Boosting Sortition via Proportional Representation
arxiv(2024)
摘要
Sortition is based on the idea of choosing randomly selected representatives
for decision making. The main properties that make sortition particularly
appealing are fairness – all the citizens can be selected with the same
probability – and proportional representation – a randomly selected panel
probably reflects the composition of the whole population. When a population
lies on a representation metric, we formally define proportional representation
by using a notion called the core. A panel is in the core if no group of
individuals is underrepresented proportional to its size. While uniform
selection is fair, it does not always return panels that are in the core. Thus,
we ask if we can design a selection algorithm that satisfies fairness and ex
post core simultaneously. We answer this question affirmatively and present an
efficient selection algorithm that is fair and provides a constant-factor
approximation to the optimal ex post core. Moreover, we show that uniformly
random selection satisfies a constant-factor approximation to the optimal ex
ante core. We complement our theoretical results by conducting experiments with
real data.
更多查看译文
AI 理解论文
溯源树
样例
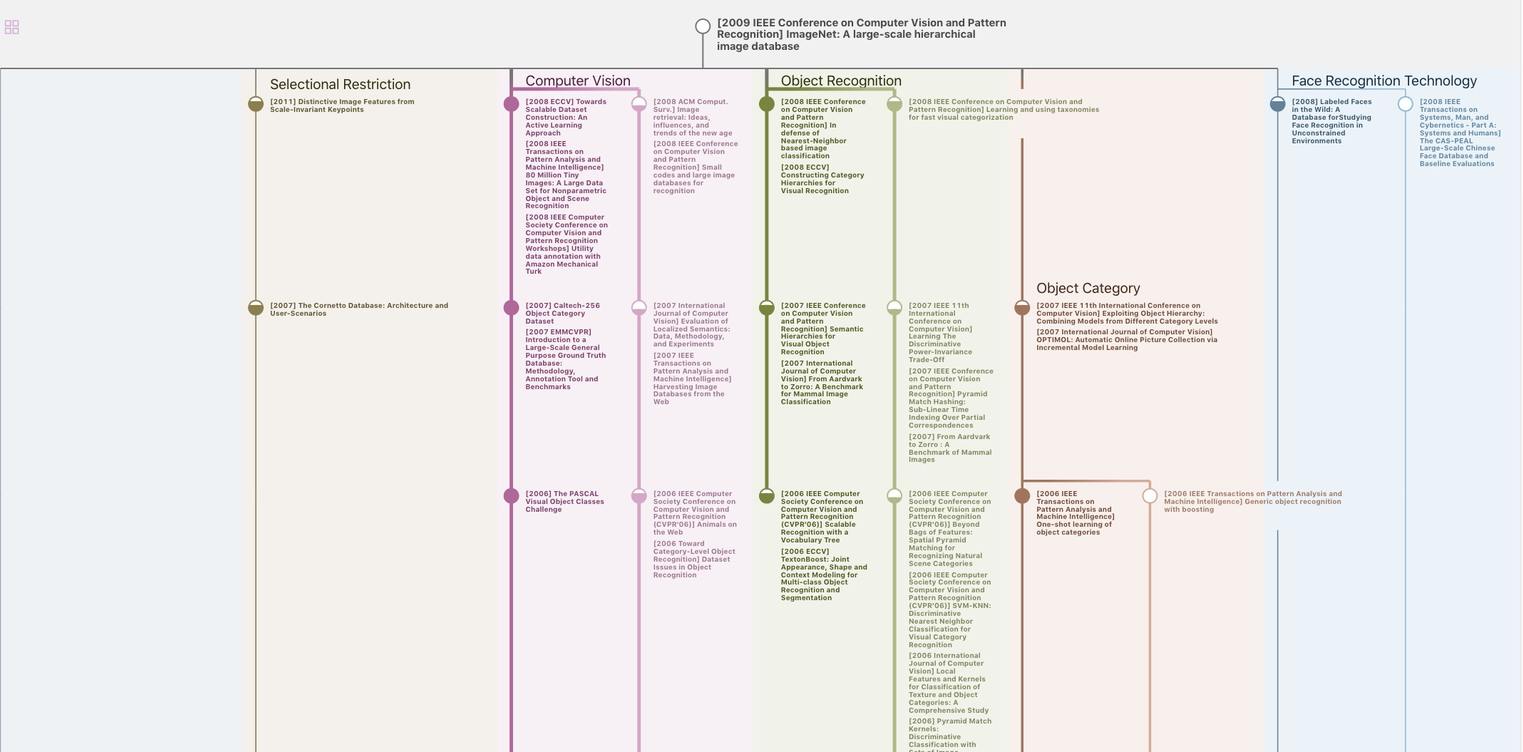
生成溯源树,研究论文发展脉络
Chat Paper
正在生成论文摘要