Effect of identical twins on deep speaker embeddings based forensic voice comparison
International Journal of Speech Technology(2024)
Abstract
Deep learning has gained widespread adoption in forensic voice comparison in recent years. It is mainly used to learn speaker representations, known as embedding features or vectors. In this work, the effect of identical twins on two state-of-the-art deep speaker embedding methods was investigated with special focus on metrics of forensic voice comparison. The speaker verification performance has been assessed using the likelihood-ratio framework by likelihood ratio cost and equal error rate. The AVTD twin speech dataset was applied. The results show a significant reduction in speaker verification performance when twin samples are present. Neither the adaptation of LR score calculation to twin samples, nor fine-tuning the pre-trained speaker embedding models seemed to be able to leverage this limitation. It was found that the recognition of same or different speakers was possible even in the case of identical twins but the performance dropped greatly. The lowest EER of the best performing model was 3.4
MoreTranslated text
Key words
Forensic voice comparison,Speaker verification,X-vectors,ECAPA-TDNN,Twins,Likelihood-ratio framework
AI Read Science
Must-Reading Tree
Example
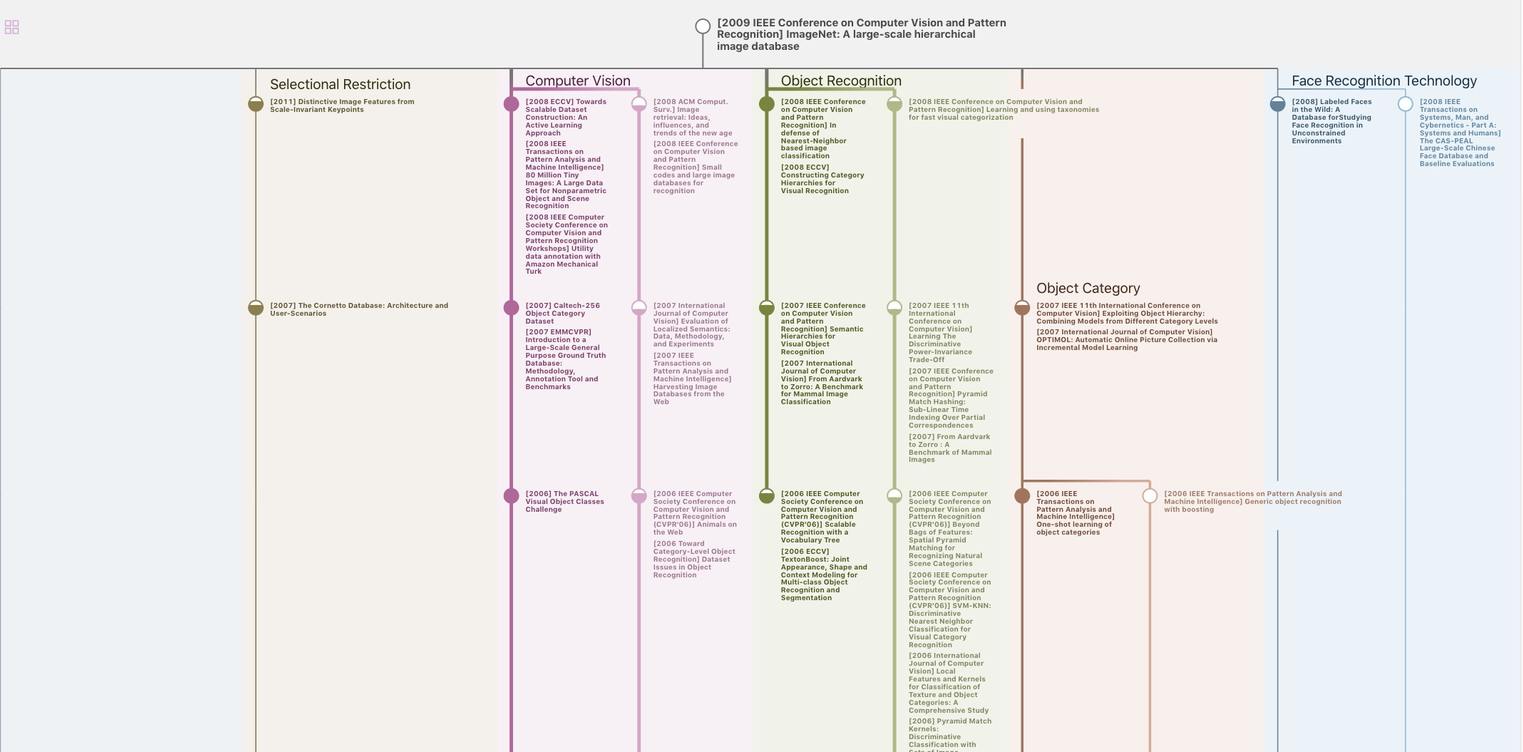
Generate MRT to find the research sequence of this paper
Chat Paper
Summary is being generated by the instructions you defined