A transfer learning strategy for tensile strength prediction in austenitic stainless steel across temperatures
Scripta Materialia(2024)
摘要
Austenitic stainless steel plays a pivotal role in the nuclear industry with exceptional mechanical properties and corrosion resistance, wherein high-temperature tensile strength (∼290 °C) is a critical factor in ensuring its performance and reliability. This study focuses on the predictive modeling of tensile strength in cast austenitic stainless steel at room and elevated temperatures by developing a transfer learning strategy. The application of a gradient-boosting decision tree algorithm, enhanced by key features derived from room-temperature analyses and augmented with partial high-temperature data, results in significantly increased accuracy. The effective selection of features underscores the stability of critical variables across different temperatures, affirming the efficacy of the proposed transfer learning strategy. The work sheds light on material selection and design for high-temperature applications, and lays the groundwork for future exploration into predictive modeling of material properties in extreme conditions.
更多查看译文
关键词
Austenitic stainless steel,Tensile strength,Machine learning,Transfer learning
AI 理解论文
溯源树
样例
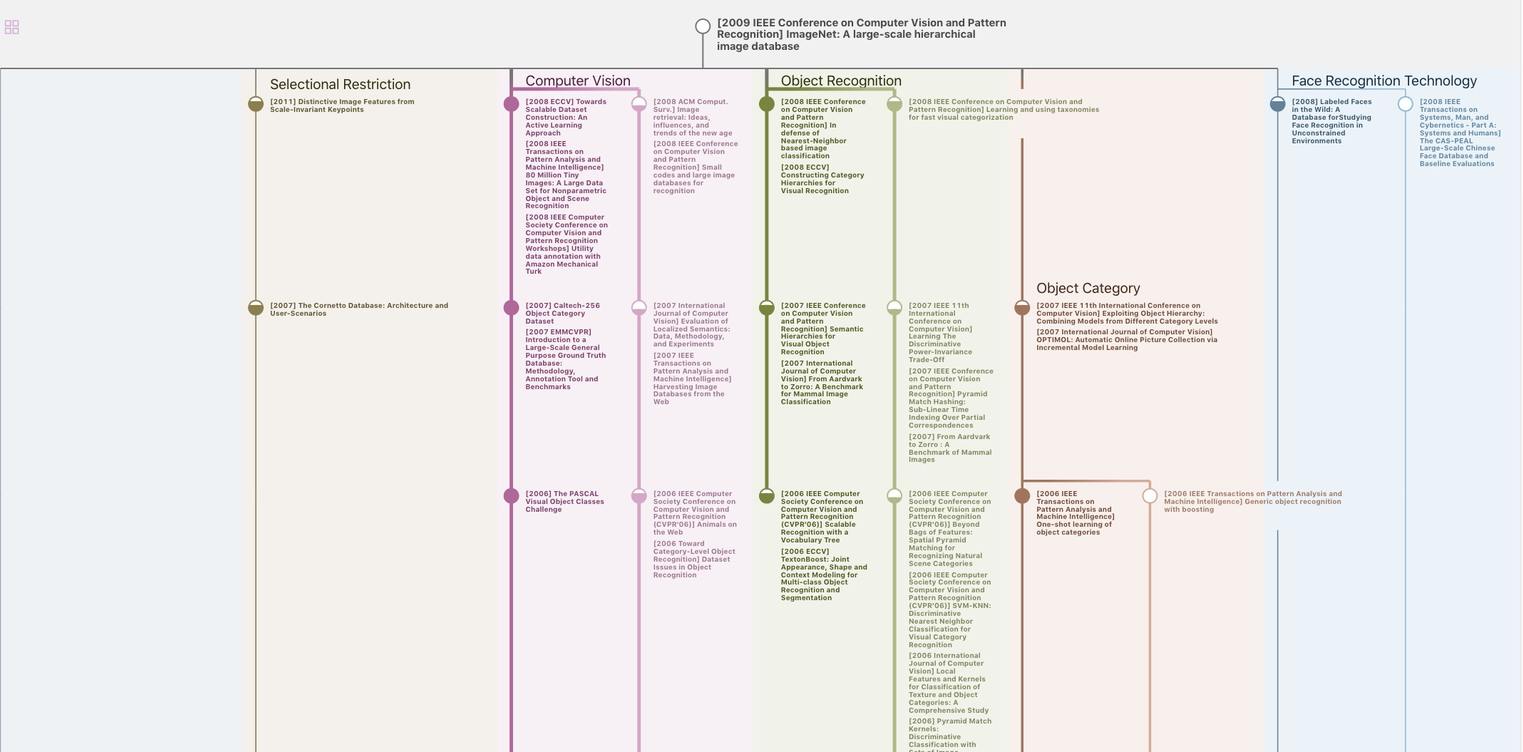
生成溯源树,研究论文发展脉络
Chat Paper
正在生成论文摘要