GAN-CNN Ensemble: A Robust Deepfake Detection Model of Social Media Images Using Minimized Catastrophic Forgetting and Generative Replay Technique
Procedia Computer Science(2024)
Abstract
Deep-fake photographs are difficult to discern from real ones, especially when utilized in social media platforms. Anyone can willfully create disinformation about public personalities, politicians, and celebrities using these deep fake photographs. So, it is an important need of society to work for an effective model for its detection. The models for deep fake detection commonly use CNN-based detectors. These detectors experience a drop in performance when used for transfer learning or continual learning techniques. A significant limitation in this process is CNN's catastrophe forgetting defect. For the solution of this problem, a Generative replay technique in the form of a GAN-CNN model is implemented that works to minimize this catastrophe forgetting issue that further helps for better detection. It involves generating and storing samples from previous tasks and then replaying them during the training of new tasks which makes CNN more robust to identify deep fakes. The GAN model used in this work is traditional DCGAN improved with necessary adjustments to achieve training stability. It is observed that the model attained a good accuracy of 98.67%(training),70.08% (testing) and minimum loss with a value of 0. 0337 for 100 epochs. Also, it acquired good precision values of 68% and 72%, Recall values are 74% and 66%, and F1 scores of 71% and 69% for classes 0 and 1 respectively. The model outcome is found stable and reliable in deep fake detection under dynamic training conditions. Optimum values of evaluation parameters ensure the model's capacity to learn new tasks preserving the existing task-learning knowledge.
MoreTranslated text
Key words
Deep Learning,CNN,GAN,Catastrophe forgetting,CNN continual learning,Lifelong learning,GAN-CNN deep fake detector
AI Read Science
Must-Reading Tree
Example
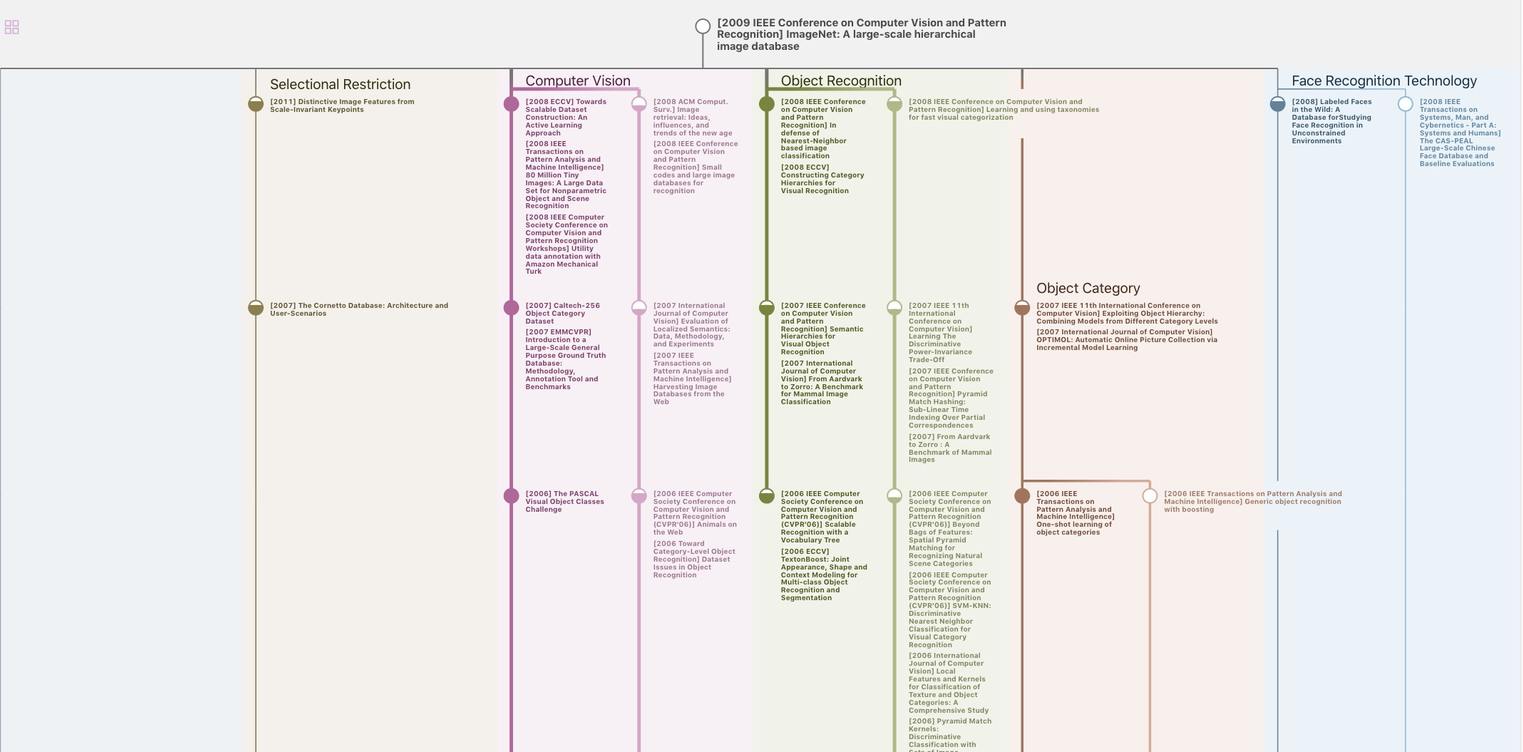
Generate MRT to find the research sequence of this paper
Chat Paper
Summary is being generated by the instructions you defined