Full-Model-Free Adaptive Graph Deep Deterministic Policy Gradient Model for Multi-Terminal Soft Open Point Voltage Control in Distribution Systems
Journal of Modern Power Systems and Clean Energy(2024)
Abstract
High renewable energy penetration induces sharply-fluctuating feeder power, leading to voltage deviation in active distribution systems. To prevent voltage violations, multi-terminal soft open points (M-SOPs) have been integrated into the distribution systems to enhance voltage control flexibility. However, the M-SOP control model recalculated in real-time cannot adapt to the rapid fluctuations of PVs, fundamentally limiting the voltage controllability of M-SOPs. To address this issue, a full-model-free adaptive graph deep deterministic policy gradient framework (FAG-DDPG) is proposed for M-SOP voltage control. Specifically, the attention-based adaptive graph convolutional network (AGCN) is leveraged to extract the complex correlation features of nodal information to improve the policy learning ability. Then, the AGCN-based surrogate model is trained to replace the power flow calculation to achieve model-free control. Furthermore, the DDPG algorithm allows FAG-DDPG to learn an M-SOP optimal control strategy by continuous interactions with the surrogate model. Numerical tests have been performed on modified IEEE 33-, 123-node, and a real 76-node distribution system. The effectiveness and generalization ability are demonstrated by the proposed model's results.
MoreTranslated text
Key words
Soft open point,graph attention,graph convolutional network,reinforcement learning,deep deterministic policy gradient
AI Read Science
Must-Reading Tree
Example
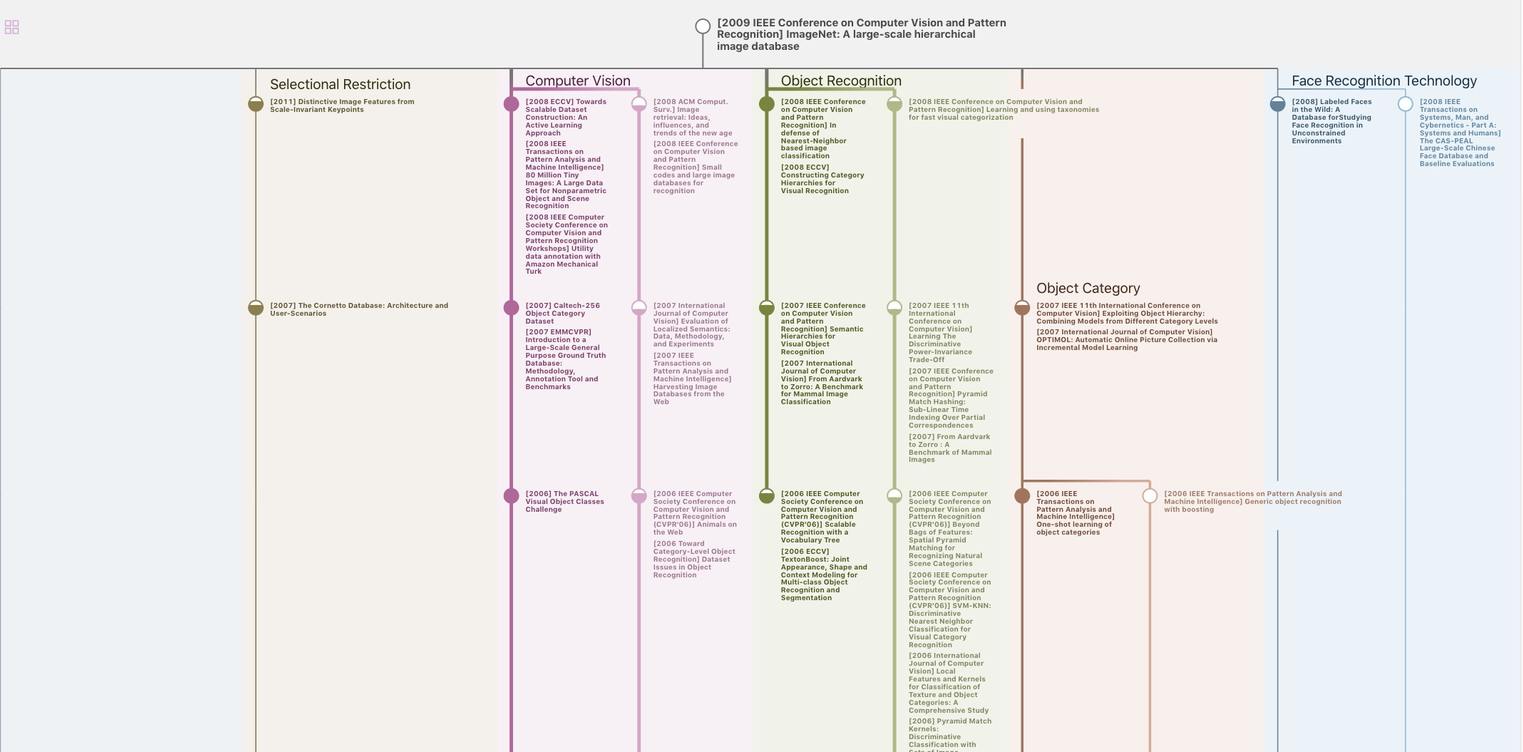
Generate MRT to find the research sequence of this paper
Chat Paper
Summary is being generated by the instructions you defined