Alterations of Brain Structural and Functional Connectivity Networks Topology and Decoupling in Pediatric Myelin Oligodendrocyte Glycoprotein Antibody-Associated Disease
Multiple sclerosis and related disorders(2024)
Abstract
ObjectiveTo investigate the alteration in structural and functional connectivity networks (SCN and FCN) as well as their coupling in pediatric myelin oligodendrocyte glycoprotein antibody-associated disease (MOGAD), and determine if these properties could serve as potential biomarkers for the disease.Materials and MethodsIn total of 32 children with MOGAD and 30 age- and sex-matched healthy controls (HC) were employed to construct the SCN and FCN, respectively. The graph-theoretical analyses of the global properties, node properties of the 90 brain nodes, and the structural-functional connectivity (SC-FC) coupling of the two networks were performed. The graph-theoretical properties that exhibited significant differences were analyzed using partial correlation analysis in conjunction with the clinical scales, including the expanded disability status scale (EDSS), modified Rankin scale (mRS), and pediatric cerebral performance category (PCPC) of the MOGAD group. Subsequently, a machine learning model was developed to discriminate between MOGAD and the HC group, aiming to explore the potential of these properties as biomarkers.ResultsThe SCN of the MOGAD group exhibited aberrant global properties, including an increased characteristic path length (Lp) and a decreased global efficiency (Eg), along with reduced nodal properties such as degree centrality (Dc), nodal efficiency (Ne), and local efficiency in multiple nodes. The FCN of the MOGAD group only exhibited decreased Dc, Ne, and betweenness centrality in two nodes of nodal properties. Besides, MOGAD showed a significant decrease in SC-FC coupling compared to the HC group. The analysis of partial correlation revealed significant correlations between several properties and the scales of EDSS and mRS in the MOGAD group. The machine learning method was used to extract six features and establish the model, achieving a classification accuracy of 82.3% for MOGAD.ConclusionsPediatric MOGAD showed a more pronounced impairment in the SCN along with decoupling of SC-FC. Both partial correlation analysis and discriminant modeling suggest that alterations in brain network properties have the potential as biomarkers for assessing brain damage in MOGAD.
MoreTranslated text
Key words
Myelin oligodendrocyte glycoprotein antibody-associated disease,Structural connectivity networks,Functional connectivity networks,Graph theory,Machine learning
AI Read Science
Must-Reading Tree
Example
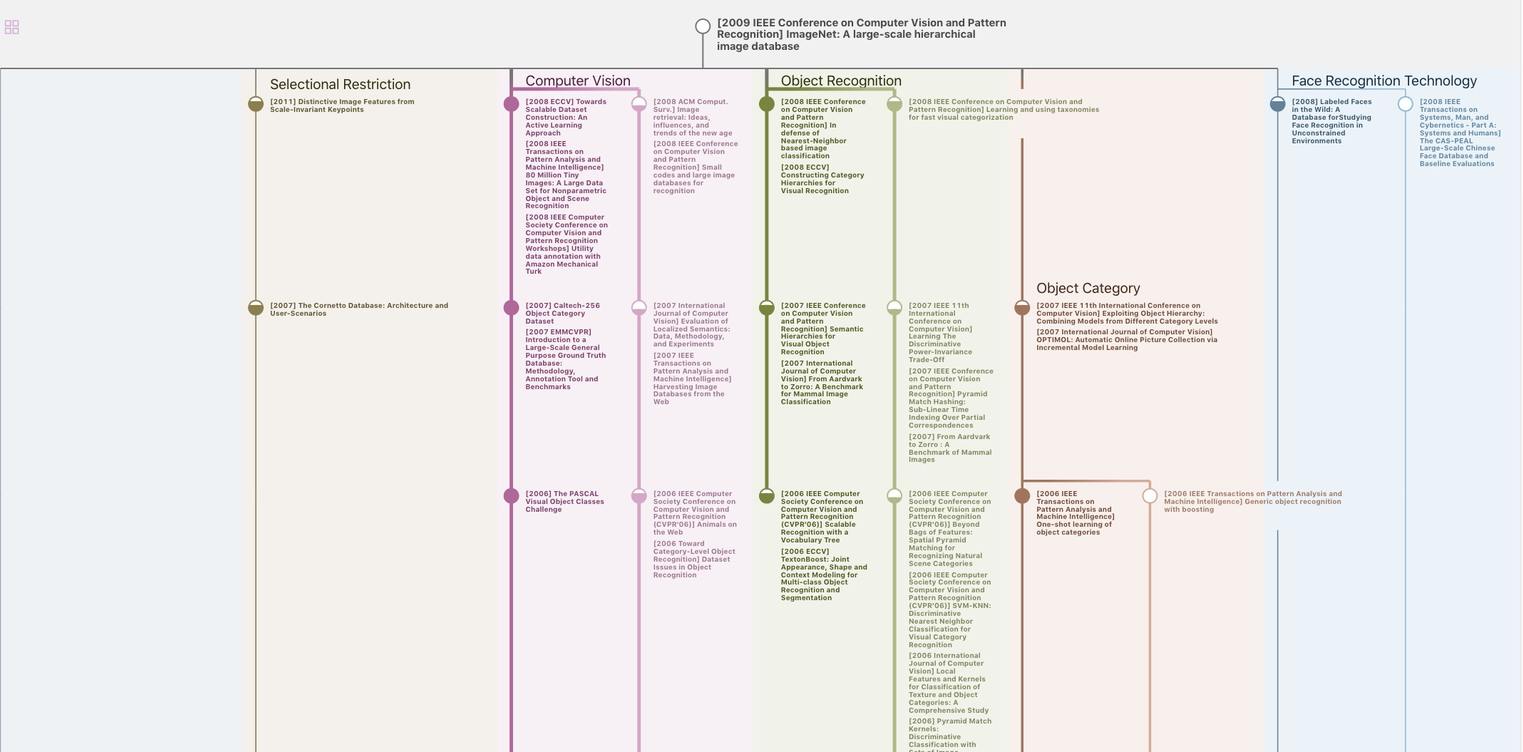
Generate MRT to find the research sequence of this paper
Chat Paper
Summary is being generated by the instructions you defined