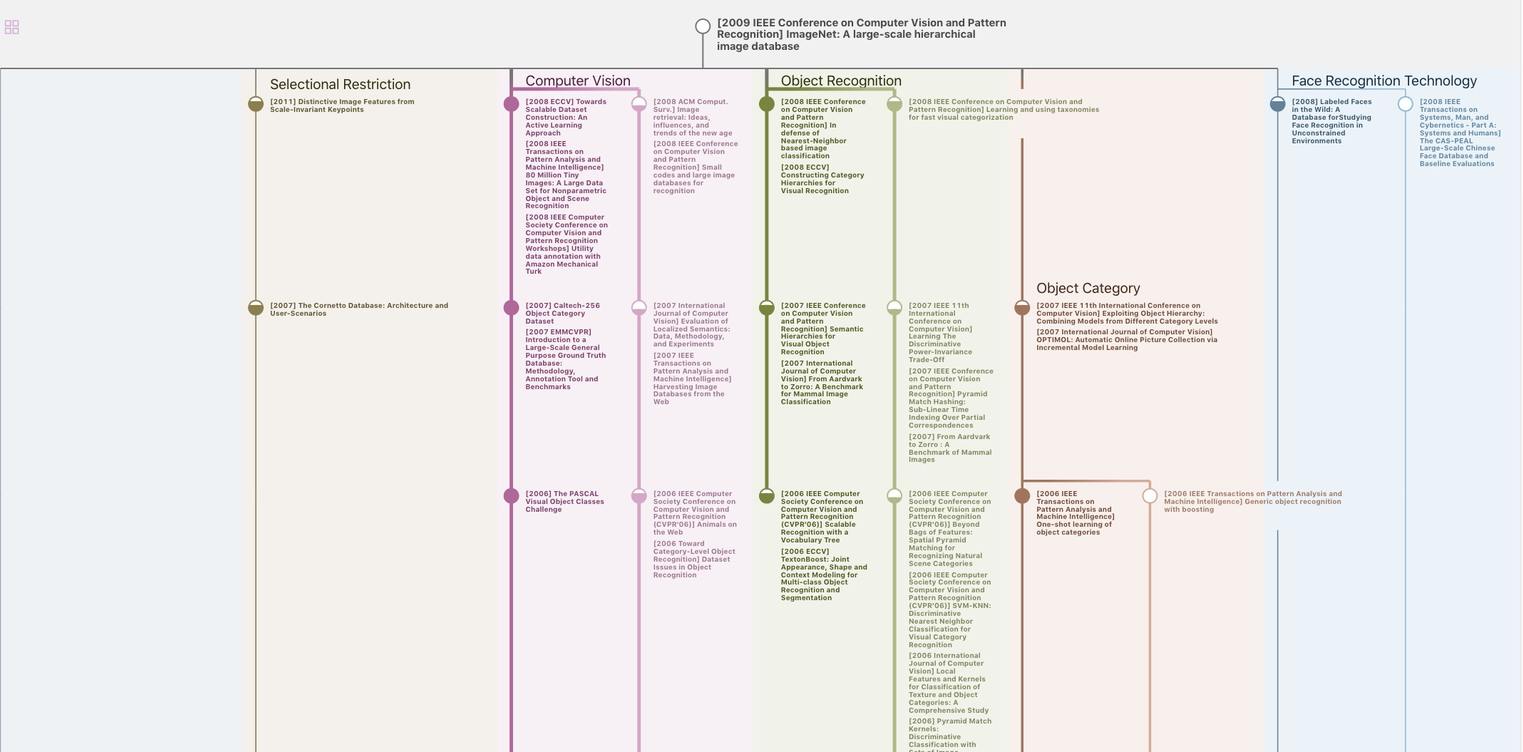
Generate MRT to find the research sequence of this paper
Chat Paper
Summary is being generated by the instructions you defined