Research on image classification based on residual group multi-scale enhanced attention network
Computers and Electrical Engineering(2024)
Abstract
To address the challenges associated with insufficient feature extraction and gradient degradation encountered when dealing with deepening network structures in image classification tasks, this paper presents a ResGMEANet(Residual Group Multi-scale Enhanced Attention Network). The model introduces a multi-scale attention enhancement module. This design draws inspiration from the original model's capability to independently capture feature correlations in channels and spaces. By implementing shuffle operations and feature transformations within the group, our method expands the receptive field through the utilization of multiple convolution kernels. Additionally, we incorporate an improved tensor synthesis attention, building upon the traditional convolution attention, to derive attention feature maps after feature enhancement. Evaluation on the CIFAR-10 and CIFAR-100 datasets shows that ResGMEANet outperforms both the original backbone model and several existing mainstream methods in classification accuracy. This work aims to provide a new perspective for the future by combining residual neural networks with different attention mechanisms.
MoreTranslated text
Key words
Multi-scale convolution,Feature enhancement,Image classification,Algorithm optimization
AI Read Science
Must-Reading Tree
Example
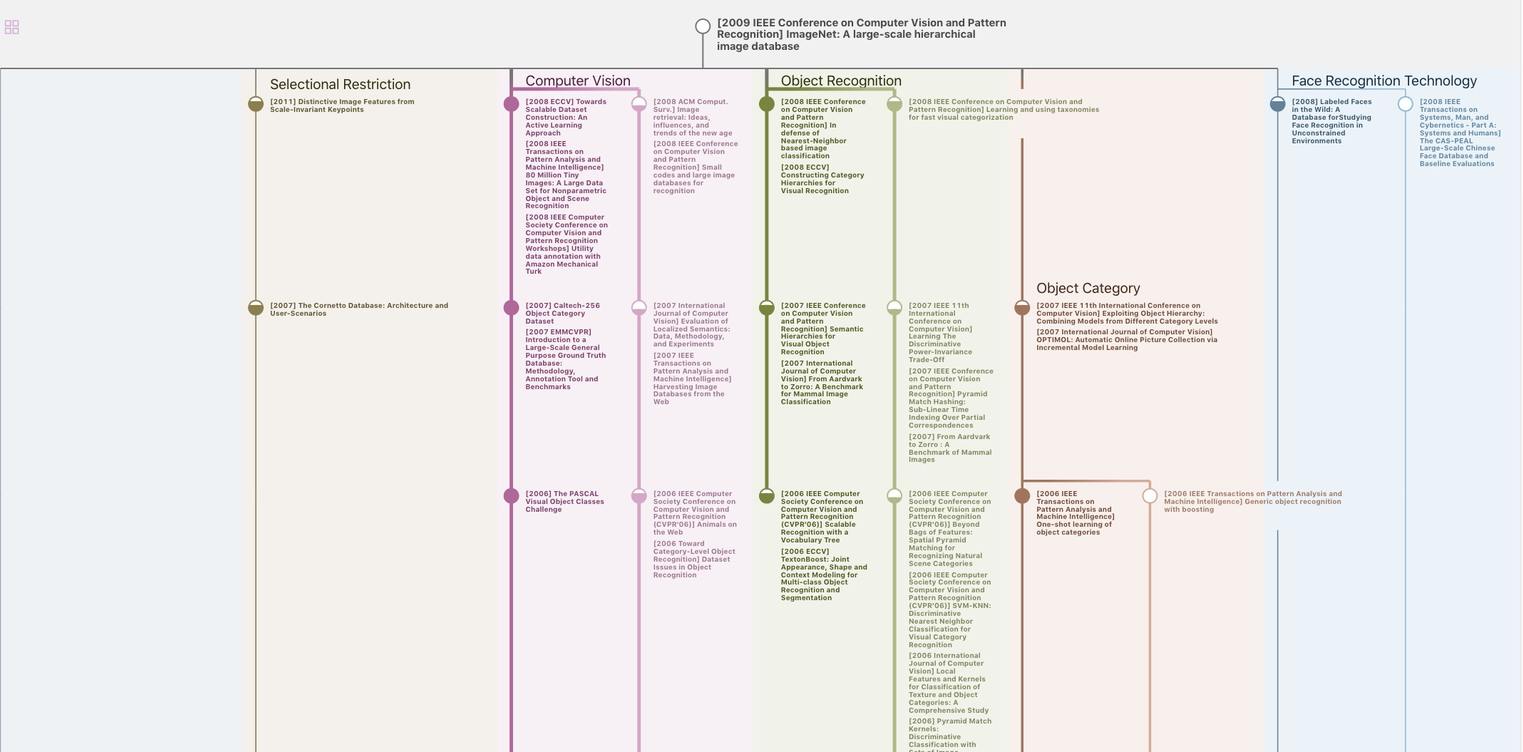
Generate MRT to find the research sequence of this paper
Chat Paper
Summary is being generated by the instructions you defined