A Multimodal Ensemble Deep Learning Model for Functional Outcome Prognosis of Stroke Patients.
Journal of stroke(2024)
摘要
BACKGROUND AND PURPOSE:The accurate prediction of functional outcomes in patients with acute ischemic stroke (AIS) is crucial for informed clinical decision-making and optimal resource utilization. As such, this study aimed to construct an ensemble deep learning model that integrates multimodal imaging and clinical data to predict the 90-day functional outcomes after AIS.
METHODS:We used data from the Korean Stroke Neuroimaging Initiative database, a prospective multicenter stroke registry to construct an ensemble model integrated individual 3D convolutional neural networks for diffusion-weighted imaging and fluid-attenuated inversion recovery (FLAIR), along with a deep neural network for clinical data, to predict 90-day functional independence after AIS using a modified Rankin Scale (mRS) of 3-6. To evaluate the performance of the ensemble model, we compared the area under the curve (AUC) of the proposed method with that of individual models trained on each modality to identify patients with AIS with an mRS score of 3-6.
RESULTS:Of the 2,606 patients with AIS, 993 (38.1%) achieved an mRS score of 3-6 at 90 days post-stroke. Our model achieved AUC values of 0.830 (standard cross-validation [CV]) and 0.779 (time-based CV), which significantly outperformed the other models relying on single modalities: b-value of 1,000 s/mm2 (P<0.001), apparent diffusion coefficient map (P<0.001), FLAIR (P<0.001), and clinical data (P=0.004).
CONCLUSION:The integration of multimodal imaging and clinical data resulted in superior prediction of the 90-day functional outcomes in AIS patients compared to the use of a single data modality.
更多查看译文
关键词
modified rankin scale,stroke,prognosis,deep learning
AI 理解论文
溯源树
样例
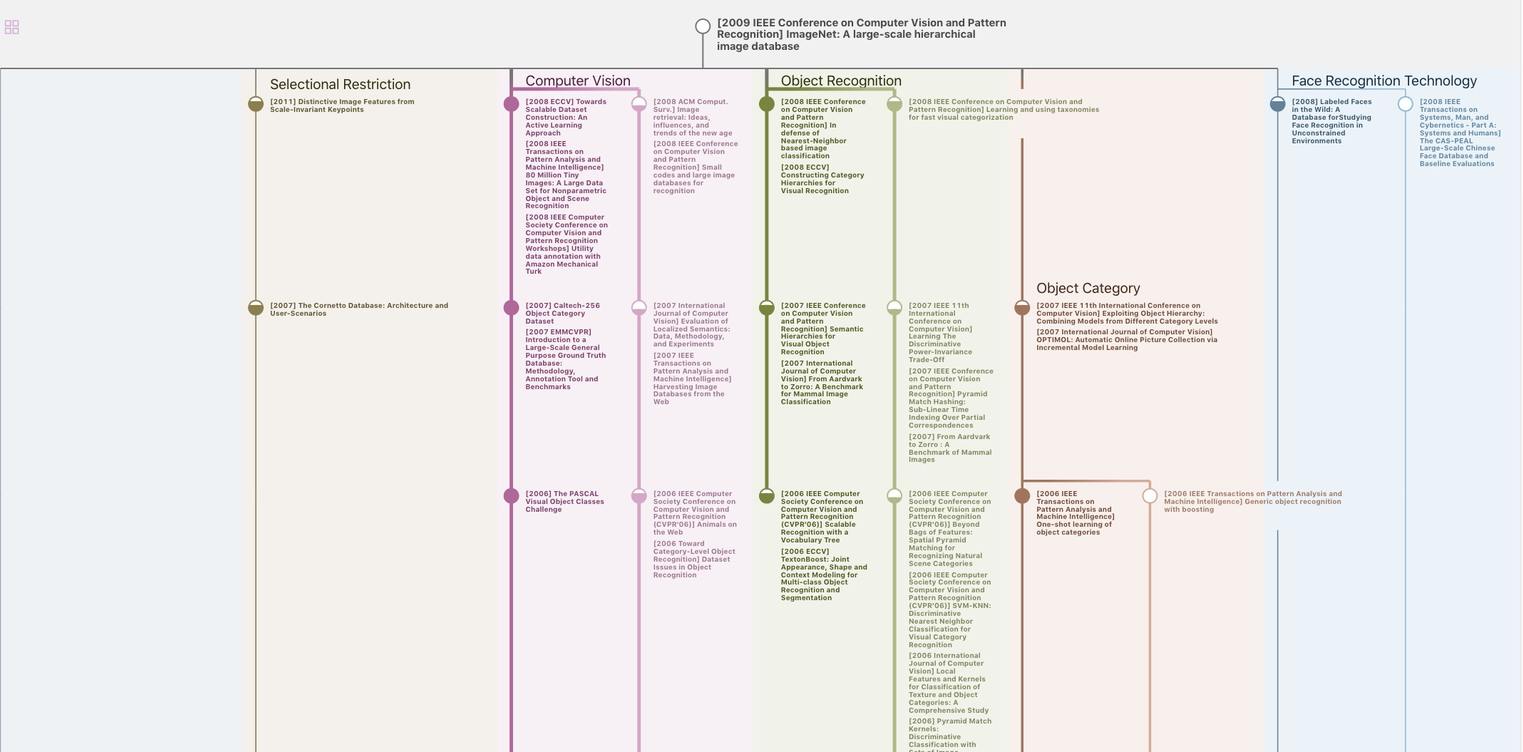
生成溯源树,研究论文发展脉络
Chat Paper
正在生成论文摘要