Perplexed by Perplexity: Perplexity-Based Data Pruning With Small Reference Models
CoRR(2024)
Abstract
In this work, we investigate whether small language models can determine
high-quality subsets of large-scale text datasets that improve the performance
of larger language models. While existing work has shown that pruning based on
the perplexity of a larger model can yield high-quality data, we investigate
whether smaller models can be used for perplexity-based pruning and how pruning
is affected by the domain composition of the data being pruned. We demonstrate
that for multiple dataset compositions, perplexity-based pruning of pretraining
data can significantly improve downstream task performance: pruning
based on perplexities computed with a 125 million parameter model improves the
average performance on downstream tasks of a 3 billion parameter model by up to
2.04 and achieves up to a 1.45× reduction in pretraining steps to reach
commensurate baseline performance. Furthermore, we demonstrate that such
perplexity-based data pruning also yields downstream performance gains in the
over-trained and data-constrained regimes.
MoreTranslated text
AI Read Science
Must-Reading Tree
Example
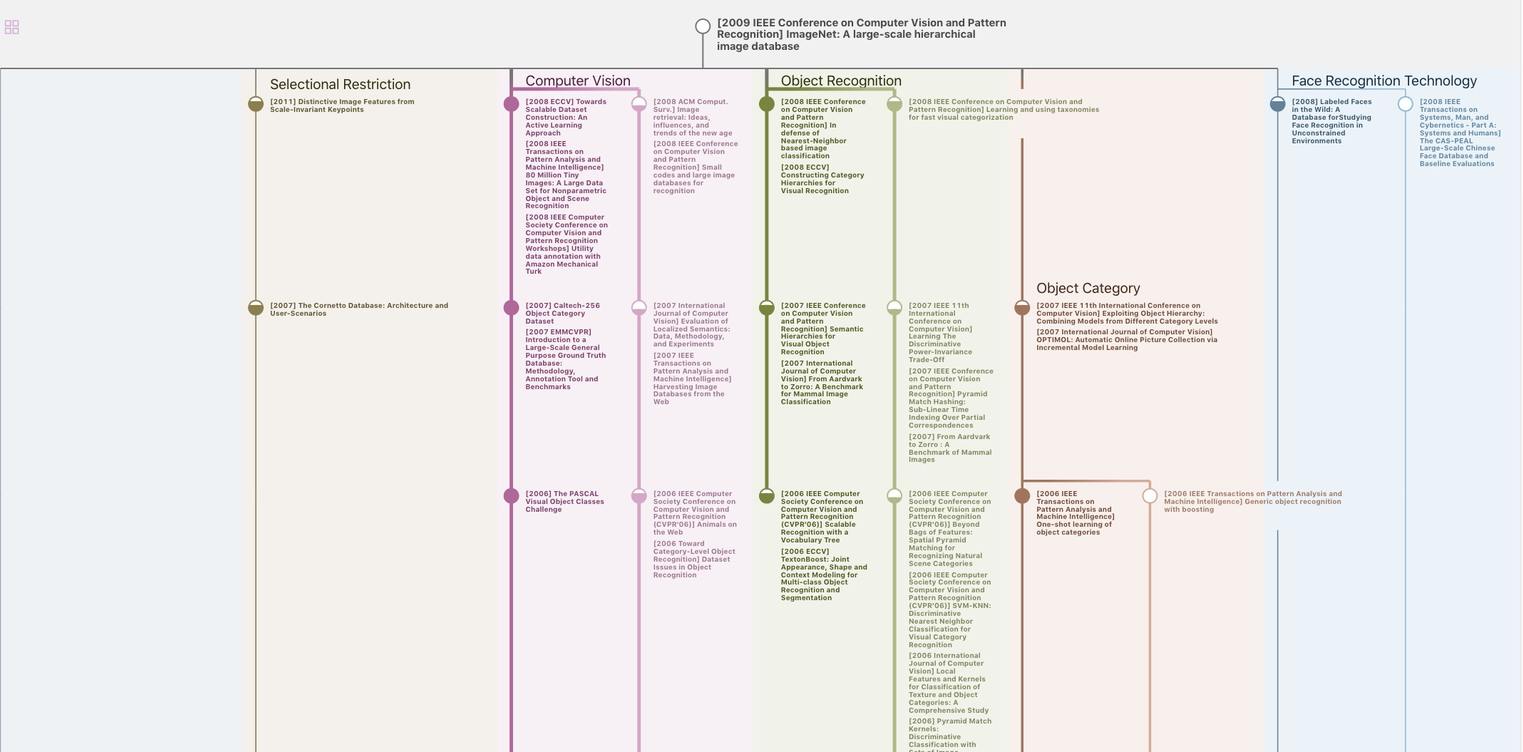
Generate MRT to find the research sequence of this paper
Chat Paper
Summary is being generated by the instructions you defined