Rethinking Open-World Semi-Supervised Learning: Distribution Mismatch and Inductive Inference
CoRR(2024)
Abstract
Open-world semi-supervised learning (OWSSL) extends conventional
semi-supervised learning to open-world scenarios by taking account of novel
categories in unlabeled datasets. Despite the recent advancements in OWSSL, the
success often relies on the assumptions that 1) labeled and unlabeled datasets
share the same balanced class prior distribution, which does not generally hold
in real-world applications, and 2) unlabeled training datasets are utilized for
evaluation, where such transductive inference might not adequately address
challenges in the wild. In this paper, we aim to generalize OWSSL by addressing
them. Our work suggests that practical OWSSL may require different training
settings, evaluation methods, and learning strategies compared to those
prevalent in the existing literature.
MoreTranslated text
AI Read Science
Must-Reading Tree
Example
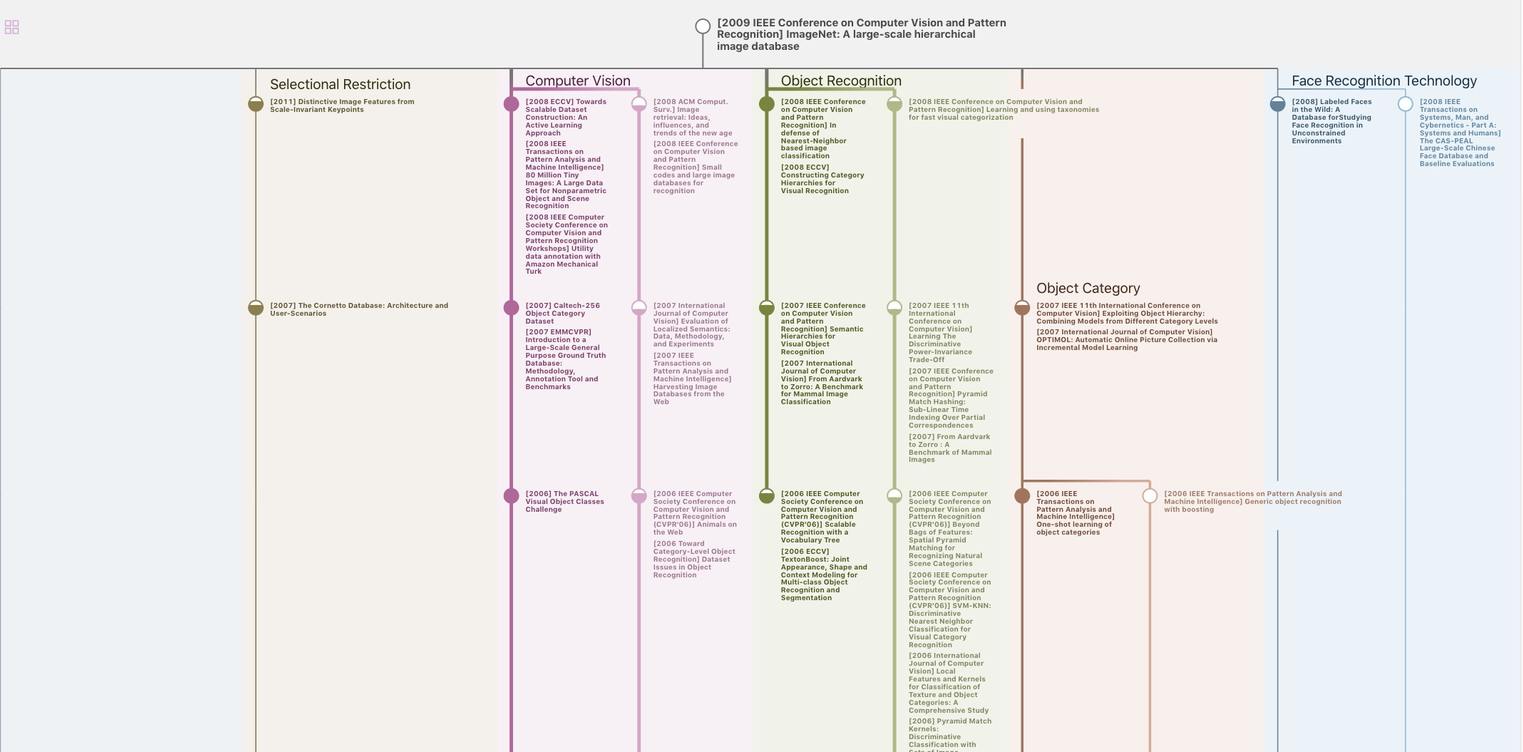
Generate MRT to find the research sequence of this paper
Chat Paper
Summary is being generated by the instructions you defined