Student Performance Prediction: A Co-Evolutionary Hybrid Intelligence model
Procedia Computer Science(2024)
摘要
Abstract - Student performance prediction has a pivotal role in educational institutions, providing valuable insights into students' academic outcomes and aiding educators in implementing personalized interventions. In the previous works, many variants of ML and AI techniques have been implemented with high accuracy. However, the lack of transparency due to the heterogeneous nature of features that lead to biases, forced inequalities or even challenges in interpreting the model implications have not been addressed. An approach that harnesses the power of hybrid intelligence, combining human expertise with artificial intelligence (AI), to enhance transparency and accountability in the decision-making process of student performance prediction is required. Co-evolutionary Hybrid Intelligence (CHI) approach, will not only discover interdisciplinary facets including past exam scores, attendance records, demographic information, and socio-economic factors but also interpret the influence of every component i.e., teacher, student, and learning environment to predict student performance. Two modules are discussed in this paper. The first module classifies the teachers into two classes of expertise by comparing the accuracy performance of six well-known machine learning techniques namely Logistic Regression (LR), MultiLayer Perceptron(MLP), Naive Bayes (NB), Support Vector Machine (SVM), K-Nearest Neighbor (kNN), and Random Forest (RF). Second, we propose a multiclass student performance prediction model (RLCHI) built upon a combination of reinforcement learning (RL) algorithms that will aid the model to perceive patterns and adjustments inherently and expert teachers' opinions(CHI) from the first model that will bring invaluably contextual understanding, nuanced judgment, and a deep knowledge of pedagogy to the equation. The performance of the RLCHI model is evaluated. The obtained results show that the proposed model scores better than models built only on the RL algorithm on the same dataset. The accuracy, precision, recall, and Fl score of the model achieved with hybrid intelligence is 79.5, 49.9, 52.3, 53.2 when compared to 71.9,48.30,46.6,46.1 achieved with only RL respectively.
更多查看译文
关键词
Coevolution,Performance,Intelligence,Reinforcement Learning
AI 理解论文
溯源树
样例
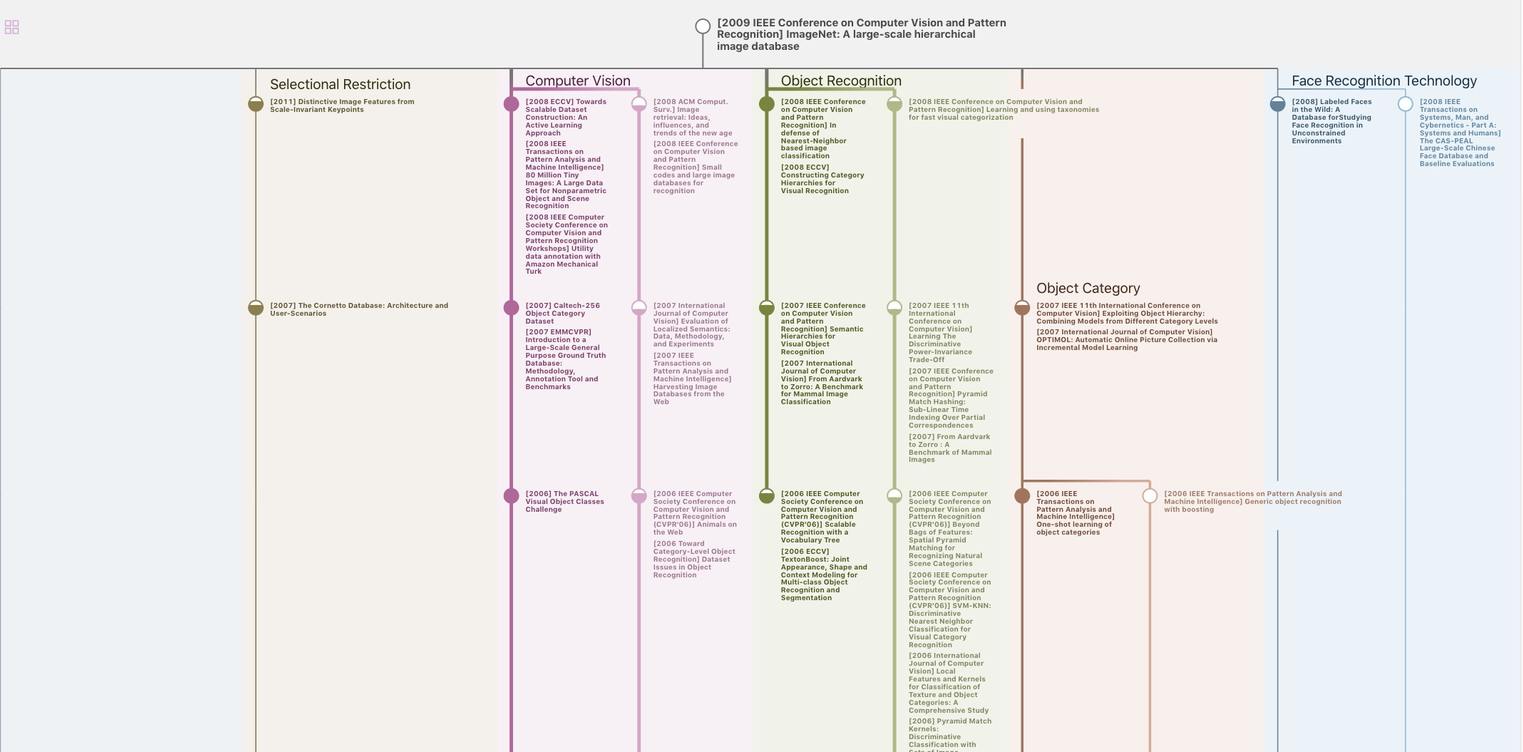
生成溯源树,研究论文发展脉络
Chat Paper
正在生成论文摘要