Monitoring of group-structured high-dimensional processes via sparse group LASSO
Annals of Operations Research(2024)
Abstract
In a general high-dimensional process, a large number of process parameters or quality characteristics is found to be featured through their dependencies and relevance. The features that have similar characteristics or behaviors in the process operation can be categorized into multiple groups. Thus, when a few quality characteristics in the process change, it is highly probable that the process shift would have occurred in a few relevant groups. Recently, several advanced statistical process control techniques are developed to monitor the changes in high-dimensional processes under sparsity. However, monitoring schemes that utilize the grouped pattern of the quality characteristics are sparse. This paper proposes a new method to monitor the high-dimensional process when the grouped structure of the process data is observed. The proposed method identifies the potentially changed groups and individual variables within the groups based on a modified sparse group LASSO (MSGL) model. Then, a monitoring statistic is obtained using MSGL-based likelihood function to test abnormality of the process. Extensive numerical studies are conducted to demonstrate the effectiveness and efficiency of the proposed method. In addition, a real-life application of a liquefied natural gas process is presented to illustrate the proposed method.
MoreTranslated text
Key words
Fault identification,High-dimensional processes,Group variable selection,Sparse group LASSO,Statistical process monitoring and control
AI Read Science
Must-Reading Tree
Example
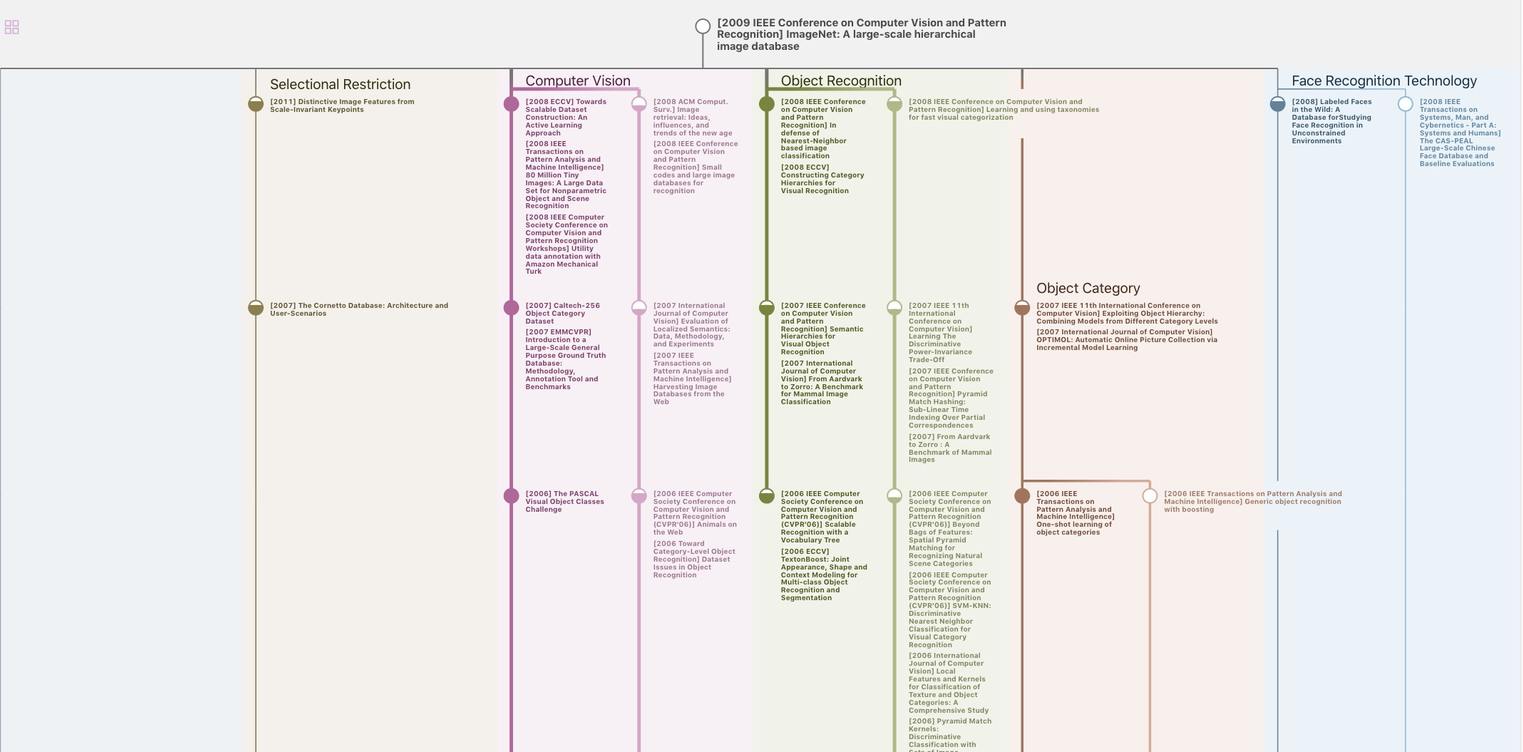
Generate MRT to find the research sequence of this paper
Chat Paper
Summary is being generated by the instructions you defined