Sparsity-Agnostic Linear Bandits with Adaptive Adversaries
CoRR(2024)
Abstract
We study stochastic linear bandits where, in each round, the learner receives
a set of actions (i.e., feature vectors), from which it chooses an element and
obtains a stochastic reward. The expected reward is a fixed but unknown linear
function of the chosen action. We study sparse regret bounds, that depend on
the number S of non-zero coefficients in the linear reward function. Previous
works focused on the case where S is known, or the action sets satisfy
additional assumptions. In this work, we obtain the first sparse regret bounds
that hold when S is unknown and the action sets are adversarially generated.
Our techniques combine online to confidence set conversions with a novel
randomized model selection approach over a hierarchy of nested confidence sets.
When S is known, our analysis recovers state-of-the-art bounds for
adversarial action sets. We also show that a variant of our approach, using
Exp3 to dynamically select the confidence sets, can be used to improve the
empirical performance of stochastic linear bandits while enjoying a regret
bound with optimal dependence on the time horizon.
MoreTranslated text
AI Read Science
Must-Reading Tree
Example
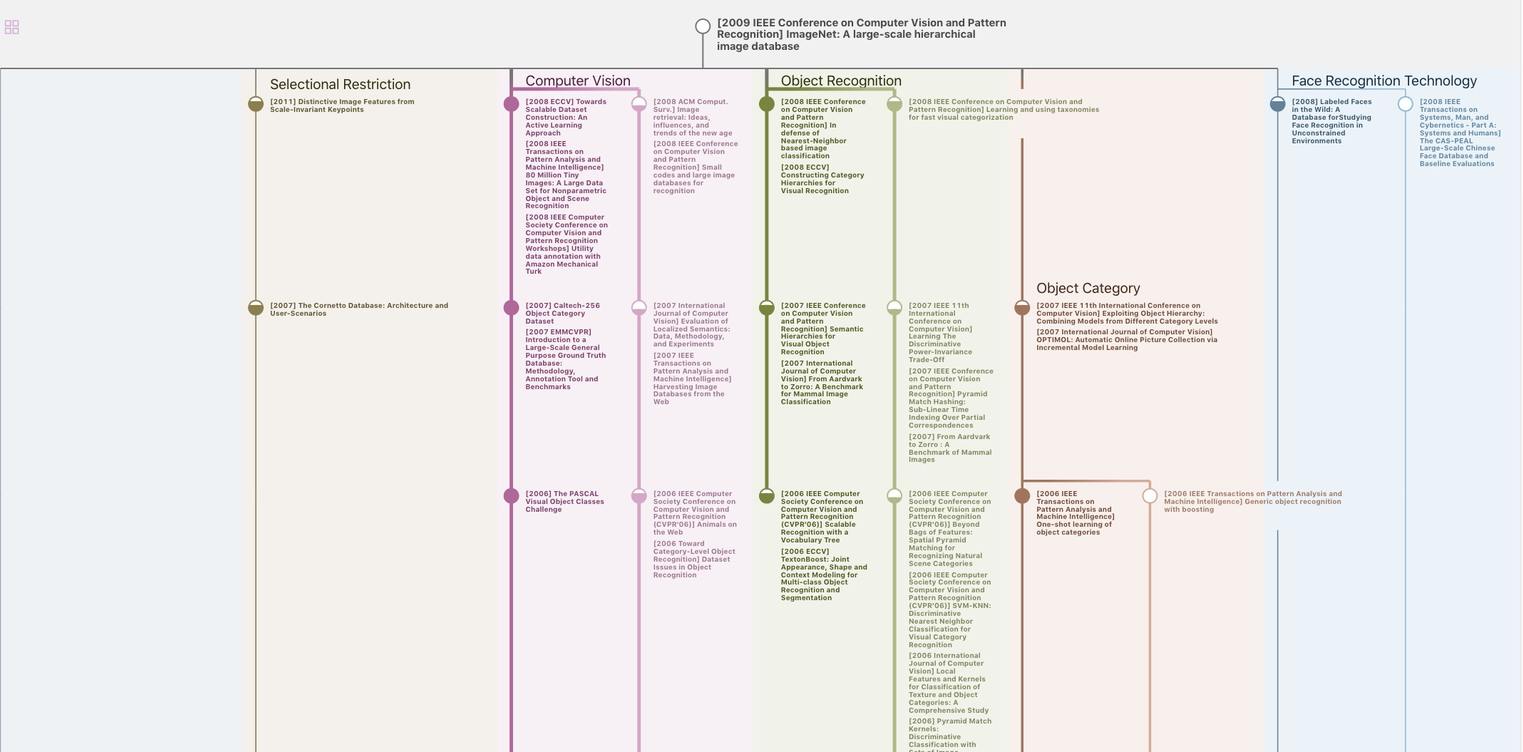
Generate MRT to find the research sequence of this paper
Chat Paper
Summary is being generated by the instructions you defined