An Investigation into Ensemble Learning Techniques for Evaluating Soil Fertility through Analytical Approaches
Procedia Computer Science(2024)
摘要
At the heart of India’s livelihood lies agriculture, a foundational and ever-evolving sector. The realm of agriculture faces modern challenges, encompassing unpredictable climate fluctuations, insufficient irrigation infrastructure, and erratic weather conditions. Amidst these challenges, machine learning has emerged as a valuable tool for evaluating soil fertility and Crop assessment in agriculture. Ensemble machine learning methodologies have garnered attention for their potential to enhance predictive capabilities. These techniques involve constructing meta-classifiers that collectively contribute to improved predictive accuracy. This paper centers on the analysis of soil data acquired from testing laboratories, to predict fertility based on a comprehensive dataset. The study employs prominent ensemble machine learning algorithms, specifically focusing on boosting techniques, to elevate predictive accuracy and ensure heightened consistency.The assessment of soil fertility categories involved the examination of 12 carefully chosen attributes. Diverse soil parameters were measured to facilitate the prediction of soil fertility levels. The outcomes of the experimentation revealed that the application of the boosting technique using the Xgboost algorithm yielded superior accuracy compared to alternative ensemble classifiers, achieving a remarkable 96% accuracy rate.
更多查看译文
关键词
Soil Fertility prediction,Agriculture,Feature Selection,Preprocessing,Correlation,Ensemble Learning,Machine Learning,Boosting,Bagging,Confusion matrix
AI 理解论文
溯源树
样例
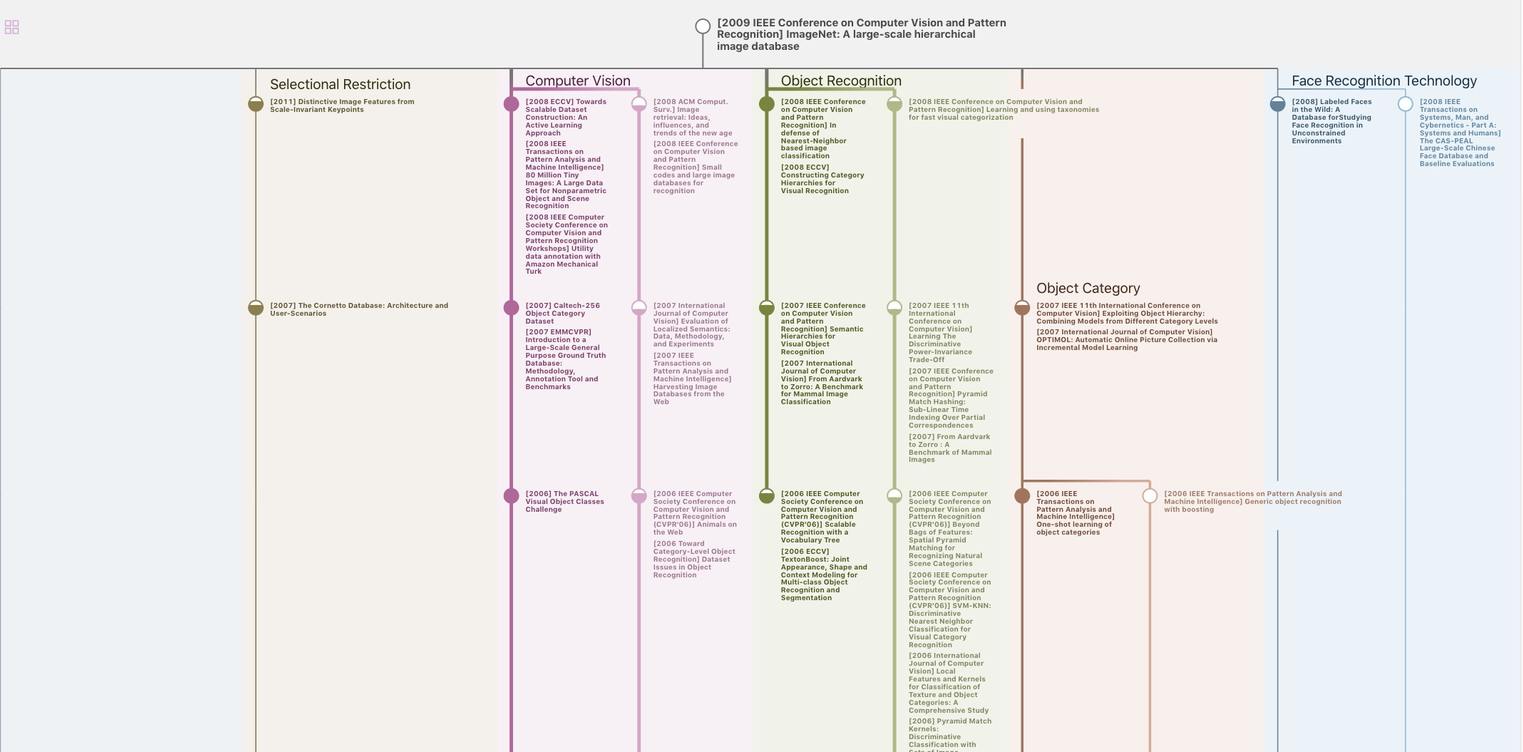
生成溯源树,研究论文发展脉络
Chat Paper
正在生成论文摘要