Cognitive Engagement Detection of Online Learners Using GloVe Embedding and Hybrid LSTM.
International Conference on Augmented Intelligence and Intelligent Tutoring Systems(2024)
摘要
This paper presents a method for classifying discussion posts of online courses, aiming to improve students’ cognitive engagement in online learning. This method utilizes deep learning models including a GloVe embedding and a hybrid long short-term memory (LSTM) network within an educational framework called interactive, constructive, active, and passives (ICAP), which classifies the students posts into interactive, constructive, active, and passives classes. These classes quantify the students’ level of cognitive engagement in their online course discussion posts. We used textual attributes and label-specific characteristics (e.g., text length, sentiment polarity, and subjectivity) to gain comprehensive insights into the forum posts’ emotional and cognitive depth. We further refined these features with a pre-trained GloVe embedding, which enhanced the classification accuracy. We interpreted the model’s decision-making process using local interpretable model-agnostic explanations (LIME) for added transparency and interpretability. By employing deep learning models with ICAP and LIME, this study demonstrates an effective use of the proposed system for improving student cognitive engagement in online learning.
更多查看译文
AI 理解论文
溯源树
样例
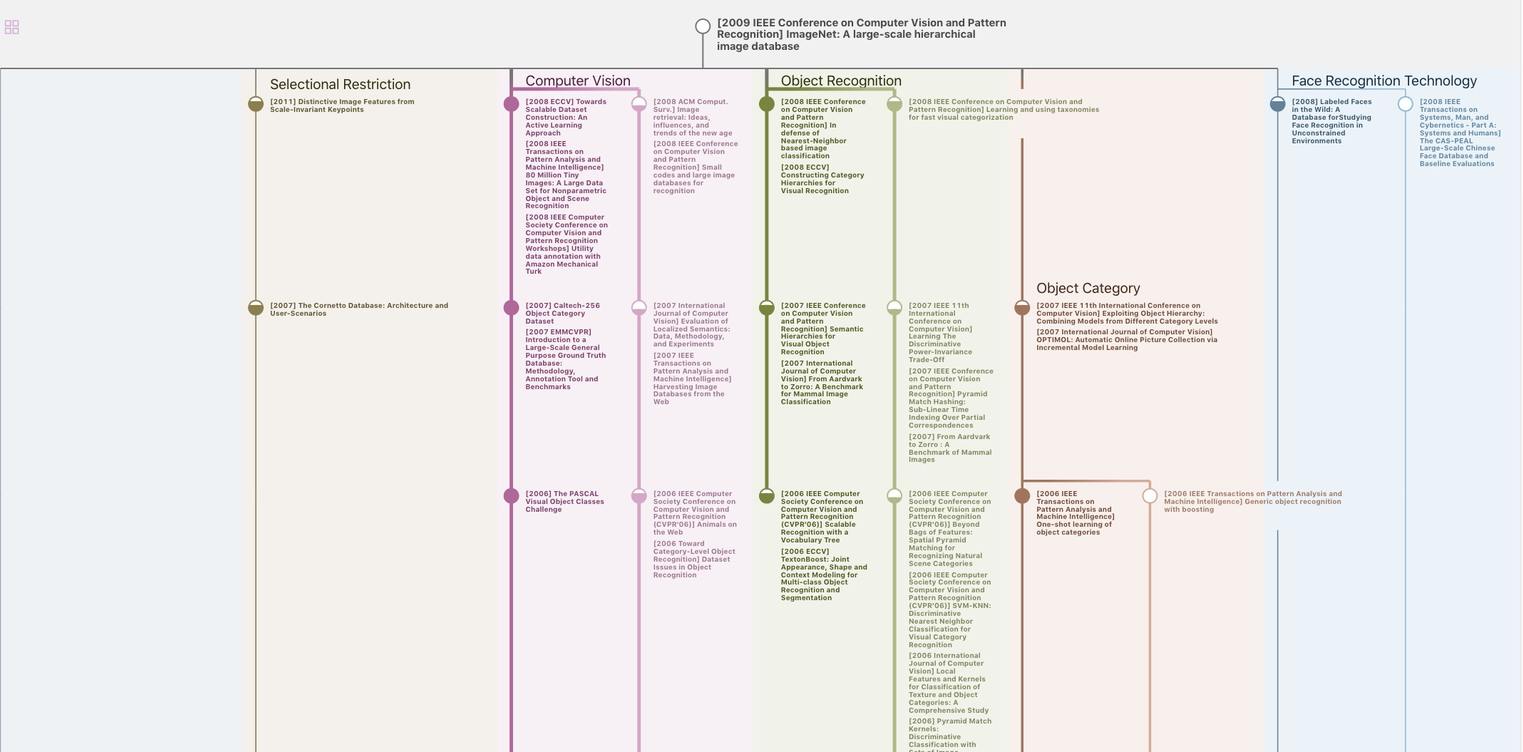
生成溯源树,研究论文发展脉络
Chat Paper
正在生成论文摘要