Exploring Large Language Model for Generic and Robust State-of-Charge Estimation of Li-ion Batteries: A Mixed Prompt Learning Method
Energy(2024)
Abstract
State-of-charge (SOC) estimation is important to ensure safe functioning of Li-ion batteries (LIBs). However, distinct measurements, harsh temperatures, and dynamic operations pose challenges in estimating intricate SOC changes of multiple LIBs with one estimator. Hence, this paper proposes a novel mixed prompt learning method to explore a large-scale pretrained language model (PLM) for SOC estimation. A new hard prompt generator is proposed to translate LIB data into instruction and answer text, eliciting the inherent representational capability of PLM to jointly learn the sequential patterns and contextual semantics of measurements through language modeling for accurate SOC estimation. A new soft prompt adapter is proposed to encode task-specific information of different LIBs into a small set of independent vectors, ensuring PLM adaptation with minimal parameters while maintaining good generalization. By integrating soft prompt vectors along the forward propagation, the hidden states of PLM are dynamically regulated to characterize volatile and variable SOC for robust estimation. Extensive experiments show that by leveraging mixed hard-soft prompts, PLM can make accurate, generic, and robust estimates for multiple LIBs simultaneously in subzero temperature, charge-pause-discharge, and low-capacity discharge scenarios, with the average MAE, RMSE, and MAX of all tasks as low as 1.20%, 1.51%, and 5.29%, respectively.
MoreTranslated text
Key words
Large language model,Li-ion batteries,prompt,state-of-charge estimation
AI Read Science
Must-Reading Tree
Example
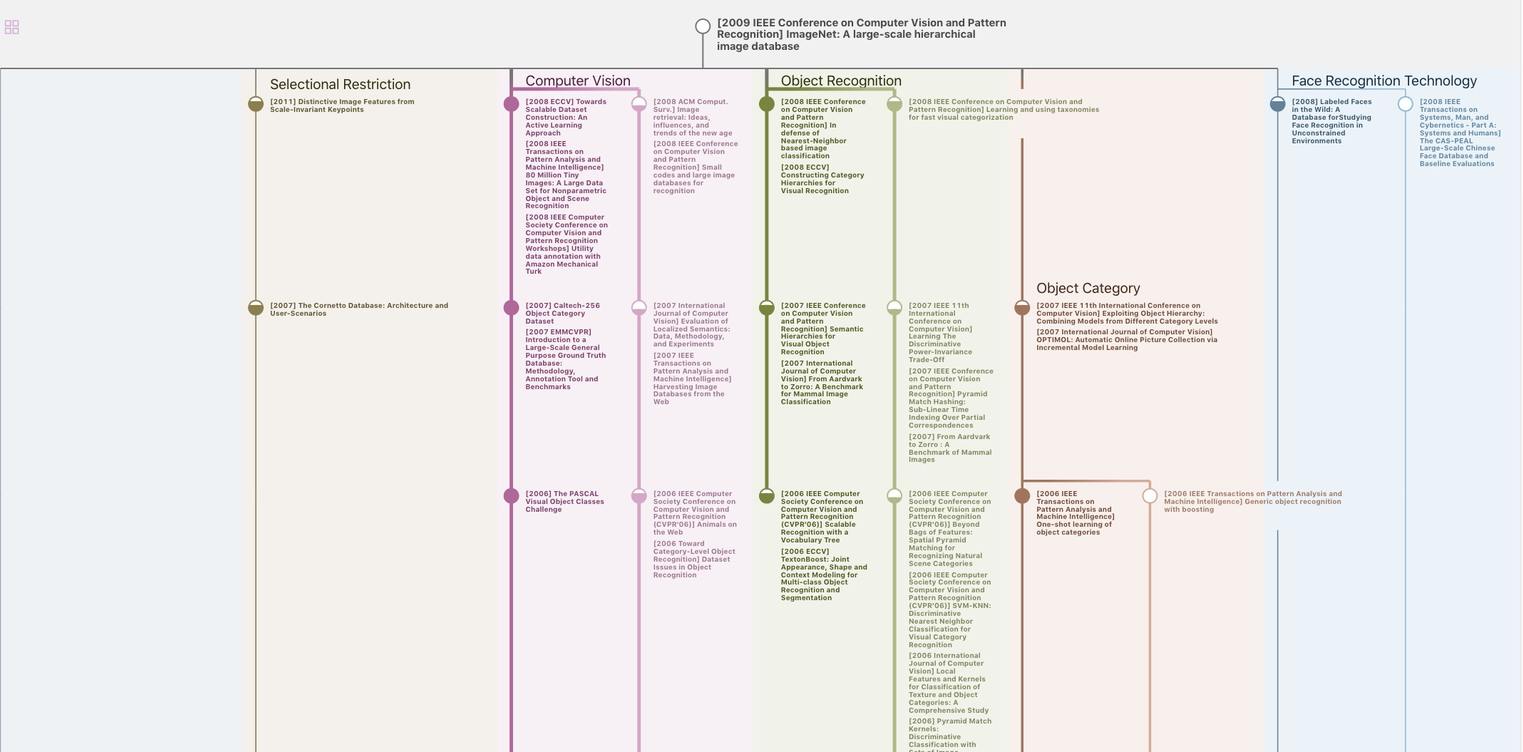
Generate MRT to find the research sequence of this paper
Chat Paper
Summary is being generated by the instructions you defined