Response Generation in Social Network With Topic and Emotion Constraints
IEEE TRANSACTIONS ON COMPUTATIONAL SOCIAL SYSTEMS(2024)
摘要
Response generation is the task of automatically generating human-like content based on the provided context. One of its prominent applications is to simulate realistic response content for social network posts. In the digital age, social network platforms play a vital role in information exchange and social interaction. This study focuses on response generation techniques for the platform of public opinion evolution simulation that simulate realistic response content, enabling a deeper understanding of the emotional expressions of network users. Recent advancements in deep learning techniques, particularly the sequence-to-sequence (Seq2Seq) model, have shown promise in the response generation field. However, we still face two challenges: content variety, topic and emotion relevancy. To this end, we propose the EmoTG-ETRS model which comprises three parts. The first is a response generation module based on Transformer architecture. Then, an auxiliary emotion improvement module is incorporated to enhance the emotional expressiveness of the response candidates. Finally, a reverse selection module, which combines maximum mutual information (MMI) evaluation, emotional expression evaluation, and topic consistency evaluation, is devised to select the highest-scoring response. Extensive experiments have been conducted to evaluate the effectiveness of the proposed model and the results demonstrate that the EmoTG-ETRS model improves the quality of produced replies in terms of topic consistency and emotional accuracy rate when compared with the SOTA research works.
更多查看译文
关键词
Computational modeling,Transformers,Decoding,Emotion recognition,Task analysis,Social networking (online),Electronic mail,Emotion constraint,social response generation,topic relevance
AI 理解论文
溯源树
样例
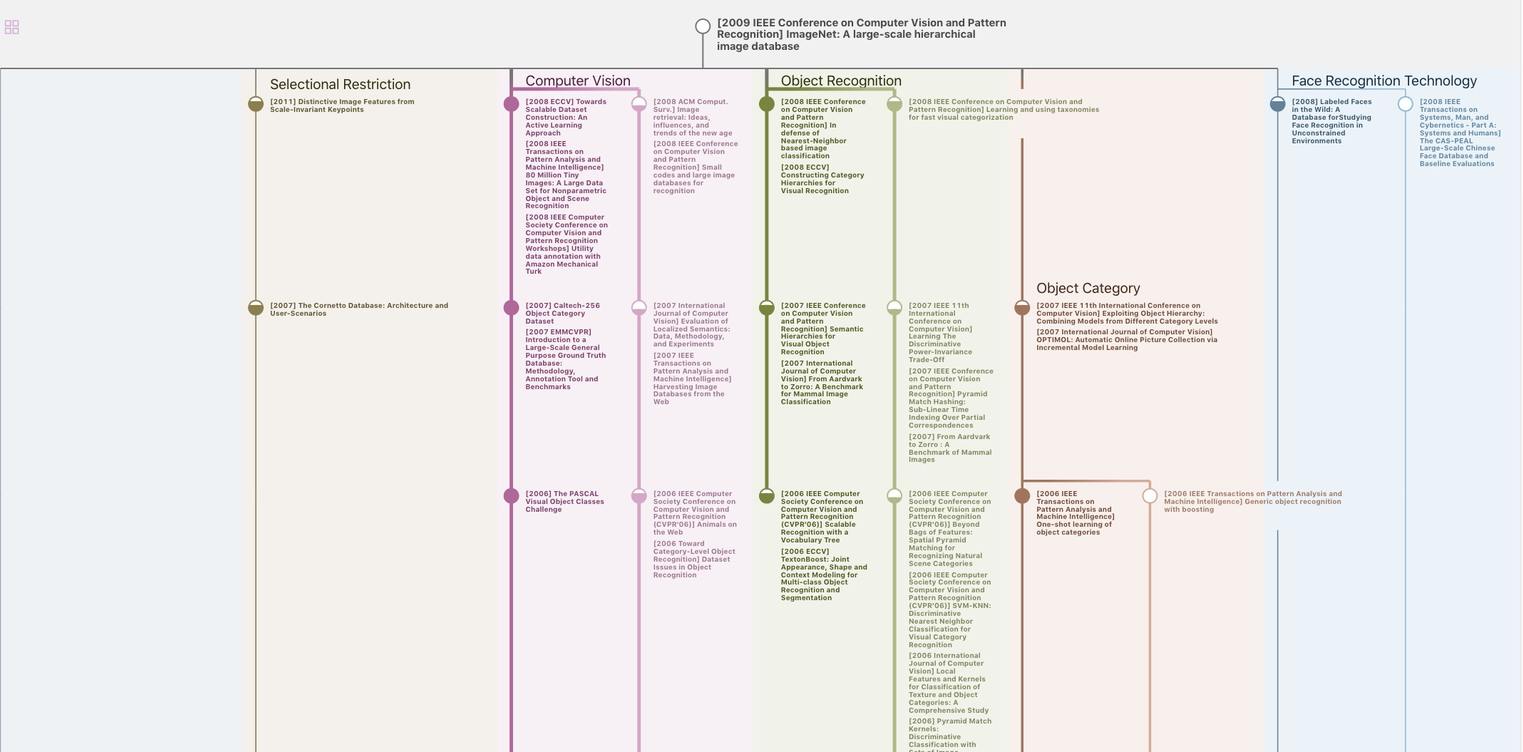
生成溯源树,研究论文发展脉络
Chat Paper
正在生成论文摘要