EfficientNet Architecture and Attention Mechanism-Based Wheat Disease Identification Model
Procedia Computer Science(2024)
Abstract
The image-based automatic plant disease identification has emerged as a prominent research area, driven by recent advancements in machine learning algorithms. Conventional disease detection methods rely on manual feature extraction from acquired images, involving extensive expertise from experts and pathologists. This labor-intensive practice is often time-consuming and impractical. With the recent advancements in digital technologies and computer vision, Deep neural networks address these challenges by learning the features automatically. This paper focuses on the EfficientNet B0 architecture-based deep neural network model integrated with a convolutional block attention mechanism module for identifying major wheat crop diseases. The attention module in the EfficientNet B0 model improves its performance by emphasizing the disease-infected regions in leaf images. To evaluate the proposed methodology, experiments were conducted on the WheatRust21 image dataset, comprising 6556 images of three major fungal diseases, namely, stripe rust, leaf rust, and stem rust, collected in natural field conditions from 2019 to 2021. Our developed EfficientNet B0-CBAM model performed well with a testing accuracy of 98.70% and an impressive F1 score of 98% on the dataset. The Grad-CAM visualization demonstrates that the attention module focuses more on the infected region and plays a crucial role in wheat disease identification. The results are quantitatively substantial, with the model achieving high accuracy and other evaluation metrics scores with an average of 98.5 %. These findings hold promise for practical applications in agriculture and sustainable crop management.
MoreTranslated text
Key words
Attention mechanism,Convolutional Neural Network,EfficientNet,Plant disease identification,Image classification
AI Read Science
Must-Reading Tree
Example
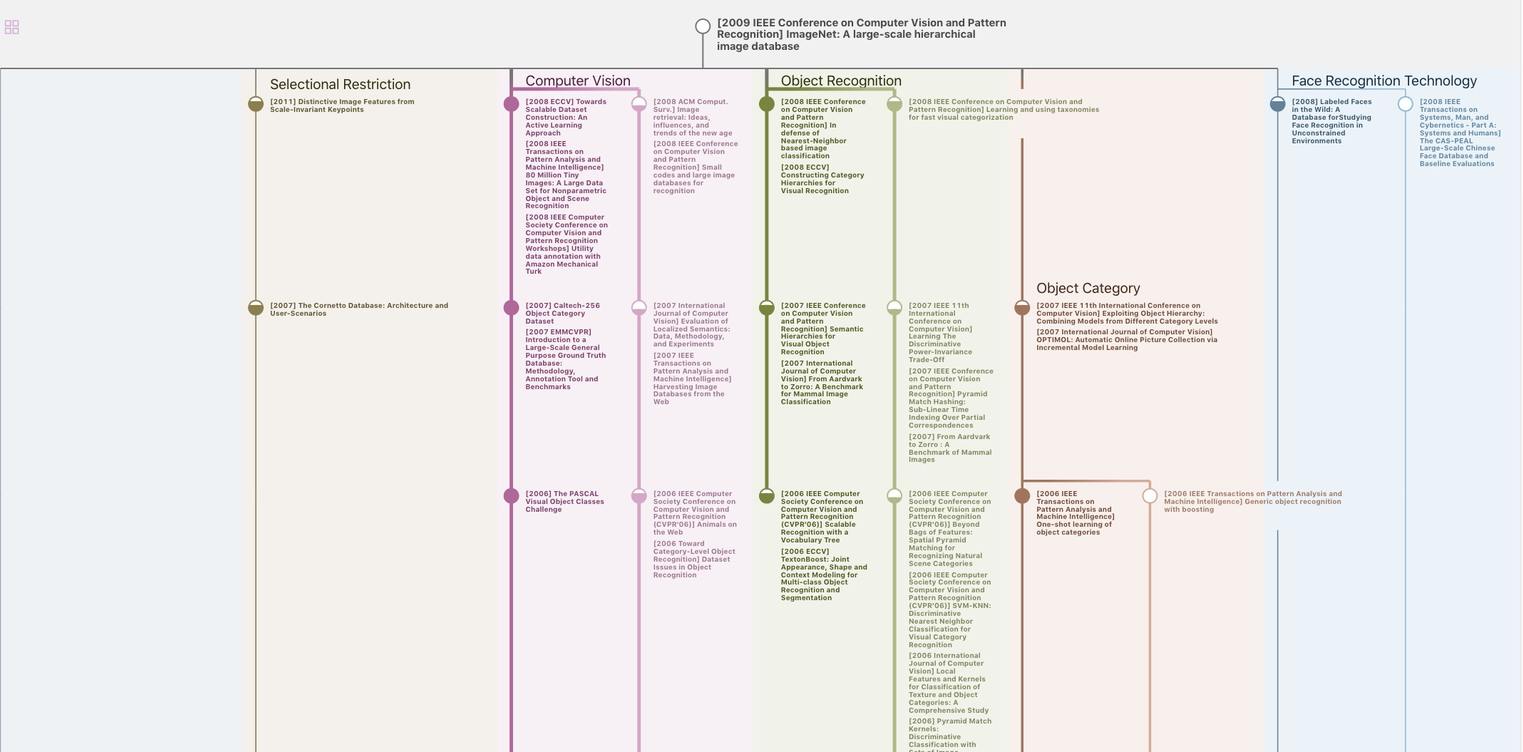
Generate MRT to find the research sequence of this paper
Chat Paper
Summary is being generated by the instructions you defined