Enhancing Prediction of Myocardial Recovery After Coronary Revascularization: Integrating Radiomics from Myocardial Contrast Echocardiography with Machine Learning.
International journal of general medicine(2024)
摘要
Introduction:Chronic coronary artery disease (CAD) management often relies on myocardial contrast echocardiography (MCE), yet its effectiveness is limited by subjective interpretations and difficulty in distinguishing hibernating from necrotic myocardium. This study explores the integration of machine learning (ML) with radiomics to predict functional recovery in dyskinetic myocardial segments in CAD patients undergoing revascularization, aiming to overcome these limitations.
Methods:This prospective study enrolled 55 chronic CAD patients, dividing into training (39 patients, 205 segments) and testing sets (16 patients, 68 segments). Dysfunctional myocardial segments were identified by initial wall motion scores (WMS) of ≥2 (hypokinesis or higher). Functional recovery was defined as a decrease of ≥1 grade in WMS during follow-up echocardiography. Radiomics features were extracted from dyssynergic segments in end-systolic phase MCE images across five cardiac cycles post- "flash" impulse and processed through a five-step feature selection. Four ML classifiers were trained and compared using these features and MCE parameters, to identify the optimal model for myocardial recovery prediction.
Results:Functional improvement was noted in 139 out of 273 dyskinetic segments (50.9%) following revascularization. Receiver Operating Characteristic (ROC) analysis determined that myocardial blood flow (MBF) was the most precise clinical predictor of recovery, with an area under the curve (AUC) of 0.770. Approximately 1.34 million radiomics features were extracted, with nine features identified as key predictors of myocardial recovery. The random forest (RF) model, integrating MBF values and radiomics features, demonstrated superior predictive accuracy over other ML classifiers. Validation of the RF model on the testing dataset demonstrated its effectiveness, evidenced by an AUC of 0.821, along with consistent calibration and clinical utility.
Conclusion:The integration of ML with radiomics from MCE effectively predicts myocardial recovery in CAD. The RF model, combining radiomics and MBF values, presents a non-invasive, precise approach, significantly enhancing CAD management.
更多查看译文
关键词
coronary artery disease,myocardial contrast echocardiography,radiomics,machine learning,myocardial recovery prediction,random forest
AI 理解论文
溯源树
样例
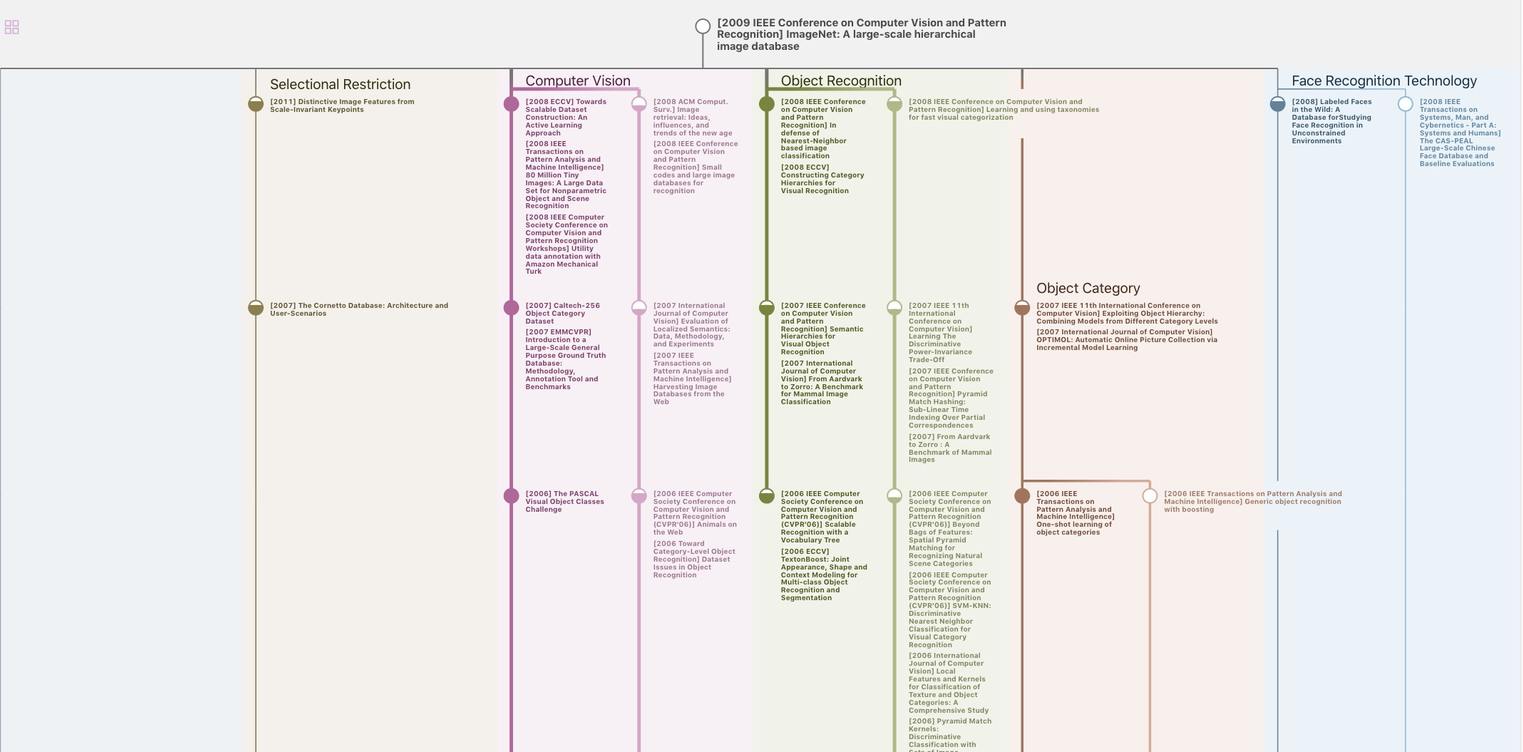
生成溯源树,研究论文发展脉络
Chat Paper
正在生成论文摘要