IIoT Intrusion Detection using Lightweight Deep Learning Models on Edge Devices.
IEEE International Conference on Factory Communication Systems(2024)
摘要
In the rapidly evolving cybersecurity landscape, detecting and preventing network attacks has become crucial within the industrial sector. This study aims to explore the potential of intrusion detection by employing deep learning within edge computing, especially for the Industrial Internet of Things. Specifically, TinyML converted CNN, LSTM, Transformer-LSTM, and GCN models on the UNSW-NB15 dataset. A comprehensive dataset analysis gained insights into the nature of attack behavior data. Subsequently, a comparative analysis in an edge computing setup using Raspberry Pi units revealed that the GCN model, with its accuracy of 97.5%, was the best suited of the compared models for this application. However, the study also explored variables like time consumption, where the CNN model was the fastest out of the compared models. This research also highlights the need for continued exploration, especially in addressing dataset imbalances and enhancing model generalizability. By recognizing each model's strengths and areas of improvement, this research serves as a step toward bolstering digital safety and security in an increasingly interconnected industrial world.
更多查看译文
关键词
Network security,NIDS,Deep learning,Machine learning,UNSW-NB15,Network attacks,TinyML,IIoT,Edge computing
AI 理解论文
溯源树
样例
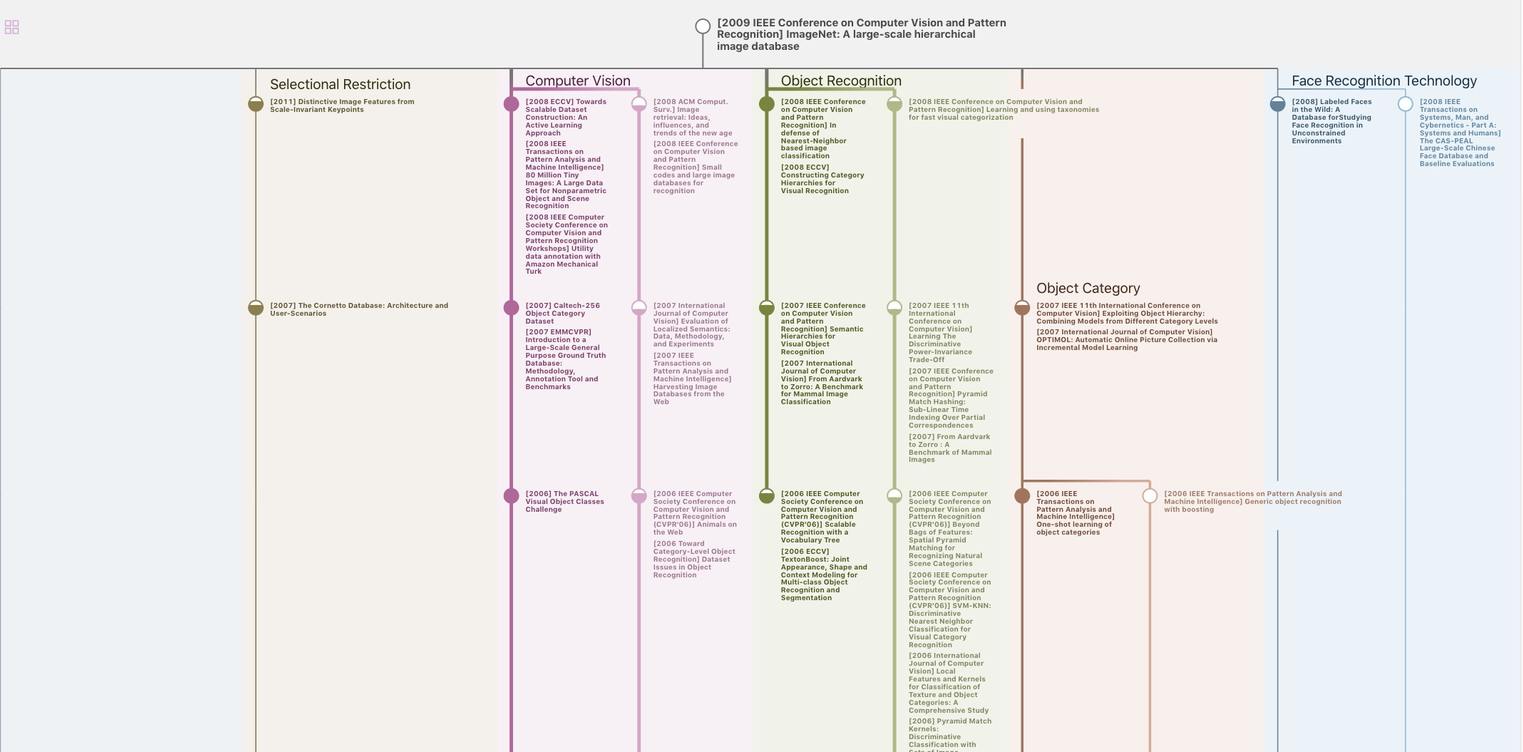
生成溯源树,研究论文发展脉络
Chat Paper
正在生成论文摘要