Robust Planning with LLM-Modulo Framework: Case Study in Travel Planning
CoRR(2024)
摘要
As the applicability of Large Language Models (LLMs) extends beyond
traditional text processing tasks, there is a burgeoning interest in their
potential to excel in planning and reasoning assignments, realms traditionally
reserved for System 2 cognitive competencies. Despite their perceived
versatility, the research community is still unraveling effective strategies to
harness these models in such complex domains. The recent discourse introduced
by the paper on LLM Modulo marks a significant stride, proposing a conceptual
framework that enhances the integration of LLMs into diverse planning and
reasoning activities. This workshop paper delves into the practical application
of this framework within the domain of travel planning, presenting a specific
instance of its implementation. We are using the Travel Planning benchmark by
the OSU NLP group, a benchmark for evaluating the performance of LLMs in
producing valid itineraries based on user queries presented in natural
language. While popular methods of enhancing the reasoning abilities of LLMs
such as Chain of Thought, ReAct, and Reflexion achieve a meager 0
0
framework for TravelPlanning domain provides a remarkable improvement,
enhancing baseline performances by 4.6x for GPT4-Turbo and even more for older
models like GPT3.5-Turbo from 0
useful roles of LLMs in the planning pipeline, as suggested in LLM-Modulo,
which can be reliably operationalized such as extraction of useful critics and
reformulator for critics.
更多查看译文
AI 理解论文
溯源树
样例
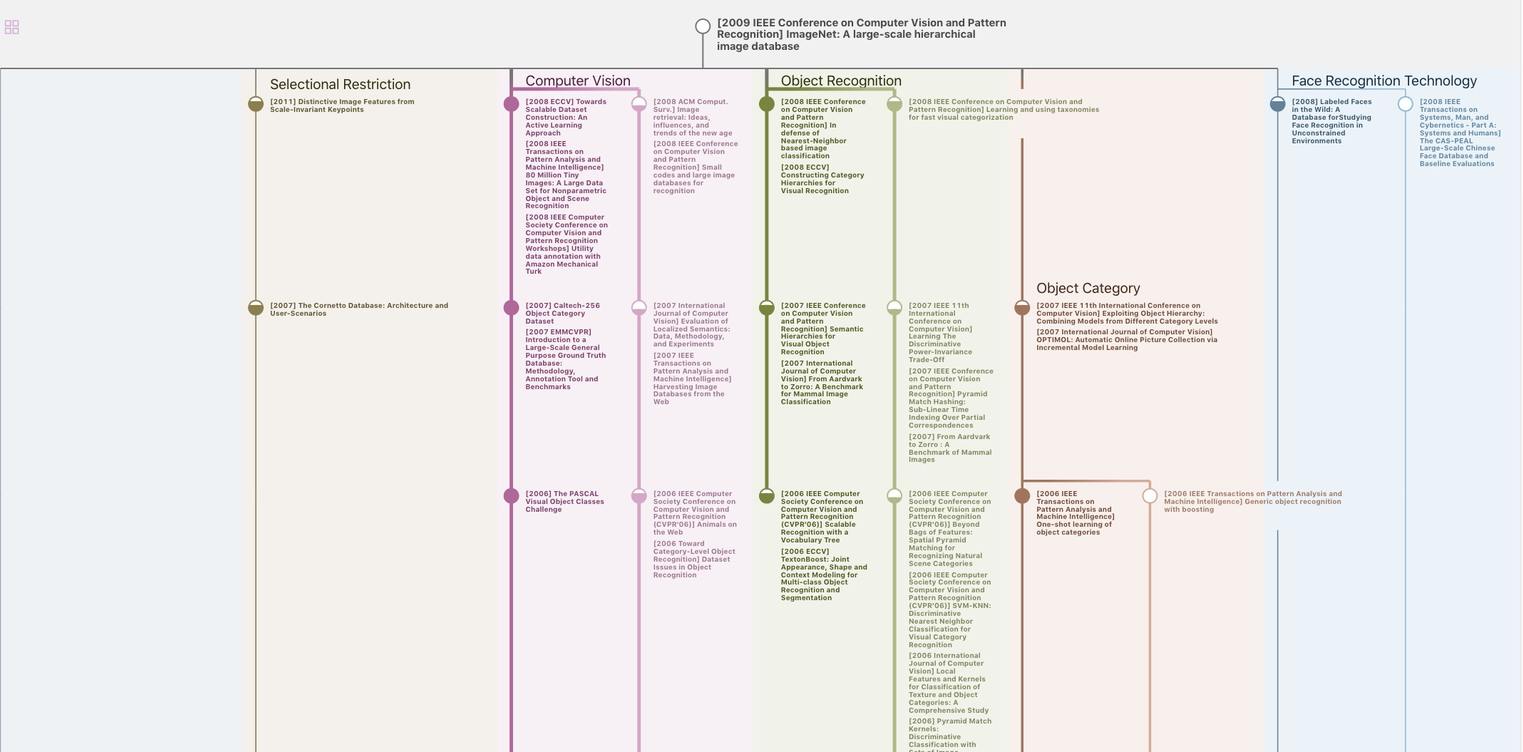
生成溯源树,研究论文发展脉络
Chat Paper
正在生成论文摘要