Land use predicition accuracy of different supervised classifiers over agriculture and livestock economy-based municipality in Brazil
REMOTE SENSING APPLICATIONS-SOCIETY AND ENVIRONMENT(2024)
Abstract
The dynamics of land use and land cover (LULC) are of great importance for the management of natural resources, sustainable development and urban planning over geographic space, and this condition is sometimes supported by geoprocessing and remote sensing techniques. In addition, machine learning methods automate the classification and modeling of spatialized prediction processes on orbital images, and with the high precision and adherence of these data, important results and conclusions are the result of these methods. The decision for which classification typology presents the best results is related to the application and considering the LULC prediction as input to a cellular automata (CA) network, the performances of Classification and Regression Tree (CART), Random Forest (RF) and Minimum Distance (MID) for predicting land use and occupation in Sinop, Brazil were assessed. Using the median of the reference years 2013 and 2015 to create a transition potential modelling (TPM) neural network, and then predict a scenario in 2017, the performance was verified with Kappa and global accuracy (OA) statistics. With the highest performance, the RF typology reached the best performance in an area of mostly agricultural occupation, separated into four classes (native forest, urban area, water and bare soil/agricultural activity). The errors inherent to each classifier were decisive for a greater prediction error, where the other classifiers (CART and MID) mistakenly classified the urban area class, but which statistically were not gross errors. Considering the ground truth and the best statistical performance, the prediction of land use and occupation for a scenario as seen in Sinop potentially achieves better results with the Random Forest classifier.
MoreTranslated text
Key words
Random forest,Neural net,Decision tree,Landsat
AI Read Science
Must-Reading Tree
Example
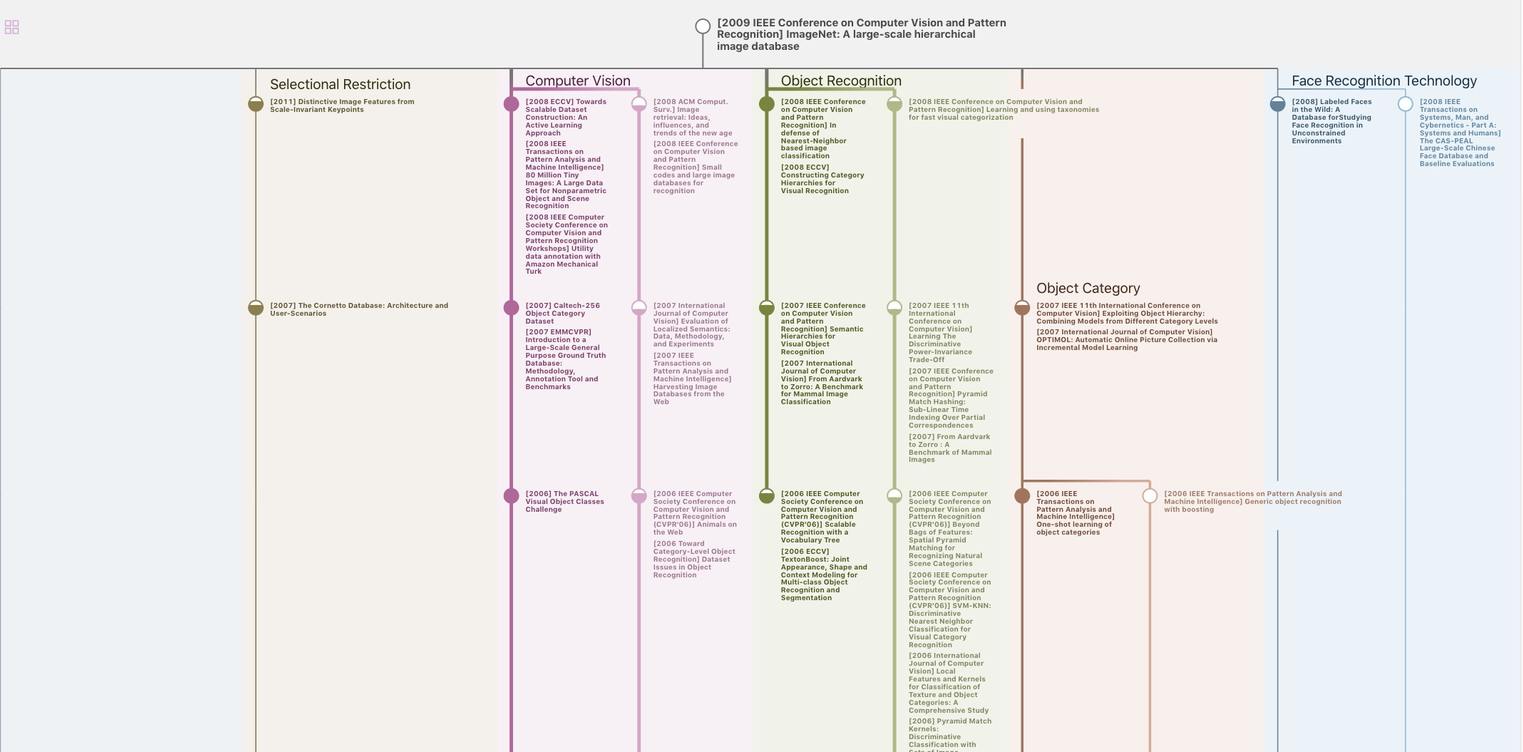
Generate MRT to find the research sequence of this paper
Chat Paper
Summary is being generated by the instructions you defined