MF2ShrT: Multimodal Feature Fusion Using Shared Layered Transformer for Face Anti-spoofing
ACM TRANSACTIONS ON MULTIMEDIA COMPUTING COMMUNICATIONS AND APPLICATIONS(2024)
摘要
In recent times, Face Anti-spoofing (FAS) has gained significant attention in both academic and industrial domains. Although various convolutional neural network (CNN)-based solutions have emerged, multimodal approaches incorporating RGB, depth, and information retrieval (IR) have exhibited better performance than unimodal classifiers. The increasing veracity of modern presentation attack instruments results in a persistent need to enhance the performance of such models. Recently, self-attention-based vision transformers (ViT) have become a popular choice in this field. Their fundamental aspects formultimodal FAS have not been thoroughly explored yet. Therefore, we propose a novel framework for FAS called MF(2)ShrT, which is based on a pretrained vision transformer. The proposed framework uses overlap patches and parameter sharing in the ViT network, allowing it to utilize multiple modalities in a computationally efficient manner. Furthermore, to effectively fuse intermediate features from different encoders of each ViT, we explore a T-encoder-based hybrid feature block enabling the system to identify correlations and dependencies across different modalities. MF(2)ShrT outperforms conventional vision transformers and achieves state-of-the-art performance on benchmarks CASIA-SURF and WMCA, demonstrating the efficiency of transformer-based models for presentation attack detection PAD).
更多查看译文
关键词
Face anti-spoofing,presentation attack detection,multimodal,vision transformer
AI 理解论文
溯源树
样例
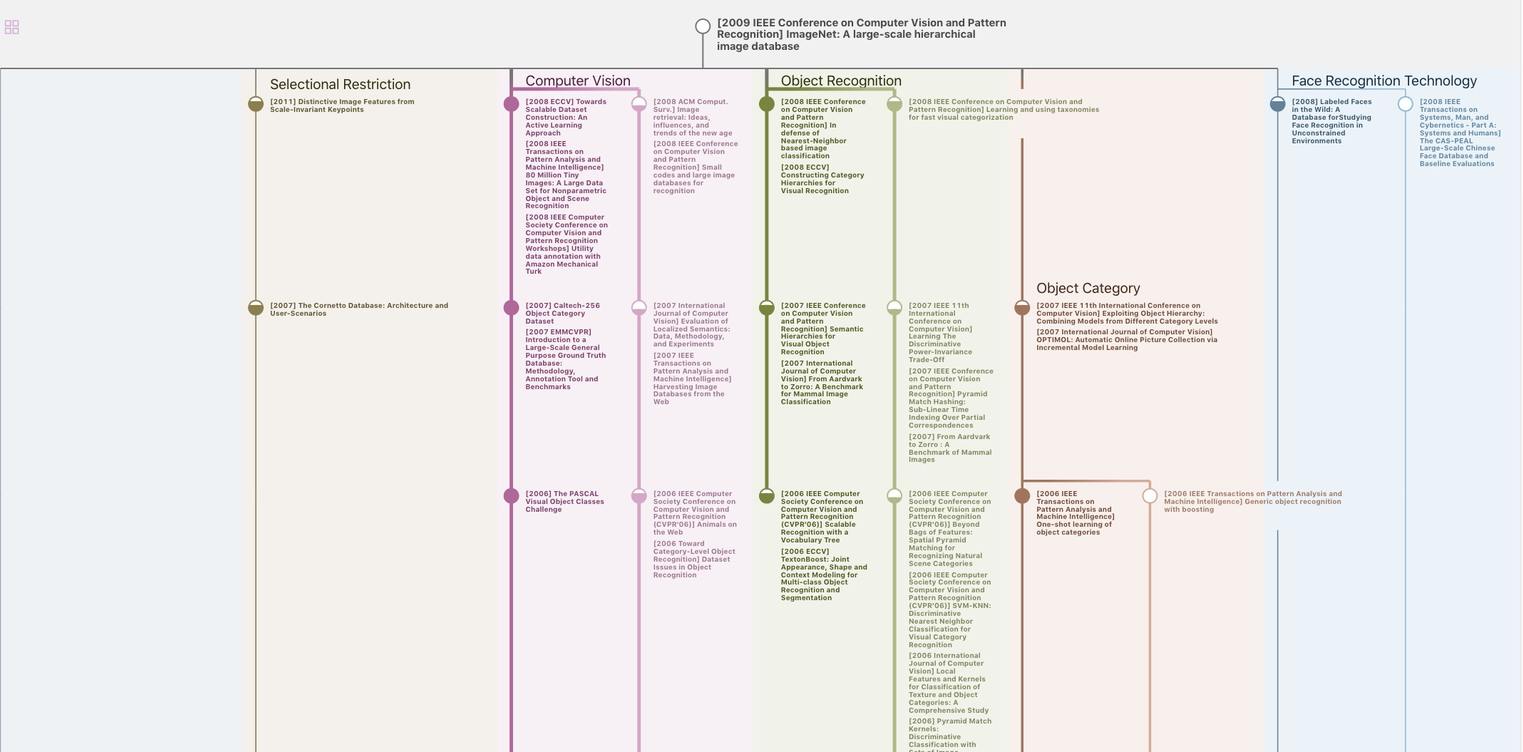
生成溯源树,研究论文发展脉络
Chat Paper
正在生成论文摘要