Local Scour Depth Around Bridge Piers: Performance Evaluation of Dimensional Analysis-based Empirical Equations and AI Techniques
KSCE Journal of Civil Engineering(2024)
摘要
Artificial Intelligence (AI) techniques, such as Artificial Neural Networks (ANN) and Adaptive Neuro-Fuzzy Inference Systems (ANFIS), and dimensional analysis-based empirical equations (DAEEs), can estimate scour depth around bridge piers. AI’s accuracy depends on various architectures, while DAEEs’ performance depends on experimental data. This study evaluated the performance of AI and DAEEs for scour depth estimation using flow velocity, depth, size of bed sediment, critical approach velocity, and pier width. The data from a smooth rectangular (20 m × 1 m) flume and a high-precision particle image velocimetry to study the flow structure around the pier - width: 1.5 – 91.5 cm evaluated DAEEs. Various ANNs (5, 10, and 15 neurons), double layer (DL) and triple layers (TL), and different ANFIS settings were trained, tested, and verified. The Generalized Reduced Gradient optimization identified the parameters of DAEEs, and Nash–Sutcliffe efficiency (NSE) and Mean Square Error (MSE) evaluated the performance of different models. The study revealed that DL ANN-3 with 10 neurons (NSE = 0.986) outperformed ANFIS, other ANN (ANN1, ANN2, ANN4 ANN5) models, and empirical equations with NSE values between 0.76 and 0.983. The study found pier dimensions to be the most influential parameter for pier scour.
更多查看译文
关键词
Bridge pier,Scour depth,Artificial intelligence,Artificial neural network,Reduced gradient
AI 理解论文
溯源树
样例
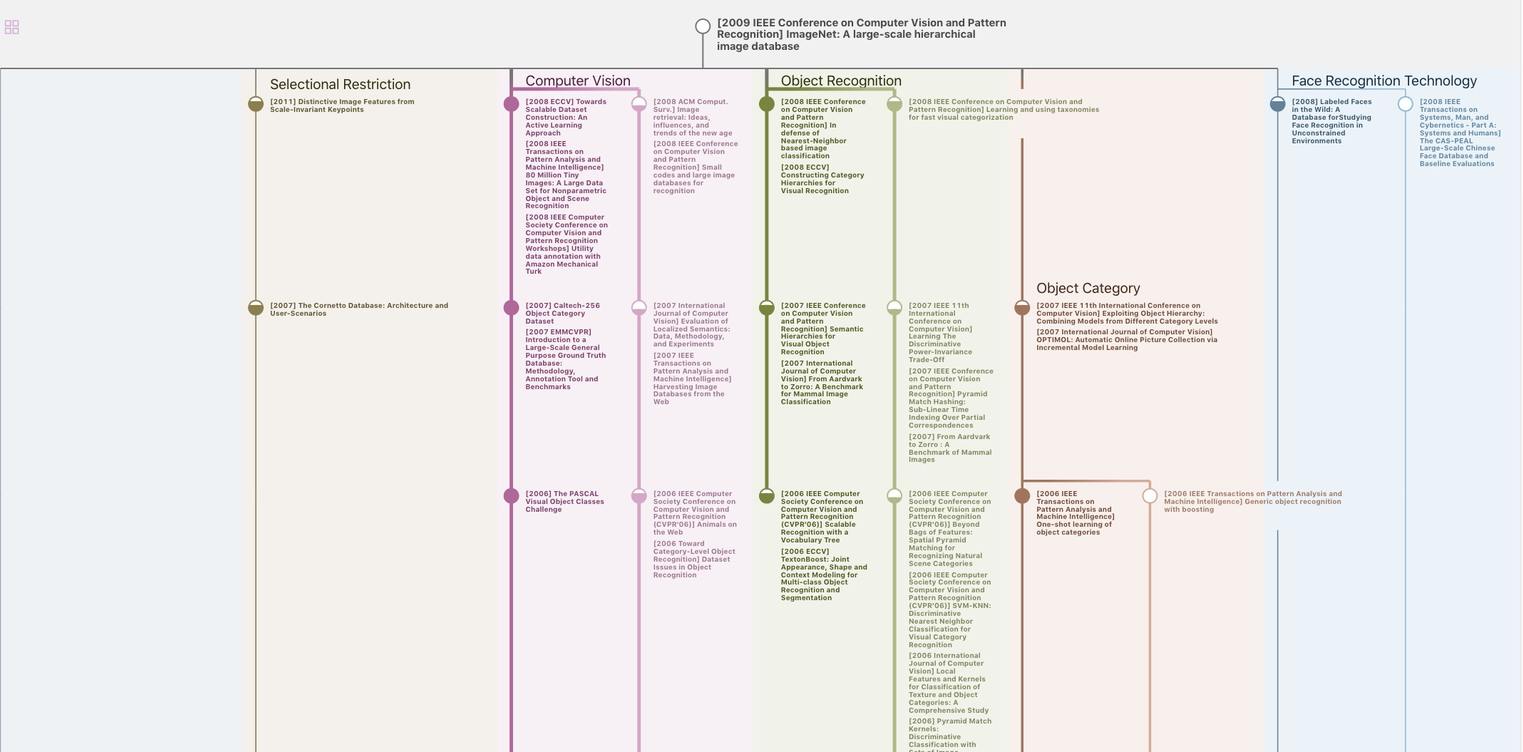
生成溯源树,研究论文发展脉络
Chat Paper
正在生成论文摘要