Cohort Squeeze: Beyond a Single Communication Round per Cohort in Cross-Device Federated Learning
CoRR(2024)
摘要
Virtually all federated learning (FL) methods, including FedAvg, operate in
the following manner: i) an orchestrating server sends the current model
parameters to a cohort of clients selected via certain rule, ii) these clients
then independently perform a local training procedure (e.g., via SGD or Adam)
using their own training data, and iii) the resulting models are shipped to the
server for aggregation. This process is repeated until a model of suitable
quality is found. A notable feature of these methods is that each cohort is
involved in a single communication round with the server only. In this work we
challenge this algorithmic design primitive and investigate whether it is
possible to “squeeze more juice" out of each cohort than what is possible in a
single communication round. Surprisingly, we find that this is indeed the case,
and our approach leads to up to 74
needed to train a FL model in the cross-device setting. Our method is based on
a novel variant of the stochastic proximal point method (SPPM-AS) which
supports a large collection of client sampling procedures some of which lead to
further gains when compared to classical client selection approaches.
更多查看译文
AI 理解论文
溯源树
样例
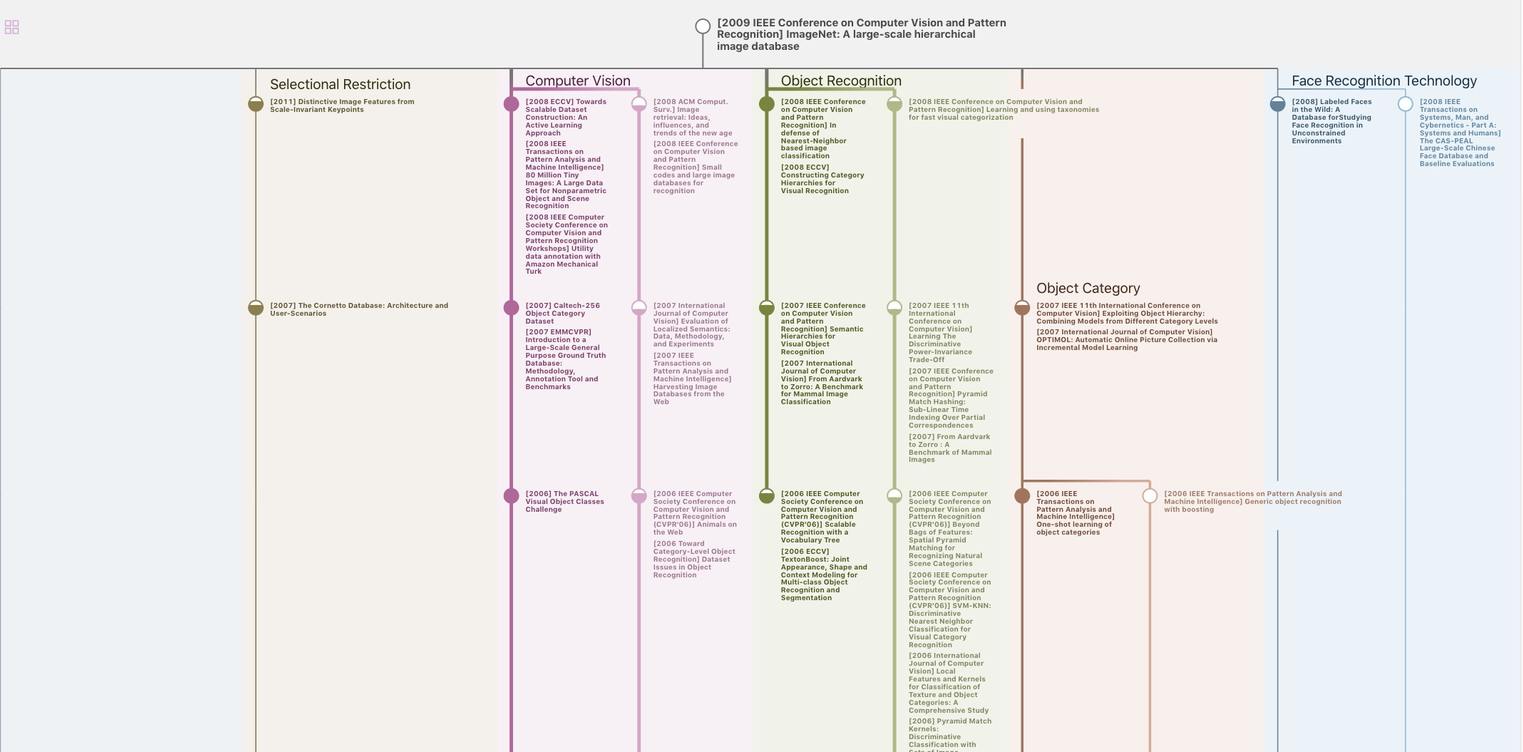
生成溯源树,研究论文发展脉络
Chat Paper
正在生成论文摘要