Unveiling built environment impacts on traffic CO2 emissions using Geo-CNN weighted regression
Transportation Research Part D: Transport and Environment(2024)
摘要
Understanding the associations between the built environment and road traffic CO2 emissions is crucial for developing strategies to mitigate carbon emissions. However, previous research struggled to capture complex spatial relationships accurately due to classical geospatial models’ limitations and the challenges of estimating CO2 emissions from operational vehicle data or limited sample sizes. Therefore, we introduce a novel model that leverages extensive vehicle trajectory data for estimating road traffic CO2 emissions. Furthermore, we develop a geographically convolutional neural network weighted regression (GCNNWR) model to analyze the correlation between the built environment and these emissions. This model employs convolutional neural networks to effectively capture non-linear spatial relationships. An empirical analysis was conducted in Beijing, China, demonstrating the superiority of the GCNNWR model in accommodating spatial heterogeneity compared to conventional geospatial models. Our findings provide critical insights into optimizing the built environment to minimize CO2 emissions.
更多查看译文
关键词
Road traffic CO2 emissions,Spatial–temporal characteristics,Built environment,Geographically weighted regression model,Convolutional neural network
AI 理解论文
溯源树
样例
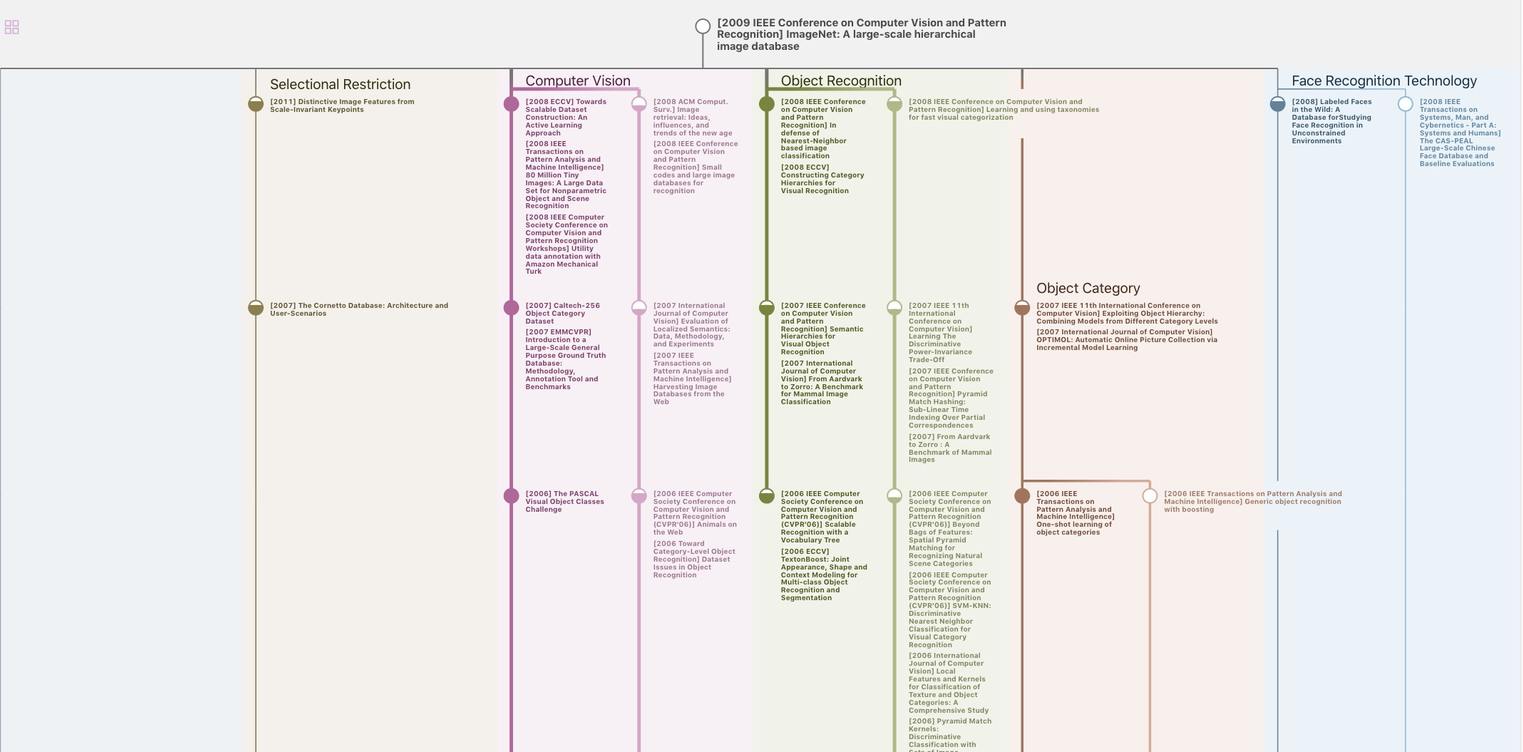
生成溯源树,研究论文发展脉络
Chat Paper
正在生成论文摘要