Performance of Machine Learning Models in Predicting Common Bean (Phaseolus vulgaris L.) Crop Nitrogen Using NIR Spectroscopy
crossref(2024)
Abstract
Beans are the most widely used protein source in the world and their productivity is directly linked to nitrogen (N). The short crop cycle imposes the need for fast methodologies for N quantification. In this work, we evaluated the performance of four machine learning algorithms in nitrogen prediction using NIR spectroscopy. Increasing doses of nitrogen were applied to the plants and leaf reflectance was collected. Weka software was used to test the algorithms. The selection of the most effective spectral zones was made with the VIP. Considering predictions with the whole NIR, the best results were achieved with RF (R2 = 0,84 and RMSE = 2,69 g kg-1) and KNN (R2 = 0,77 and RMSE = 3,86 g kg-1). The intervals of 700-740 nm and 983-995 nm were considered the most important for the study of N. More efficient predictions were verified when only spectral regions screened by VIP were included, increasing the accuracy of the RF, KNN and M5 models by 6%, 4% and 8%, respectively. The efficiency of N prediction based on NIR reflectance combined with machine learning was verified. This approach can optimize the management of nitrogen fertilization, serving as an important tool in precision agriculture.
MoreTranslated text
AI Read Science
Must-Reading Tree
Example
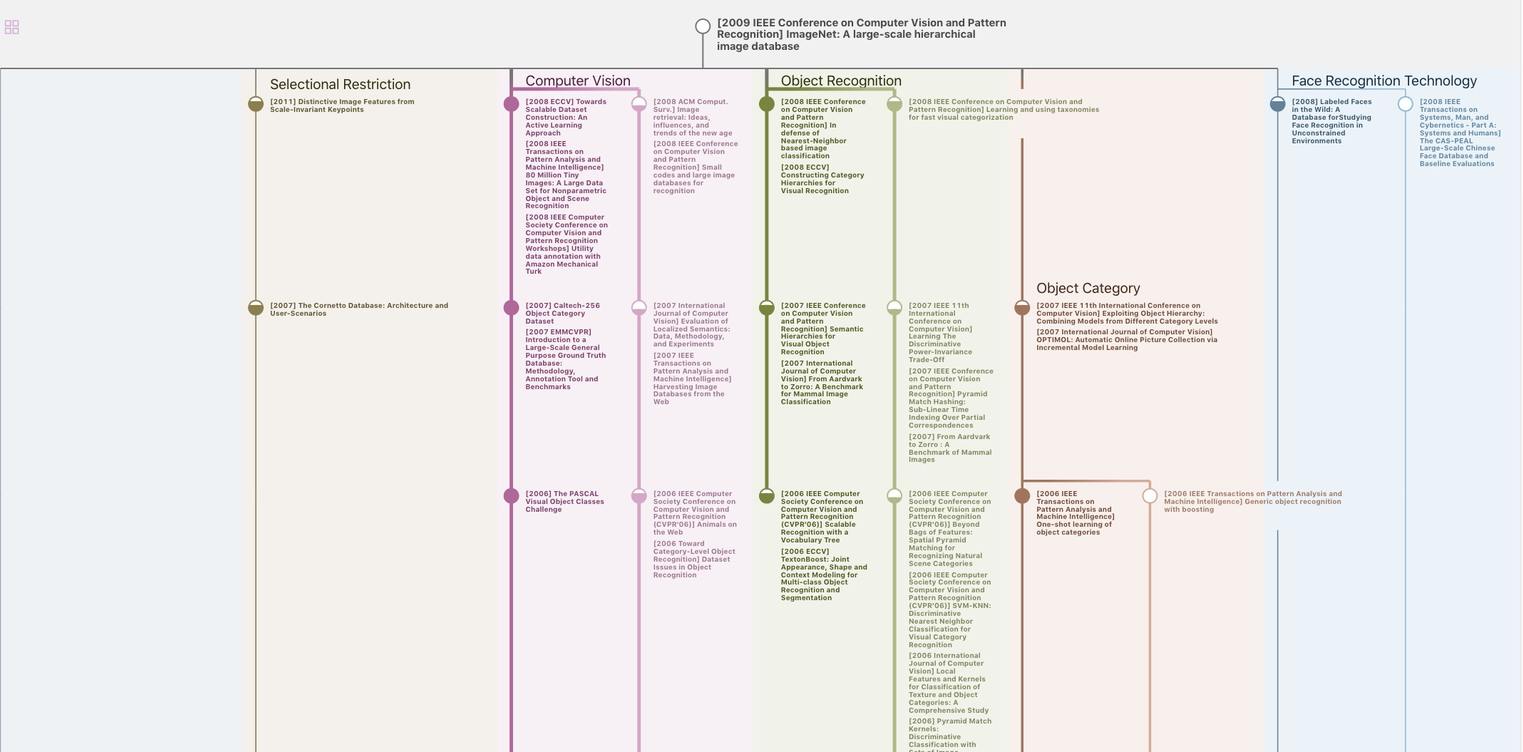
Generate MRT to find the research sequence of this paper
Chat Paper
Summary is being generated by the instructions you defined